Objects2action: Classifying and Localizing Actions without Any Video Example
ICCV(2015)
摘要
The goal of this paper is to recognize actions in video without the need for examples. Different from traditional zero-shot approaches we do not demand the design and specification of attribute classifiers and class-to-attribute mappings to allow for transfer from seen classes to unseen classes. Our key contribution is objects2action, a semantic word embedding that is spanned by a skip-gram model of thousands of object categories. Action labels are assigned to an object encoding of unseen video based on a convex combination of action and object affinities. Our semantic embedding has three main characteristics to accommodate for the specifics of actions. First, we propose a mechanism to exploit multiple-word descriptions of actions and objects. Second, we incorporate the automated selection of the most responsive objects per action. And finally, we demonstrate how to extend our zero-shot approach to the spatio-temporal localization of actions in video. Experiments on four action datasets demonstrate the potential of our approach.
更多查看译文
关键词
spatio-temporal localization,automated selection,multiple-word description,semantic embedding,object affinity,convex combination,object encoding,action label,object category,skip-gram model,semantic word embedding,class-to-attribute mapping,attribute classifier,zero-shot approach,action recognition,video example,action localization,action classification,objects2action
AI 理解论文
溯源树
样例
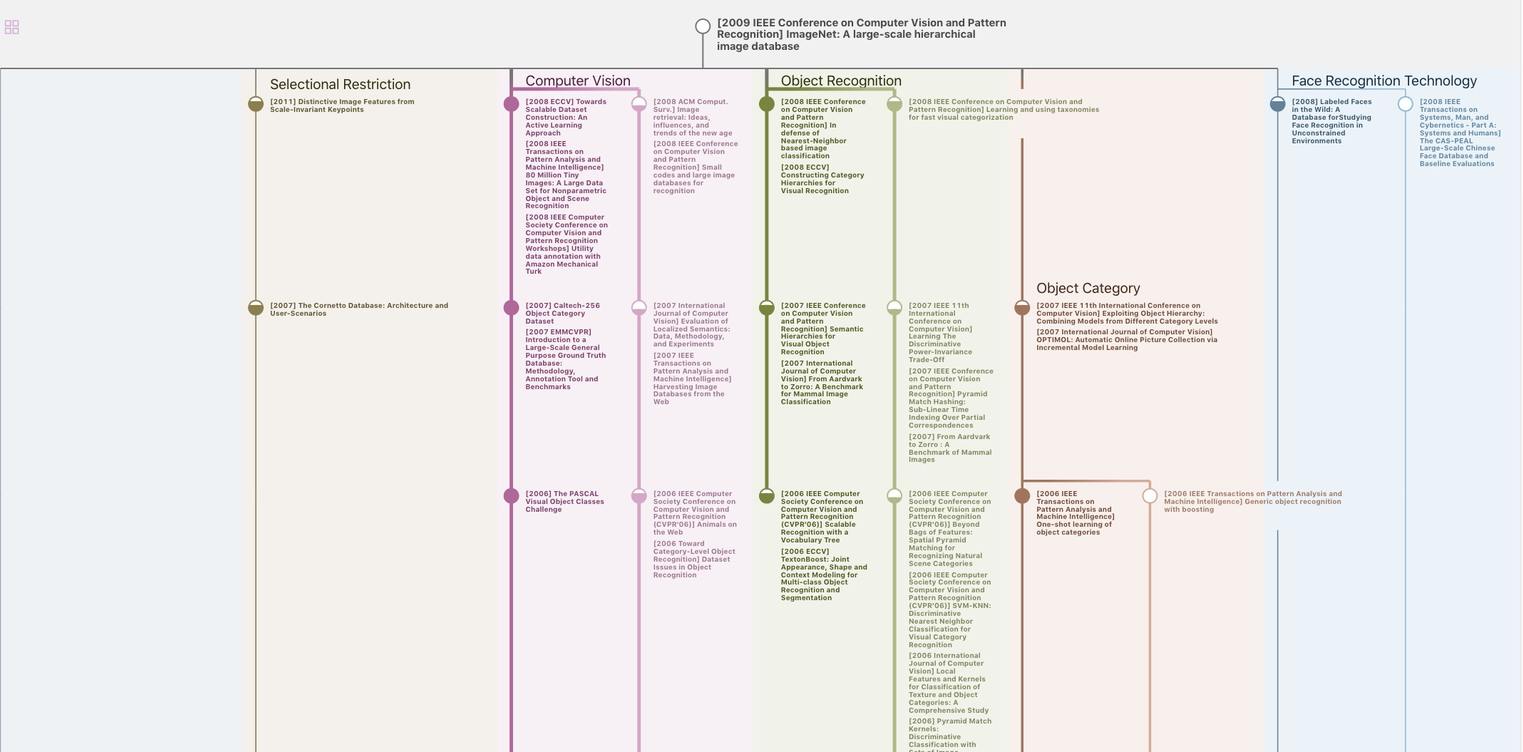
生成溯源树,研究论文发展脉络
Chat Paper
正在生成论文摘要