Using Linked Open Data in Recommender Systems
WIMS(2015)
摘要
In this paper, we present our work in progress on using LOD data to enhance recommending on existing e-commerce sites. We imagine a situation of e-commerce website employing content-based or hybrid recommendation. Such recommending algorithms need relevant object attributes to produce useful recommendations. However, on some domains, usable attributes may be difficult to fill in manually and yet accessible from LOD cloud. A pilot study was conducted on the domain of secondhand bookshops. In this domain, recommending is extraordinary difficult because of high ratio between objects and users, lack of significant attributes and limited availability of items. Both collaborative filtering and content-based recommendation applicability is questionable under this conditions. We queried both Czech and English language edition of DBPedia in order to receive additional information about objects (books) and used various recommending algorithms to learn user preferences. Our approach is general and can be applied on other domains as well. Proposed methods were tested in an off-line recommending scenario with promising results; however there are a lot of challenges for the future work including more complex algorithm analysis, improving SPARQL queries or improving DBPedia matching rules and resource identification.
更多查看译文
AI 理解论文
溯源树
样例
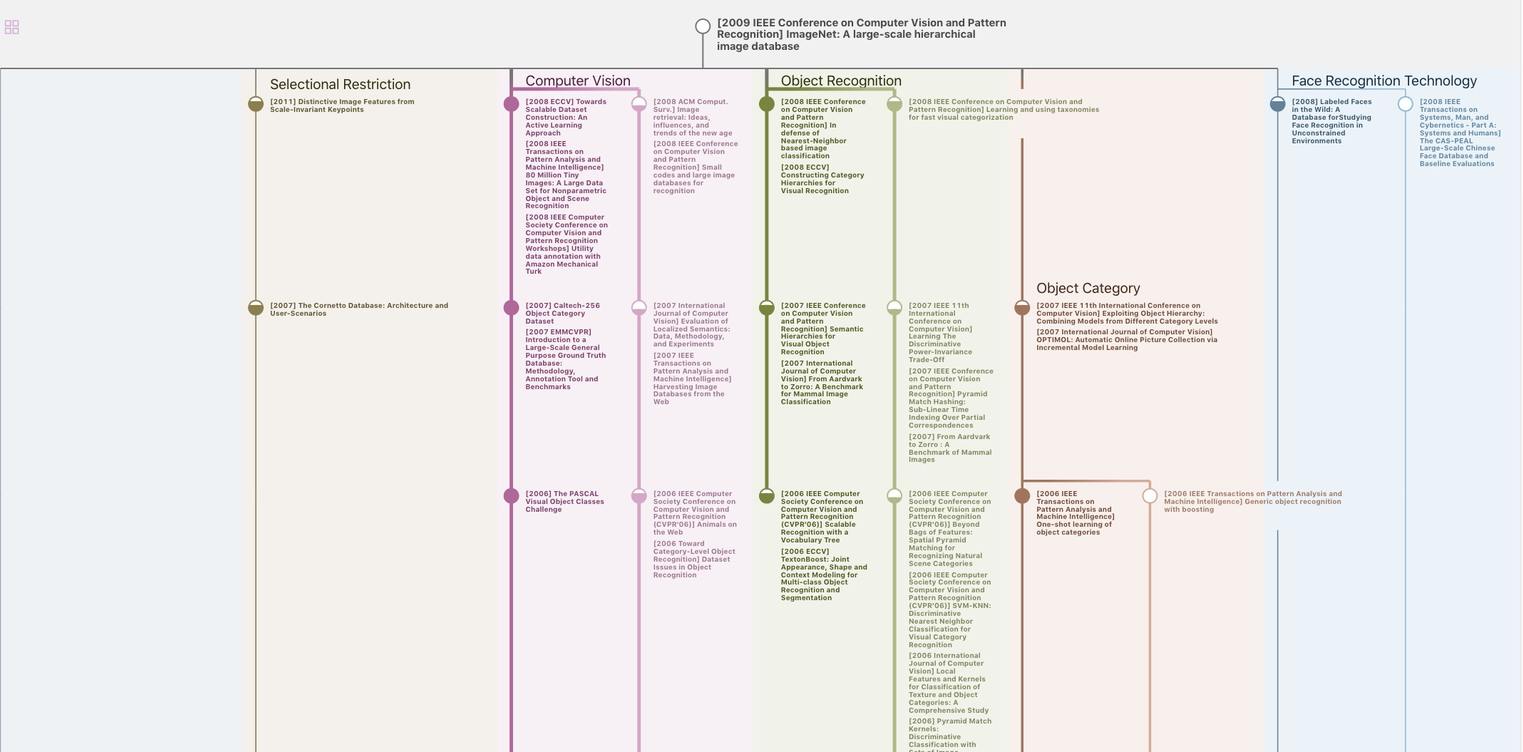
生成溯源树,研究论文发展脉络
Chat Paper
正在生成论文摘要