Sequential UBM adaptation for speaker verification
ChinaSIP(2013)
摘要
GMM-UBM-based speaker verification heavily relies on a well trained UBM. In practice, it is not often easy to obtain an UBM that fully matches acoustic channels in operation. To solve this problem, we propose a novel sequential MAP adaptation approach: by being sequentially updated with data from new enrollments, the UBM learns and converges to the working channel. Our experiments are conducted on a time-varying speech database, with two channel-mismatched UBMs as the initial model. The results confirm that the sequential UBM adaptation provides significant performance improvement, leading to a relative EER reduction of 6.3% and 14.8% for the two mismatched UBMs, respectively. © 2013 IEEE.
更多查看译文
关键词
Gaussian processes,maximum likelihood estimation,speaker recognition,Gaussian mixture model,acoustic channels,channel mismatched UBM,maximum a posterior estimation,sequential MAP adaptation approach,sequential UBM adaptation,speaker verification,time varying speech database,universal background model,MAP,UBM,speaker verification,
AI 理解论文
溯源树
样例
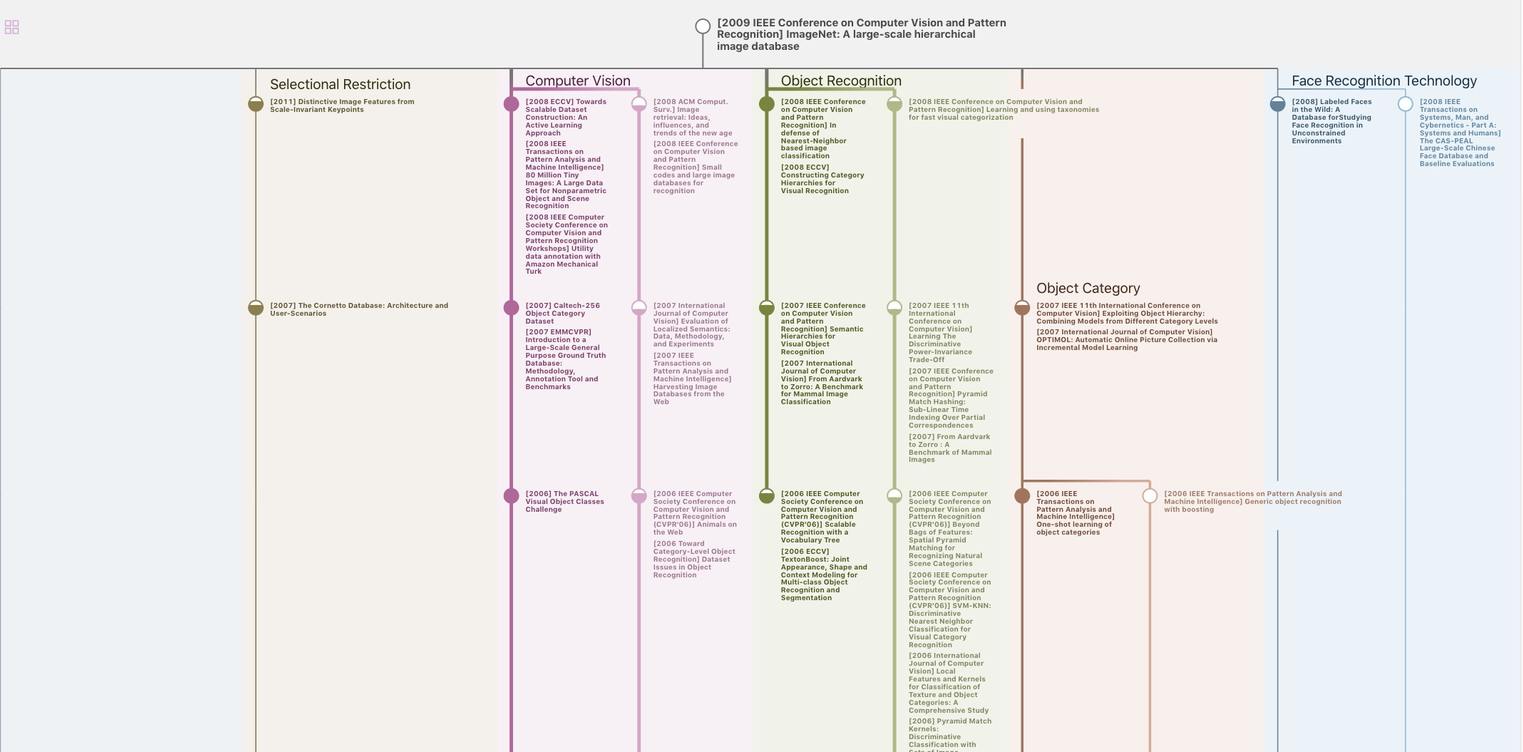
生成溯源树,研究论文发展脉络
Chat Paper
正在生成论文摘要