Augmenting Strong Supervision Using Web Data For Fine-Grained Categorization
2015 IEEE International Conference on Computer Vision (ICCV)(2015)
摘要
We propose a new method for fine-grained object recognition that employs part-level annotations and deep convolutional neural networks (CNNs) in a unified framework. Although both schemes have been widely used to boost recognition performance, due to the difficulty in acquiring detailed part annotations, strongly supervised fine-grained datasets are usually too small to keep pace with the rapid evolution of CNN architectures. In this paper, we solve this problem by exploiting inexhaustible web data. The proposed method improves classification accuracy in two ways: more discriminative CNN feature representations are generated using a training set augmented by collecting a large number of part patches from weakly supervised web images; and more robust object classifiers are learned using a multi-instance learning algorithm jointly on the strong and weak datasets. Despite its simplicity, the proposed method delivers a remarkable performance improvement on the CUB200-2011 dataset compared to baseline part-based R-CNN methods, and achieves the highest accuracy on this dataset even in the absence of test image annotations.
更多查看译文
关键词
strong supervision augmentation,Web data,fine-grained categorization,fine-grained object recognition,part-level annotations,deep convolutional neural networks,recognition performance,strongly supervised fine-grained datasets,CNN architectures,classification accuracy improvement,discriminative CNN feature representations,weakly supervised Web images,robust object classifiers,multiinstance learning algorithm,performance improvement,CUB200-2011 dataset
AI 理解论文
溯源树
样例
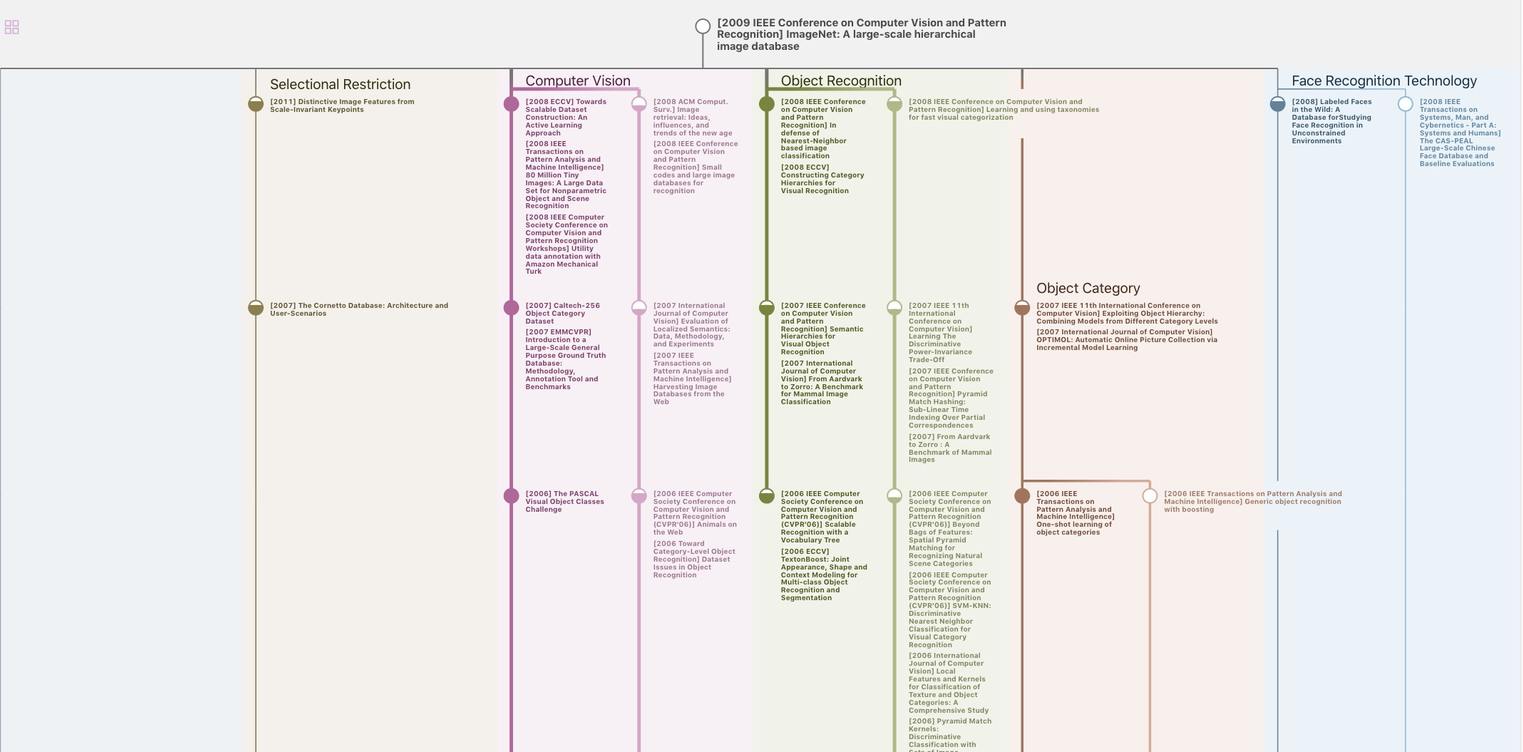
生成溯源树,研究论文发展脉络
Chat Paper
正在生成论文摘要