Surface Classification For Sensor Deployment From Uav Landings
2015 IEEE International Conference on Robotics and Automation (ICRA)(2015)
摘要
Using Unmanned Aerial Vehicles (UAVs) to deploy sensor networks promises an autonomous and useful method of installation in remote or hard to access locations. Some sensors, such as soil moisture sensors, must be physically installed in soft soil, yet UAVs cannot easily determine soil softness with remote sensors. In this paper, we use data from an onboard accelerometer measured during UAV landings to determine the softness of the ground. We collect and analyze over 200 data sets gathered from 8 different materials: foam, carpet, wood, tile, grass, dirt, concrete, and woodchips. Based on this analysis, we examine a number of features from the accelerometer and four classification algorithms: LDA, QDA, SVM, and binary decision trees. The decision tree performs well and is simple to implement onboard the UAV. We implement this in our UAV control system and perform experiments to verify that the UAV can accurately classify the softness of the surface with 90% accuracy. This lays the groundwork for our future work on developing a UAV capable of installing sensors in soft soil.
更多查看译文
关键词
surface classification,sensor deployment,unmanned aerial vehicles,sensor networks,soil moisture sensors,soil softness,onboard accelerometer,UAV landings,accelerometer,classification algorithms,LDA,QDA,SVM,binary decision trees
AI 理解论文
溯源树
样例
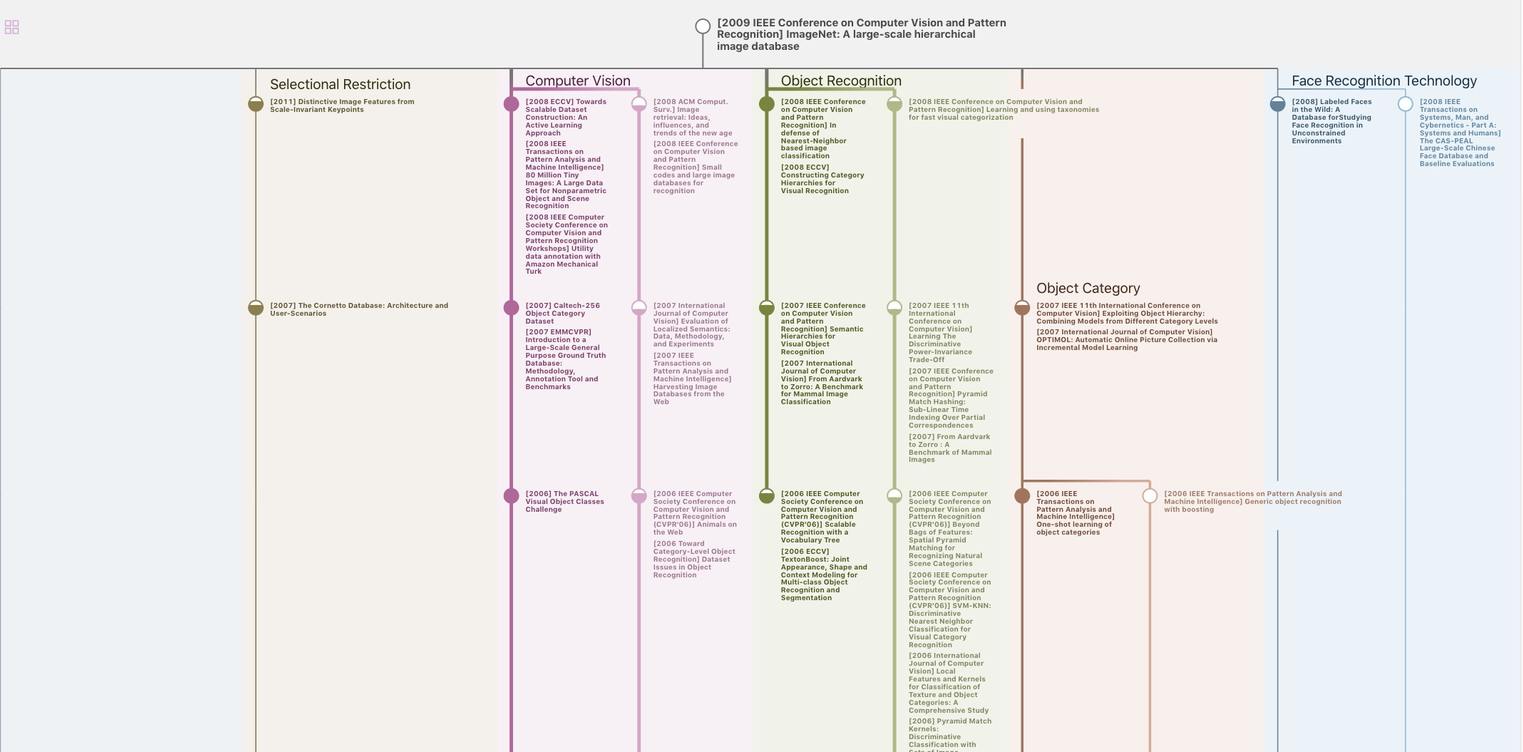
生成溯源树,研究论文发展脉络
Chat Paper
正在生成论文摘要