Unsupervised Domain Adaptation With Imbalanced Cross-Domain Data
2015 IEEE International Conference on Computer Vision (ICCV)(2015)
摘要
We address a challenging unsupervised domain adaptation problem with imbalanced cross-domain data. For standard unsupervised domain adaptation, one typically obtains labeled data in the source domain and only observes unlabeled data in the target domain. However, most existing works do not consider the scenarios in which either the label numbers across domains are different, or the data in the source and/or target domains might be collected from multiple datasets. To address the aforementioned settings of imbalanced cross-domain data, we propose Closest Common Space Learning (CCSL) for associating such data with the capability of preserving label and structural information within and across domains. Experiments on multiple cross-domain visual classification tasks confirm that our method performs favorably against state-of-the-art approaches, especially when imbalanced cross-domain data are presented.
更多查看译文
关键词
unsupervised domain adaptation problem,imbalanced cross-domain data,closest common space learning,CCSL,multiple cross-domain visual classification tasks,preserving label,structural information
AI 理解论文
溯源树
样例
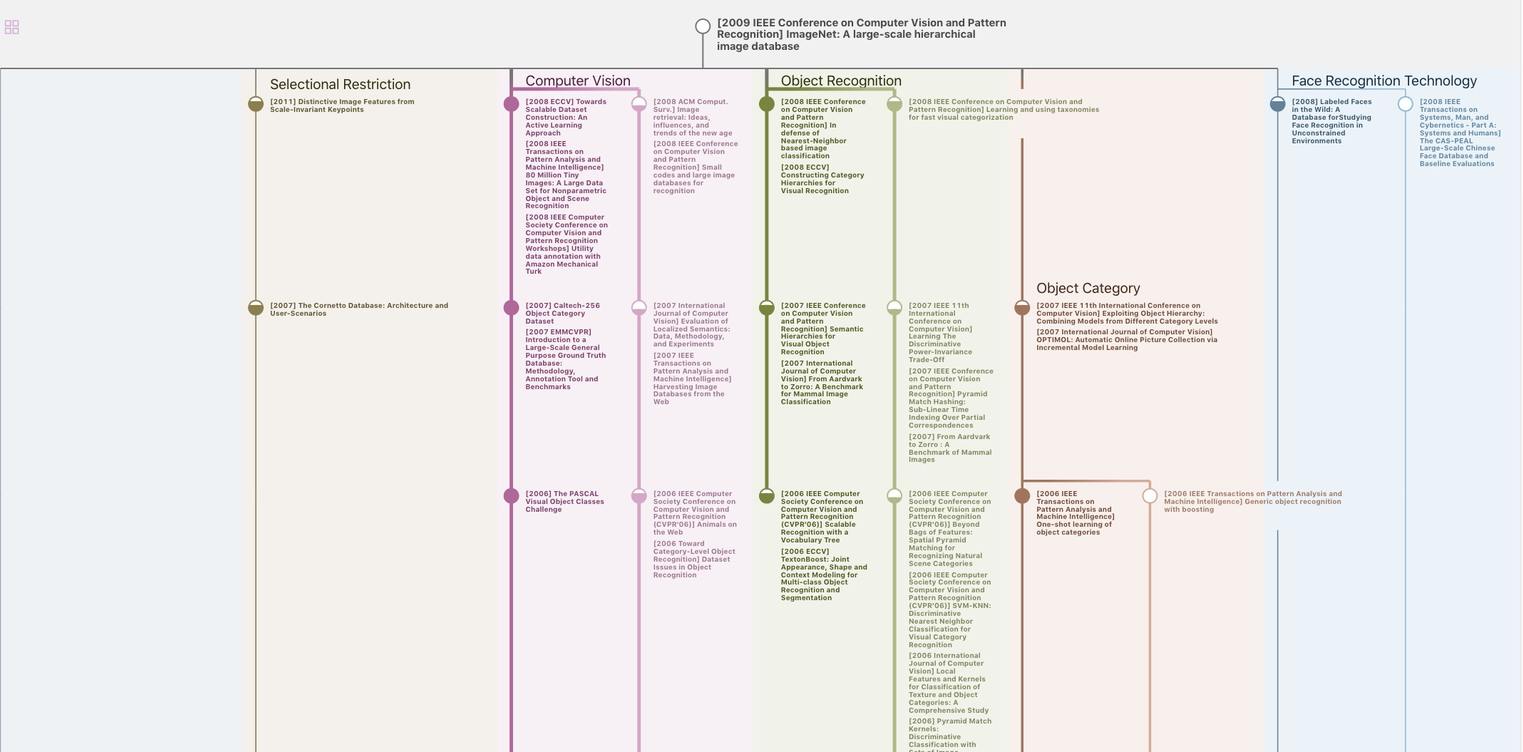
生成溯源树,研究论文发展脉络
Chat Paper
正在生成论文摘要