Selectively Inhibiting Learning Bias for Active Sampling.
BRACIS(2015)
摘要
Efficient training of machine learning algorithms requires a reliable labeled set from the application domain. Usually, data labeling is a costly process. Therefore, a selective approach is desirable. Active learning has been successfully used to reduce the labeling effort, due to its parsimonious process of querying the labeler. Nevertheless, many active learning strategies are dependent on early predictions made by learning algorithms. This might be a major problem when the learner is still unable to provide reliable information. In this context, agnostic strategies can be convenient, since they spare internal learners - usually favoring exploratory queries. On the other hand, prospective queries could benefit from a learning bias. In this article, we highlight the advantages of the agnostic approach and propose how to explore some of them without foregoing prospect ion. A simple hybrid strategy and a visualization tool called ranking curves, are proposed as a proof of concept. The tool allowed to see clearly when the presence of a learner was possibly detrimental. Finally, the hybrid strategy was successfully compared to its counterpart in the literature, to pure agnostic strategies and to the usual baseline of the field.
更多查看译文
关键词
active learning, machine learning, agnostic
AI 理解论文
溯源树
样例
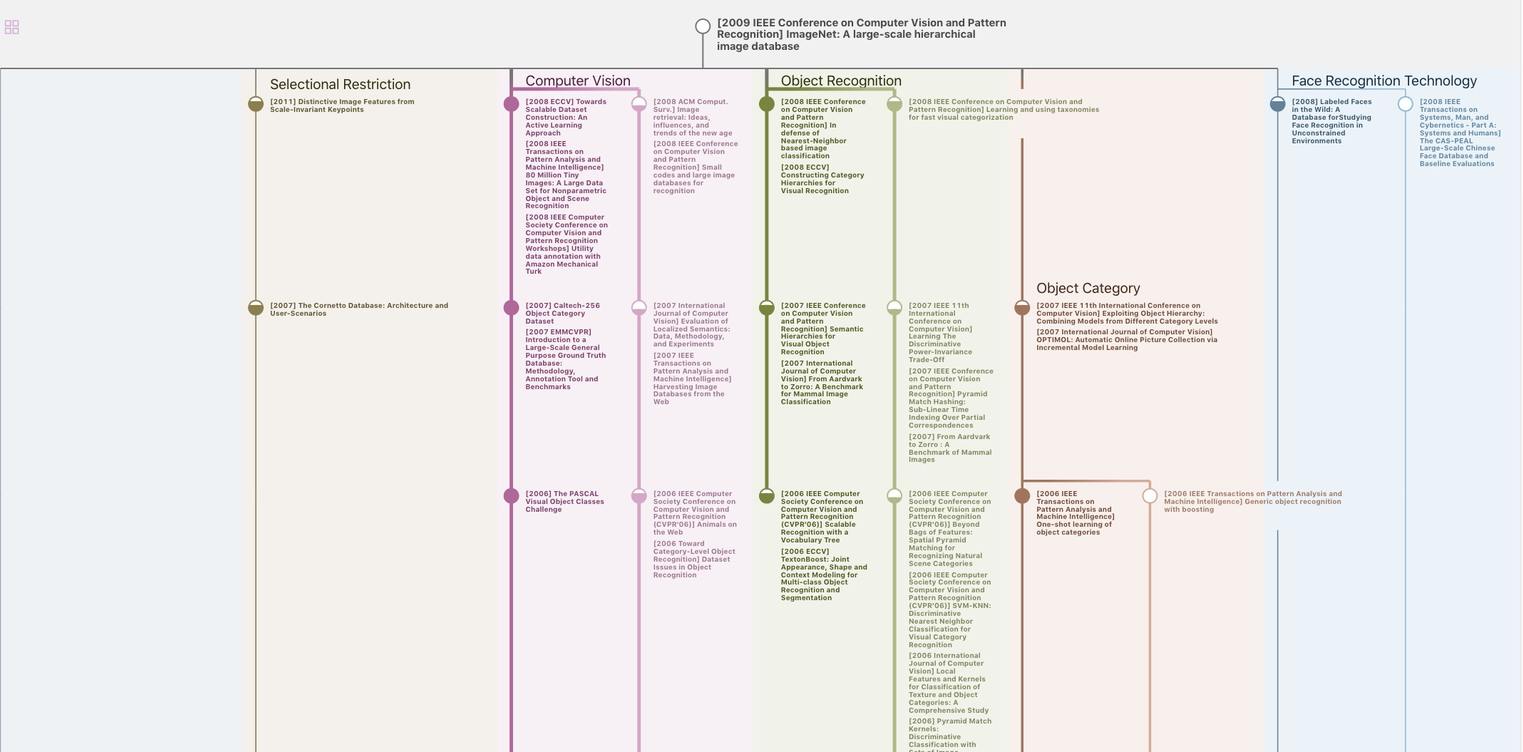
生成溯源树,研究论文发展脉络
Chat Paper
正在生成论文摘要