Improving deep neural networks by using sparse dropout strategy
ChinaSIP(2014)
摘要
Recently, deep neural networks(DNNs) have achieved excellent results on benchmarks for acoustic modeling of speech recognition. By randomly discarding network units, a strategy which is called as dropout can improve the performance of DNNs by reducing the influence of over-fitting. However, the random dropout strategy treats units indiscriminately, which may lose information on distributions of units outputs. In this paper, we improve the dropout strategy by differential treatment to units according to their outputs. Only minor changes to an existing neural network system can achieve a significant improvement. Experiments of phone recognition on TIMIT show that the sparse dropout fine-tuning gets significant performance improvement.
更多查看译文
关键词
dropout, sparse dropout, deep neural networks, deep learning
AI 理解论文
溯源树
样例
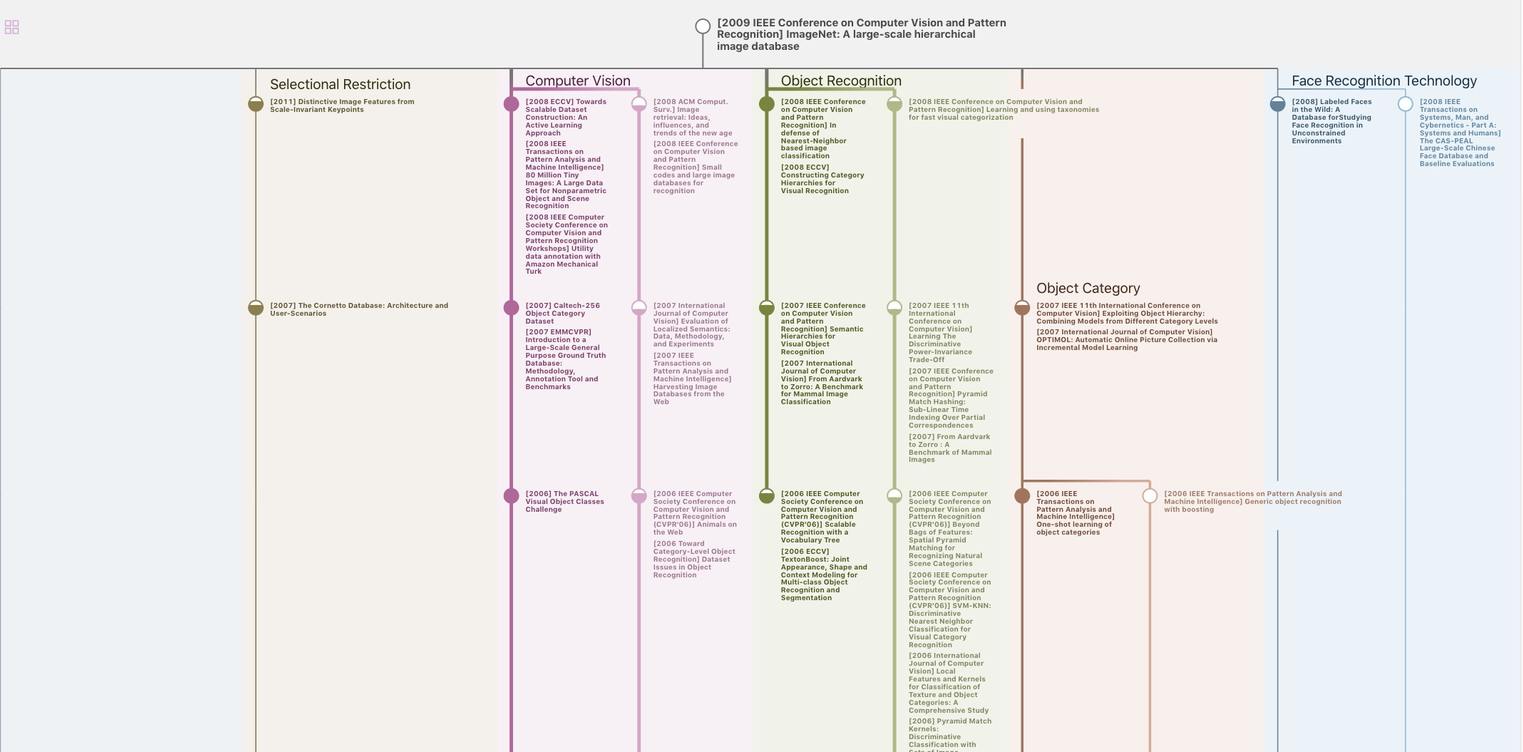
生成溯源树,研究论文发展脉络
Chat Paper
正在生成论文摘要