Iris: Tapping Wearable Sensing To Capture In-Store Retail Insights On Shoppers
2016 IEEE INTERNATIONAL CONFERENCE ON PERVASIVE COMPUTING AND COMMUNICATIONS (PERCOM)(2016)
摘要
We investigate the possibility of using a combination of a smartphone and a smartwatch, carried by a shopper, to get insights into the shopper's behavior inside a retail store. The proposed IRIS framework uses standard locomotive and gestural micro-activities as building blocks to define novel composite features that help classify different facets of a shopper's interaction/experience with individual items, as well as attributes of the overall shopping episode or the store. Besides defining such novel features, IRIS builds a novel segmentation algorithm, which partitions the duration of an entire shopping episode into atomic item-level interactions, by using a combination of feature-based landmarking, change point detection and variable-order HMM-based sequence prediction. Experiments with 50 real-life grocery shopping episodes, collected from 25 shoppers, we show that IRIS can demarcate item-level interactions with an accuracy of approx. 91%, and subsequently characterize item-and-episode level shopper behavior with accuracies of over 90%.
更多查看译文
关键词
variable-order HMM-based sequence prediction,change point detection,feature-based landmarking combination,atomic item-level interactions,retail store,smartwatch,smartphone,IRIS
AI 理解论文
溯源树
样例
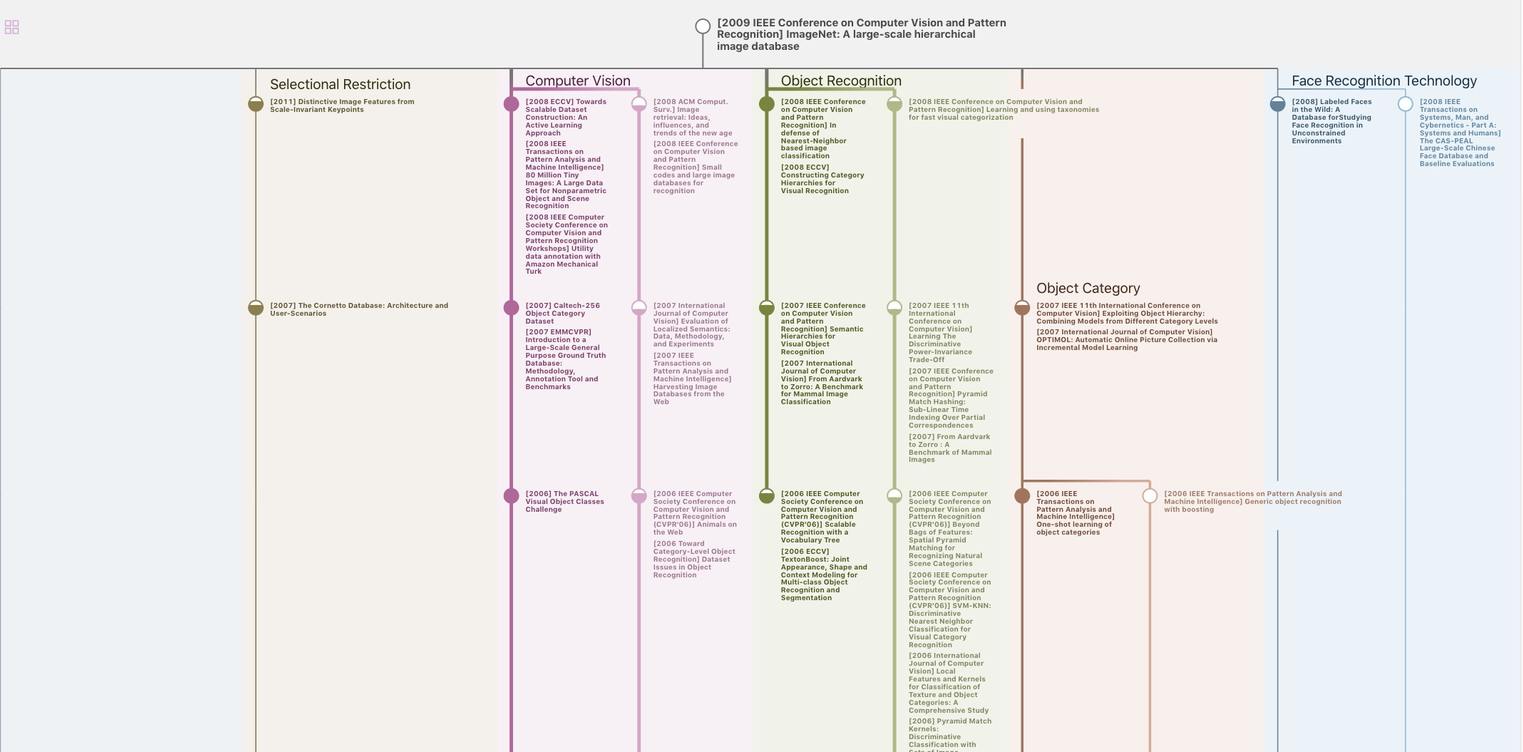
生成溯源树,研究论文发展脉络
Chat Paper
正在生成论文摘要