Enhancing Stock Price Prediction With A Hybrid Approach Based Extreme Learning Machine
2015 IEEE International Conference on Data Mining Workshop (ICDMW)(2015)
摘要
In this paper, we focus on the problem of how to design a methodology which can improve the prediction accuracy as well as speed up prediction process for stock market prediction. As market news and stock prices are commonly believed as two important market data sources, we present the design of our stock price prediction model based on those two data sources concurrently. Firstly, in order to get the most significant features of the market news documents, we propose a new feature selection algorithm (NRDC), as well as a new feature weighting algorithm (N-TF-IDF) to help improve the prediction accuracy. Then we employ a fast learning model named Extreme Learning Machine( ELM) and use the kernel-based ELM (K-ELM) to improve the prediction speed. Comprehensive experimental comparisons between our hybrid proposal K-ELM with NRDC and N-TF-IDF(N-N-K-ELM) and the state-of-the-art learning algorithms, including Support Vector Machine (SVM) and Back-Propagation Neural Network (BP-NN), have been undertaken on the intra-day tick-by-tick data of the H-share market and contemporaneous news archives. Experimental results show that our N-N-K-ELM model can achieve better performance on the consideration of both prediction accuracy and prediction speed in most cases.
更多查看译文
关键词
hybrid approach based extreme learning machine,prediction process,stock market prediction,market data sources,stock price prediction model,market news documents,feature selection algorithm,NRDC,feature weighting algorithm,N-TF-IDF,prediction accuracy,kernel-based ELM,K-ELM,state-of-the-art learning algorithms,support vector machine,SVM,back-propagation neural network,BP-NN,intraday tick-by-tick data,H-share market,contemporaneous news archives,N-N-K-ELM model
AI 理解论文
溯源树
样例
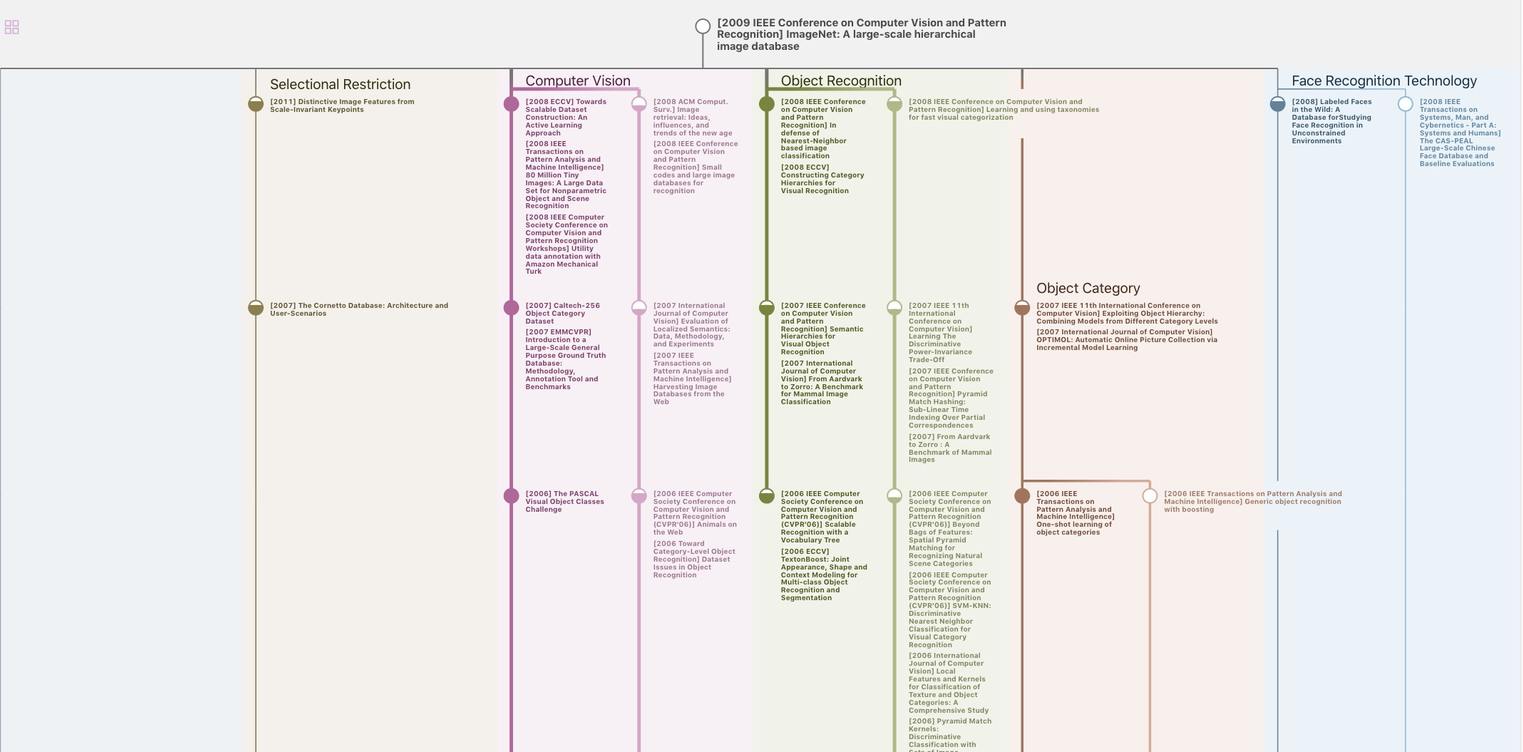
生成溯源树,研究论文发展脉络
Chat Paper
正在生成论文摘要