Large-Scale, Sequence-Discriminative, Joint Adaptive Training For Masking-Based Robust Asr
16TH ANNUAL CONFERENCE OF THE INTERNATIONAL SPEECH COMMUNICATION ASSOCIATION (INTERSPEECH 2015), VOLS 1-5(2015)
摘要
Recently, it was shown that the performance of supervised time frequency masking based robust automatic speech recognition techniques can be improved by training them jointly with the acoustic model [1]. The system in [1], termed deep neural network based joint adaptive training, used fully-connected feed forward deep neural networks for estimating time-frequency masks and for acoustic modeling; stacked log mel spectra was used as features and training minimized cross entropy loss. In this work, we extend such jointly trained systems in several ways. First, we use recurrent neural networks based on long short-term memory (LSTM) units - this allows the use of un-stacked features, simplifying joint optimization. Next, we use a sequence discriminative training criterion for optimizing parameters. Finally, we conduct experiments on large scale data and show that joint adaptive training can provide gains over a strong baseline. Systematic evaluations on noisy voice-search data show relative improvements ranging from 2% at 15 dB to 5.4% at -5 dB over a sequence discriminative, multi-condition trained LSTM acoustic model.
更多查看译文
关键词
automatic speech recognition, noise robustness, joint adaptive training, deep neural network, LSTM
AI 理解论文
溯源树
样例
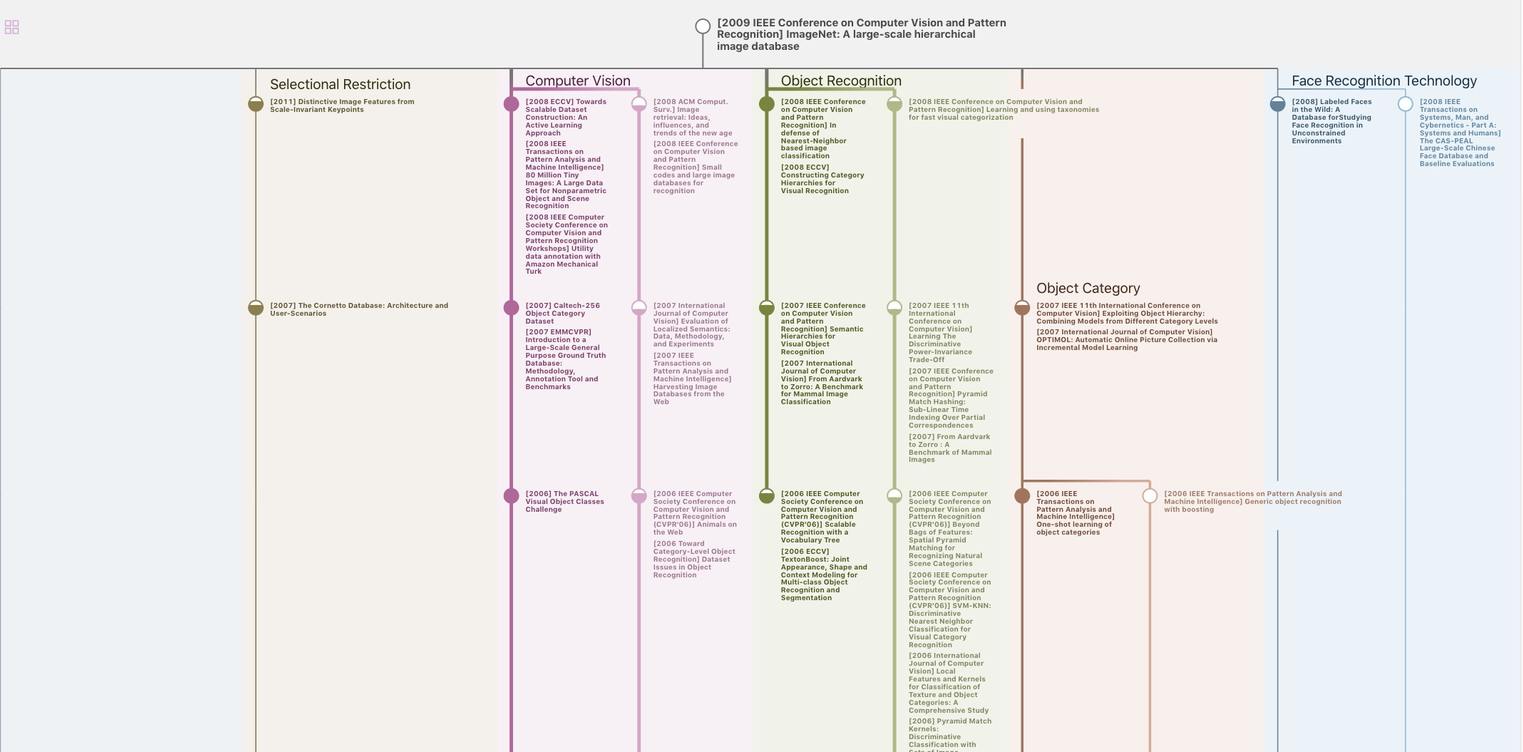
生成溯源树,研究论文发展脉络
Chat Paper
正在生成论文摘要