Global Structure-from-Motion by Similarity Averaging
ICCV(2015)
摘要
Global structure-from-motion (SfM) methods solve all cameras simultaneously from all available relative motions. It has better potential in both reconstruction accuracy and computation efficiency than incremental methods. However, global SfM is challenging, mainly because of two reasons. Firstly, translation averaging is difficult, since an essential matrix only tells the direction of relative translation. Secondly, it is also hard to filter out bad essential matrices due to feature matching failures. We propose to compute a sparse depth image at each camera to solve both problems. Depth images help to upgrade an essential matrix to a similarity transformation, which can determine the scale of relative translation. Thus, camera registration is formulated as a well-posed similarity averaging problem. Depth images also make the filtering of essential matrices simple and effective. In this way, translation averaging can be solved robustly in two convex L1 optimization problems, which reach the global optimum rapidly. We demonstrate this method in various examples including sequential data, Internet data, and ambiguous data with repetitive scene structures.
更多查看译文
关键词
global structure-from-motion,similarity averaging,SfM method,reconstruction accuracy,computation efficiency,translation averaging,feature matching failure,sparse depth image,essential matrix,similarity transformation,relative translation scale,camera registration,convex L1 optimization problem
AI 理解论文
溯源树
样例
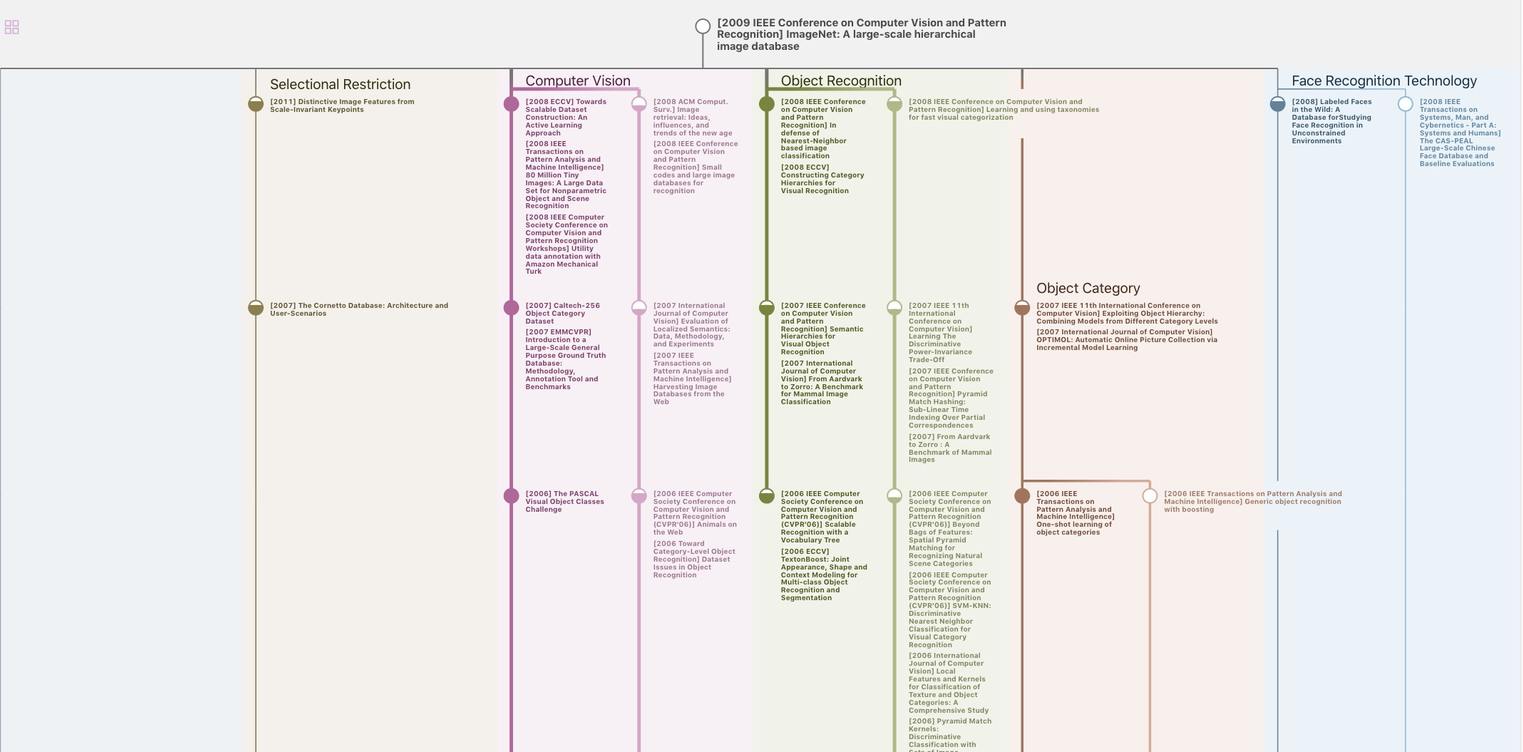
生成溯源树,研究论文发展脉络
Chat Paper
正在生成论文摘要