Relaxed Multiple-Instance SVM with Application to Object Discovery
ICCV(2015)
摘要
Multiple-instance learning (MIL) has served as an important tool for a wide range of vision applications, for instance, image classification, object detection, and visual tracking. In this paper, we propose a novel method to solve the classical MIL problem, named relaxed multiple-instance SVM (RMI-SVM). We treat the positiveness of instance as a continuous variable, use Noisy-OR model to enforce the MIL constraints, and optimize them jointly in a unified framework. The optimization problem can be efficiently solved using stochastic gradient decent. The extensive experiments demonstrate that RMI-SVM consistently achieves superior performance on various benchmarks for MIL. Moreover, we simply applied RMI-SVM to a challenging vision task, common object discovery. The state-of-the arts results of object discovery on PASCAL VOC datasets further confirm the advantages of the proposed method.
更多查看译文
关键词
relaxed multiple-instance SVM,multiple-instance learning,MIL,vision applications,RMI-SVM,noisy-OR model,optimization problem,stochastic gradient decent,common object discovery,PASCAL VOC datasets
AI 理解论文
溯源树
样例
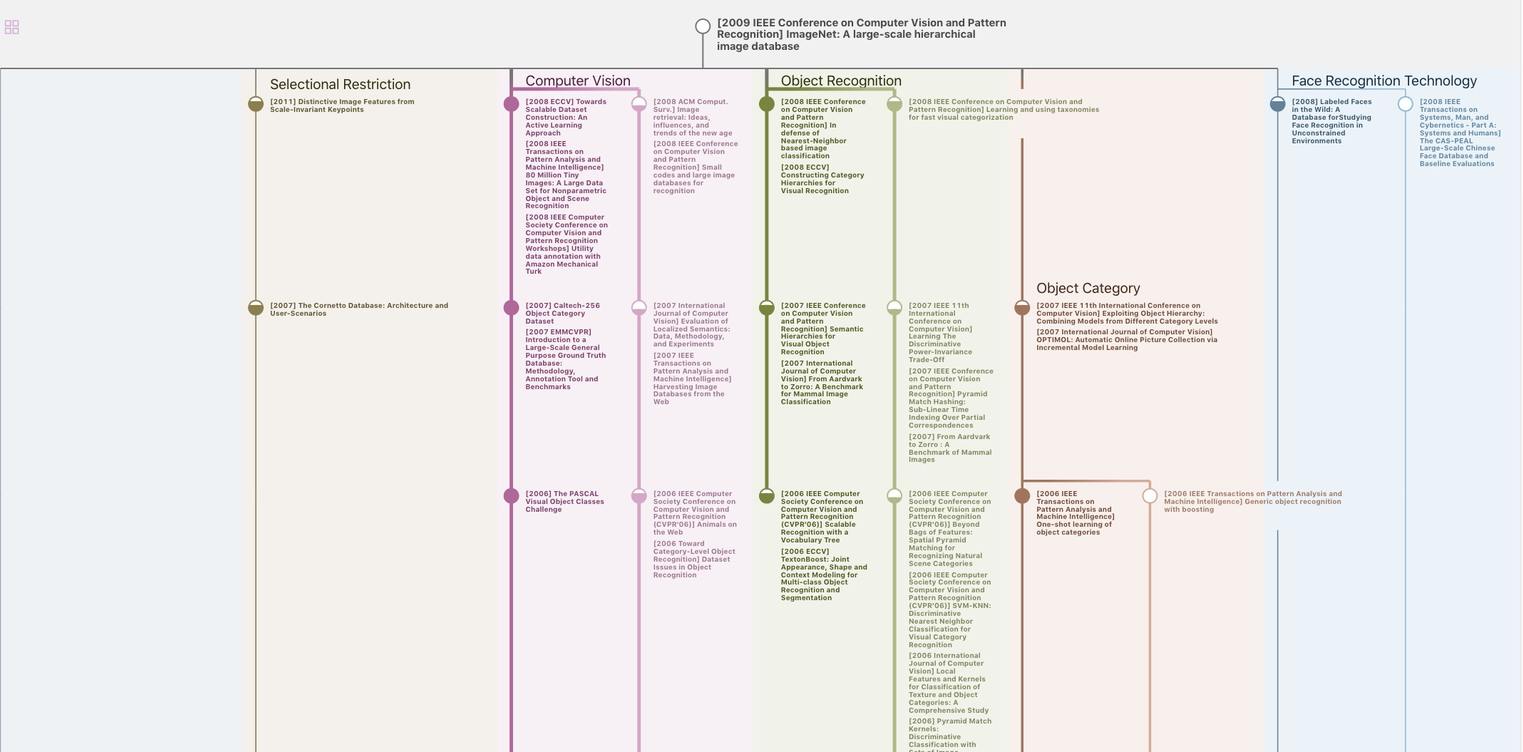
生成溯源树,研究论文发展脉络
Chat Paper
正在生成论文摘要