Improving Image Classification with Location Context
2015 IEEE International Conference on Computer Vision (ICCV)(2015)
摘要
With the widespread availability of cellphones and cameras that have GPS capabilities, it is common for images being uploaded to the Internet today to have GPS coordinates associated with them. In addition to research that tries to predict GPS coordinates from visual features, this also opens up the door to problems that are conditioned on the availability of GPS coordinates. In this work, we tackle the problem of performing image classification with location context, in which we are given the GPS coordinates for images in both the train and test phases. We explore different ways of encoding and extracting features from the GPS coordinates, and show how to naturally incorporate these features into a Convolutional Neural Network (CNN), the current state-of-the-art for most image classification and recognition problems. We also show how it is possible to simultaneously learn the optimal pooling radii for a subset of our features within the CNN framework. To evaluate our model and to help promote research in this area, we identify a set of location-sensitive concepts and annotate a subset of the Yahoo Flickr Creative Commons 100M dataset that has GPS coordinates with these concepts, which we make publicly available. By leveraging location context, we are able to achieve almost a 7% gain in mean average precision.
更多查看译文
关键词
Yahoo Flickr Creative Commons 100M dataset,location-sensitive concepts,CNN framework,optimal pooling radii,image recognition problems,convolutional neural network,feature encoding,feature extraction,GPS coordinates,visual features,Internet,GPS capabilities,cameras,cellphones,location context,image classification
AI 理解论文
溯源树
样例
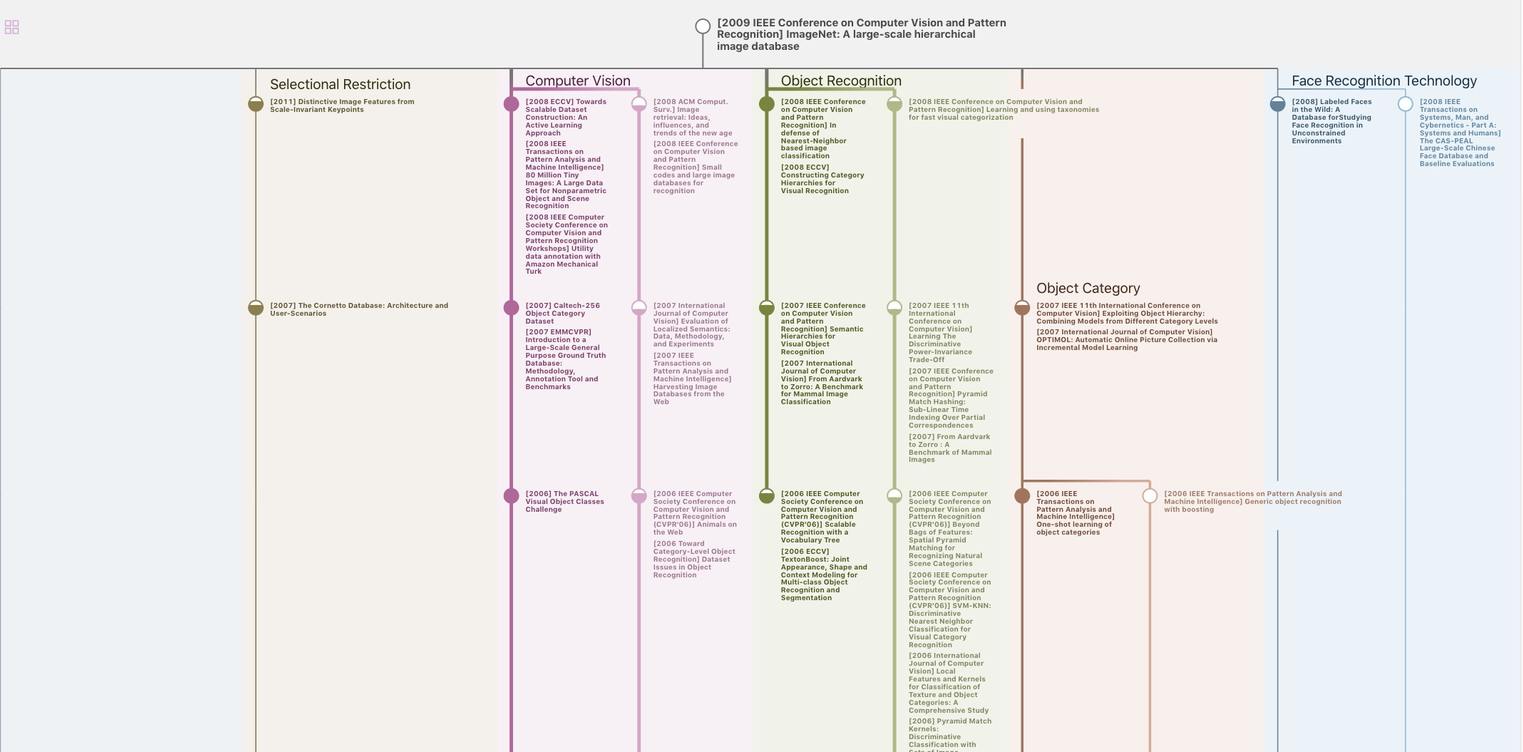
生成溯源树,研究论文发展脉络
Chat Paper
正在生成论文摘要