Correlation in Hard Distributions in Communication Complexity
international workshop and international workshop on approximation randomization and combinatorial optimization algorithms and techniques(2015)
摘要
We study the effect that the amount of correlation in a bipartite distribution has on the communication complexity of a problem under that distribution. We introduce a new family of complexity measures that interpolates between the two previously studied extreme cases: the (standard) randomised communication complexity and the case of distributional complexity under product distributions. We give a tight characterisation of the randomised complexity of Disjointness under distributions with mutual information $k$, showing that it is $\Theta(\sqrt{n(k+1)})$ for all $0\leq k\leq n$. This smoothly interpolates between the lower bounds of Babai, Frankl and Simon for the product distribution case ($k=0$), and the bound of Razborov for the randomised case. The upper bounds improve and generalise what was known for product distributions, and imply that any tight bound for Disjointness needs $\Omega(n)$ bits of mutual information in the corresponding distribution. We study the same question in the distributional quantum setting, and show a lower bound of $\Omega((n(k+1))^{1/4})$, and an upper bound, matching up to a logarithmic factor. We show that there are total Boolean functions $f_d$ on $2n$ inputs that have distributional communication complexity $O(\log n)$ under all distributions of information up to $o(n)$, while the (interactive) distributional complexity maximised over all distributions is $\Theta(\log d)$ for $6n\leq d\leq 2^{n/100}$. We show that in the setting of one-way communication under product distributions, the dependence of communication cost on the allowed error $\epsilon$ is multiplicative in $\log(1/\epsilon)$ -- the previous upper bounds had the dependence of more than $1/\epsilon$.
更多查看译文
AI 理解论文
溯源树
样例
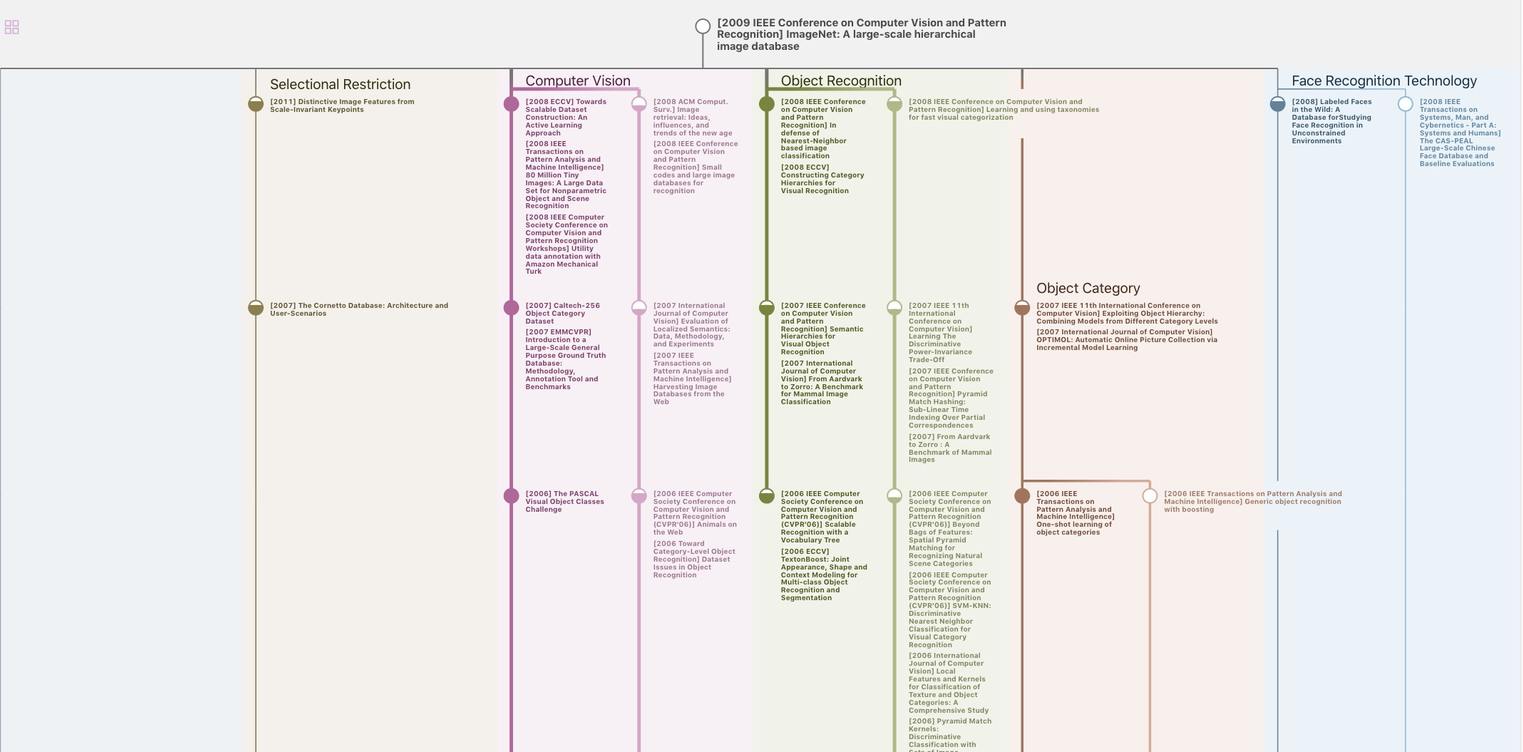
生成溯源树,研究论文发展脉络
Chat Paper
正在生成论文摘要