Improving the Weighted Distribution Estimation for AdaBoost Using a Novel Concurrent Approach.
Studies in Computational Intelligence(2016)
摘要
AdaBoost is one of the most known Ensemble approaches used in the Machine Learning literature. Several AdaBoost approaches that use Parallel processing, in order to speed up the computation in Large datasets, have been recently proposed. These approaches try to approximate the classic AdaBoost, thus sacrificing its generalization ability. In this work, we use Concurrent Computing in order to improve the Distribution Weight estimation, hence obtaining improvements in the capacity of generalization. We train in parallel in each round several weak hypotheses, and using a weighted ensemble we update the distribution weights of the following boosting rounds. Our results show that in most cases the performance of AdaBoost is improved and that the algorithm converges rapidly. We validate our proposal with 4 well-known real data sets.
更多查看译文
关键词
Concurrent Approach, Well-known Real Data Sets, AdaBoost Classifier, Weak Learners, Accelerated Gradient Method
AI 理解论文
溯源树
样例
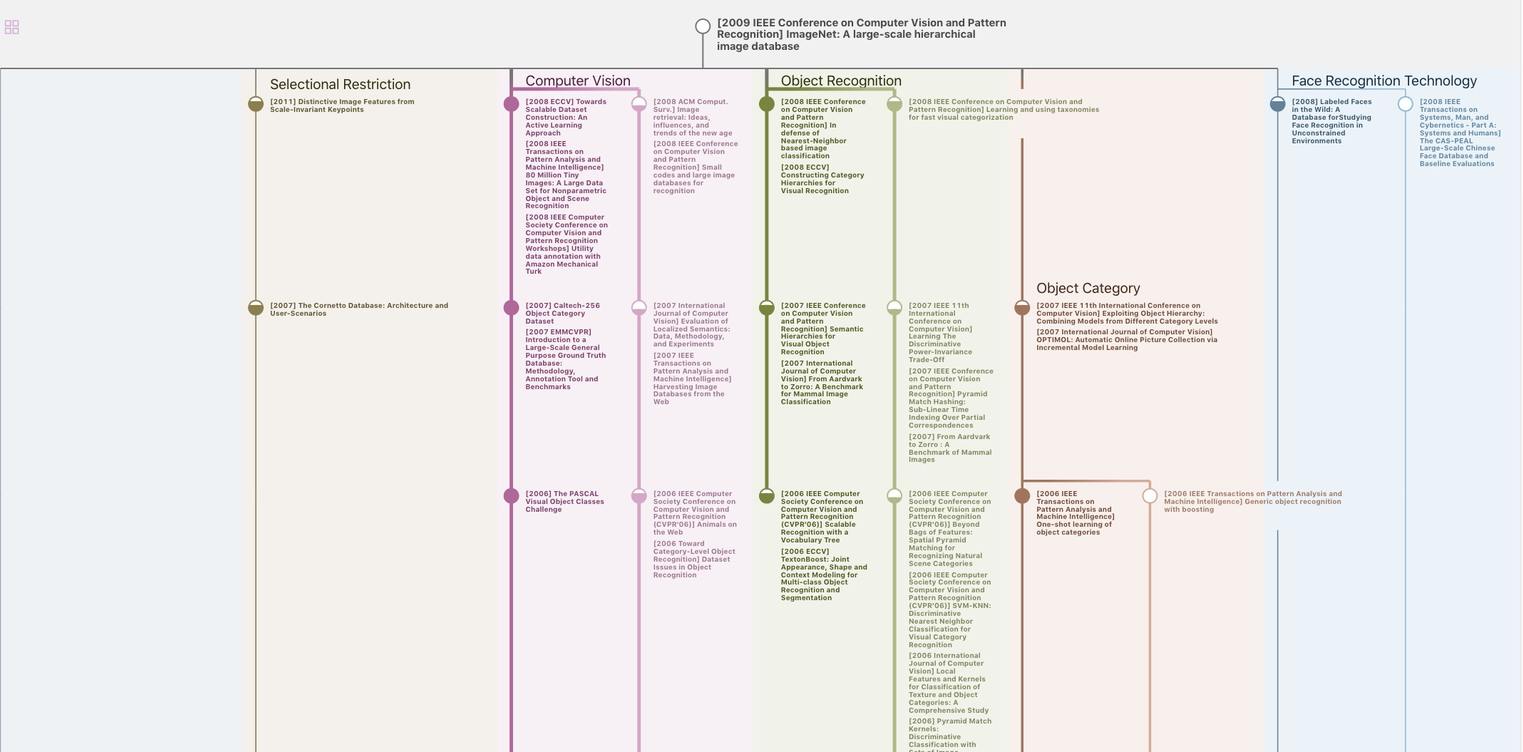
生成溯源树,研究论文发展脉络
Chat Paper
正在生成论文摘要