Learning Bayesian Networks with Non-Decomposable Scores.
GKR(2015)
摘要
Modern approaches for optimally learning Bayesian network structures require decomposable scores. Such approaches include those based on dynamic programming and heuristic search methods. These approaches operate in a search space called the order graph, which has been investigated extensively in recent years. In this paper, we break from this tradition, and show that one can effectively learn structures using non-decomposable scores by exploring a more complex search space that leverages state-of-the-art learning systems based on order graphs. We show how the new search space can be used to learn with priors that are not structure-modular (a particular class of non-decomposable scores). We also show that it can be used to efficiently enumerate the (k)-best structures, in time that can be up to three orders of magnitude faster, compared to existing approaches.
更多查看译文
关键词
Bayesian Network, Goal Node, Integer Linear Programming, Linear Extension, Priority Queue
AI 理解论文
溯源树
样例
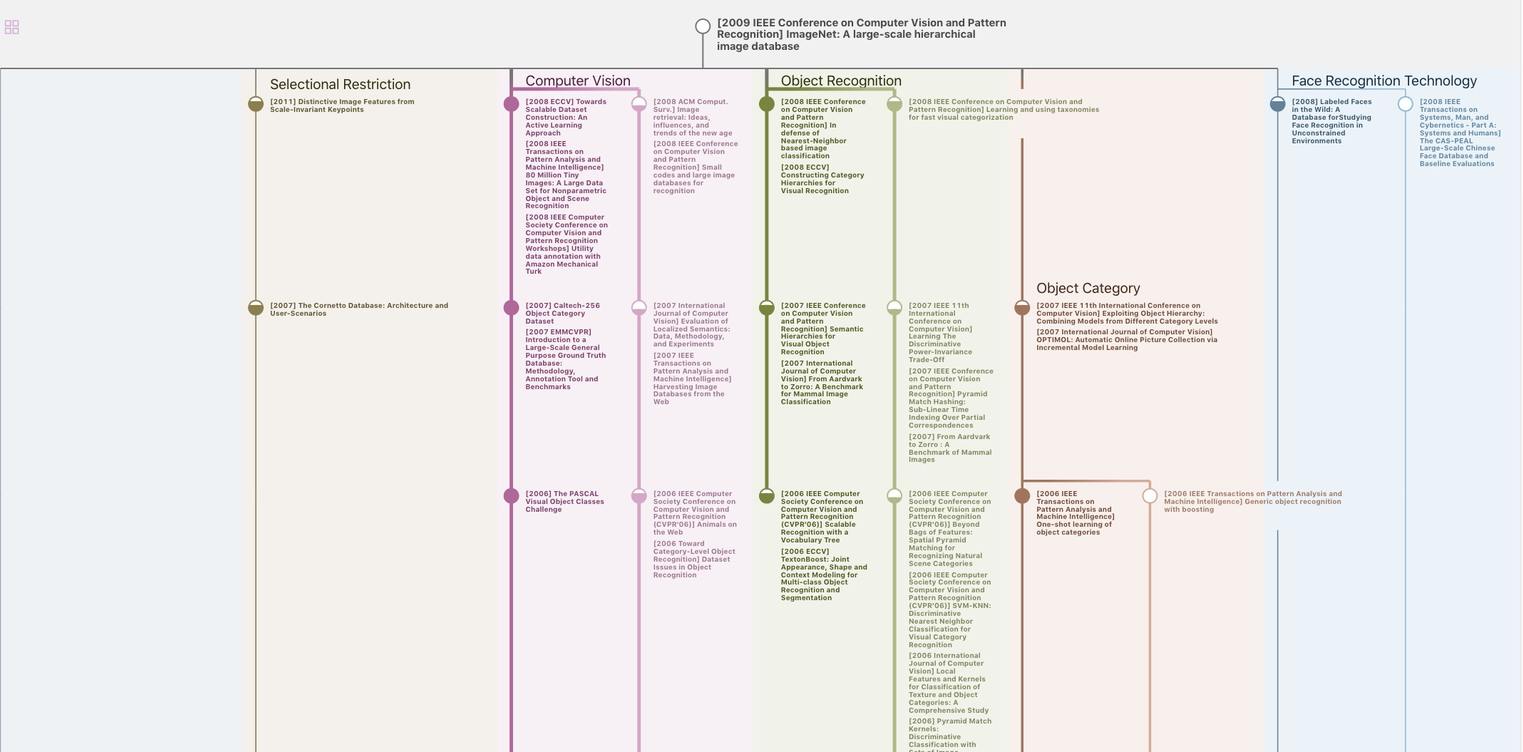
生成溯源树,研究论文发展脉络
Chat Paper
正在生成论文摘要