Exos: Expansion On Session For Enhancing Effectiveness Of Query Auto-Completion
2015 IEEE International Conference on Big Data (Big Data)(2015)
摘要
Query auto-completion (QAC) is an important feature of a search system that helps users reach their target queries faster. Personalization is a powerful technique that improves the relevance of QAC to the current query. This paper proposes a novel framework, EXOS, to augment the native query by important tokens in the user's search contexts to provide personalized auto-completions dynamically. EXOS is composed of three major steps: expansion, selection and boosting. Each token from the user's search contexts is evaluated and stored by EXOS. The tokens with the highest importance are chosen by the expansion step to augment the native query from the user. These augmented queries are used to retrieve QAC results. The selection and boosting models then extract qualified results, merge and re-rank these results based on their query importance to provide the user with a personalized QAC.We designed two large-scale experiments with over six million queries to evaluate the effectiveness of EXOS. Compared with an advanced MostPopularCompletion system, EXOS has 126% gain on Mean Reciprocal Rank (MRR) and 270% gain on Success Rate at Top-1 in a prefix-based experiment. EXOS shows 10.5% gain on first-token MRR and about 9% gain on Token Saved Rate in a token-based experiment. The results of our experiments show that EXOS significantly enhances the relevance of QAC by predicting the user's target query with using fewer keystrokes.
更多查看译文
关键词
query auto-completion,personalization,interactive search,context-awareness
AI 理解论文
溯源树
样例
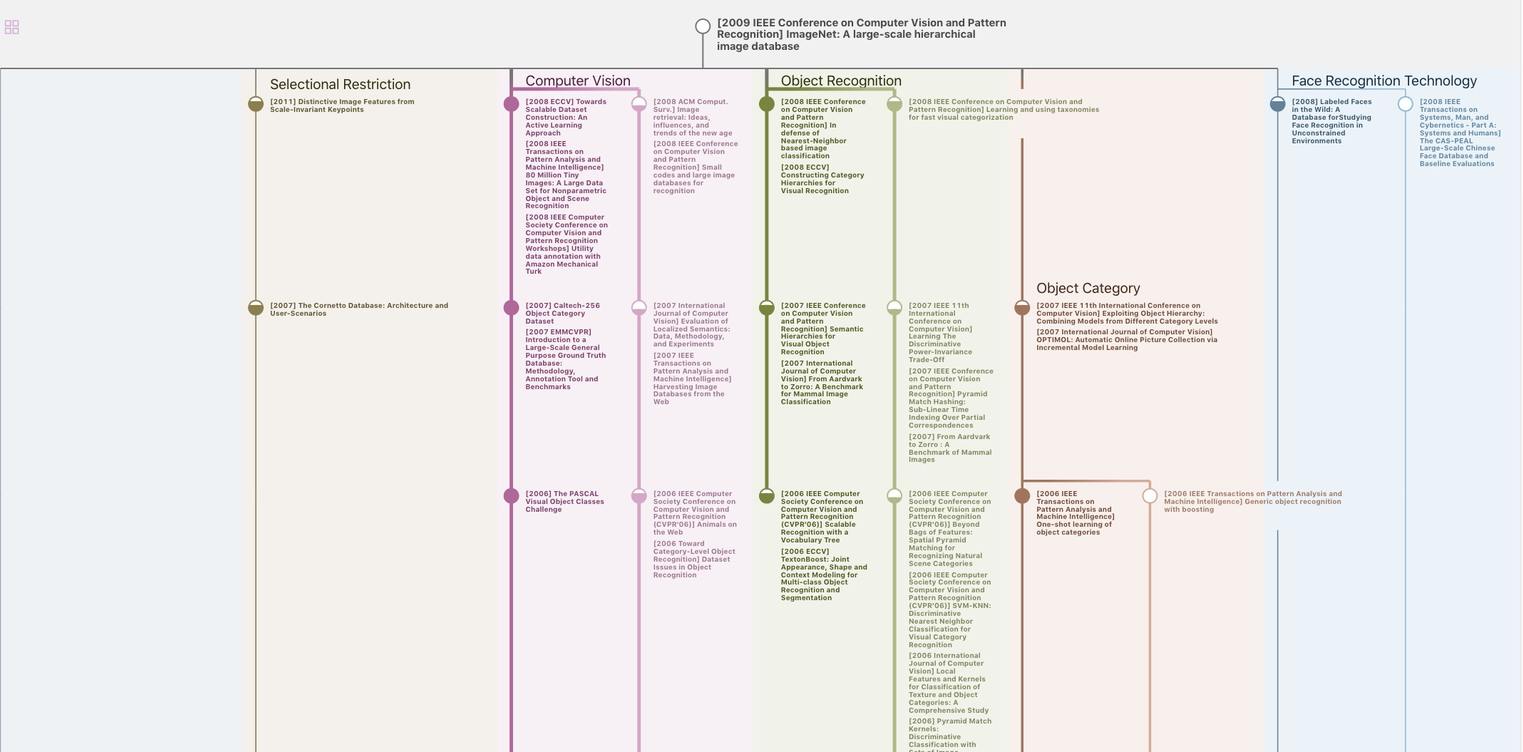
生成溯源树,研究论文发展脉络
Chat Paper
正在生成论文摘要