Hierarchical Discriminative Model For Spoken Language Understanding Based On Convolutional Neural Network
16TH ANNUAL CONFERENCE OF THE INTERNATIONAL SPEECH COMMUNICATION ASSOCIATION (INTERSPEECH 2015), VOLS 1-5(2015)
摘要
This paper presents a novel method for processing automatic speech recognition (ASR) lattices (and generally weighted finite state acceptors) in feed-forward artificial neural networks. It is based on the existing work focused on the text classification using convolutional neural networks (CNNs). The presented method generalizes the convolutional layer of the neural network so that it is able to process both the posterior probabilities and the lexical information contained in an ASR lattice. The convolutional layer was used in a CNN-based implementation of a hierarchical discriminative model (HDM). The method was evaluated using two semantically annotated corpora and the CNN-based HDM improves performance of a spoken language understanding module in comparison with an original HDM based on Support Vector Machines (SVM).
更多查看译文
关键词
spoken language understanding, convolutional neural networks, max-pooling
AI 理解论文
溯源树
样例
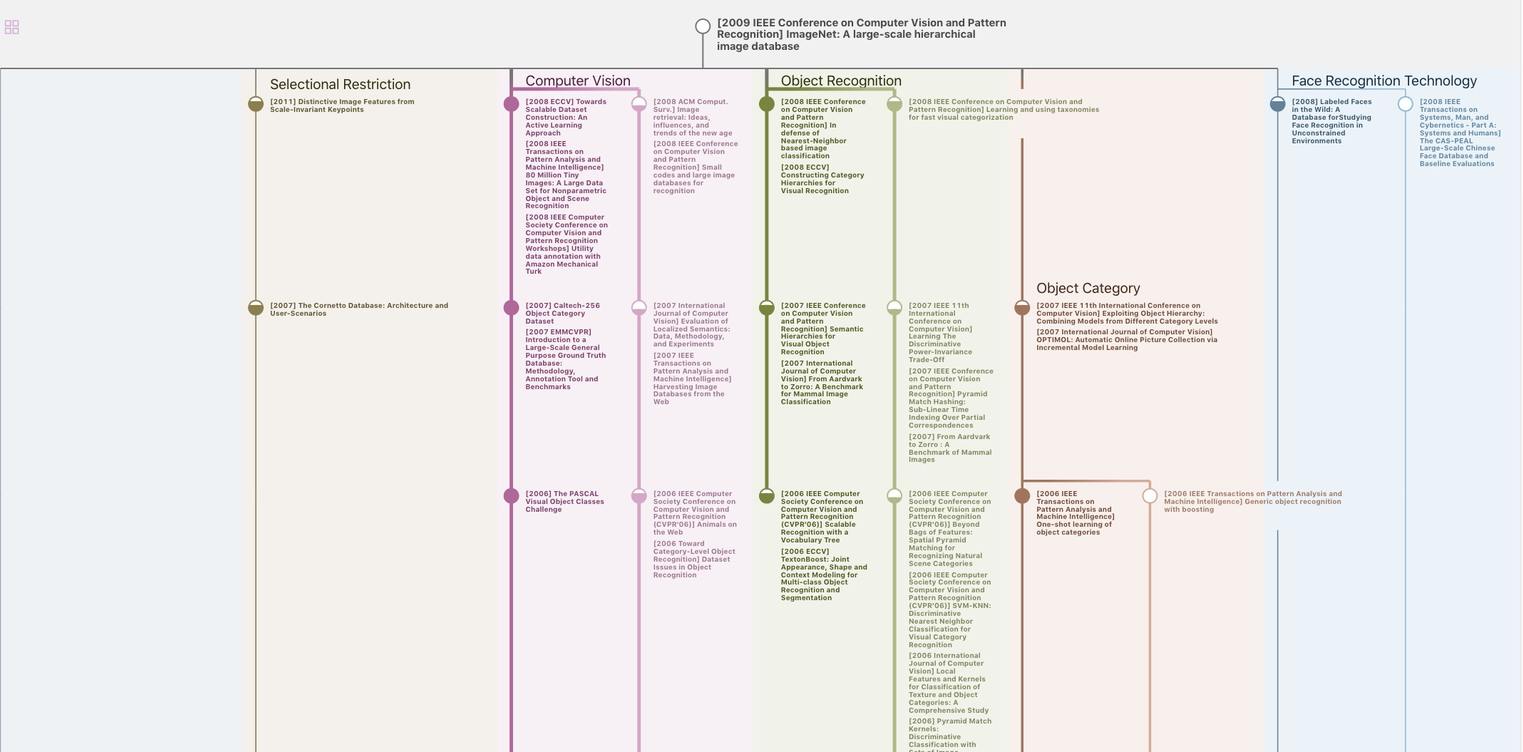
生成溯源树,研究论文发展脉络
Chat Paper
正在生成论文摘要