Detecting Overlapping Communities from Local Spectral Subspaces
IEEE International Conference on DataMining(2015)
摘要
Based on the definition of local spectral subspace, we propose a novel approach called LOSP for local overlapping community detection. Using the power method for a few steps, LOSP finds an approximate invariant subspace, which depicts the embedding of the local neighborhood structure around the seeds of interest. LOSP then identifies the local community expanded from the given seeds by seeking a sparse indicator vector in the subspace where the seeds are in its support. We provide a systematic investigation on LOSP, and thoroughly evaluate it on large real world networks across multiple domains. With the prior information of very few seed members, LOSP can detect the remaining members of a target community with high accuracy. Experiments demonstrate that LOSP outperforms the Heat Kernel and PageRank diffusions. Using LOSP as a subroutine, we further address the problem of multiple membership identification, which aims to find all the communities a single vertex belongs to. High F1 scores are achieved in detecting multiple local communities with respect to arbitrary single seed for various large real world networks.
更多查看译文
关键词
Community detection,Clustering,Local spectral subspace,Seed set expansion
AI 理解论文
溯源树
样例
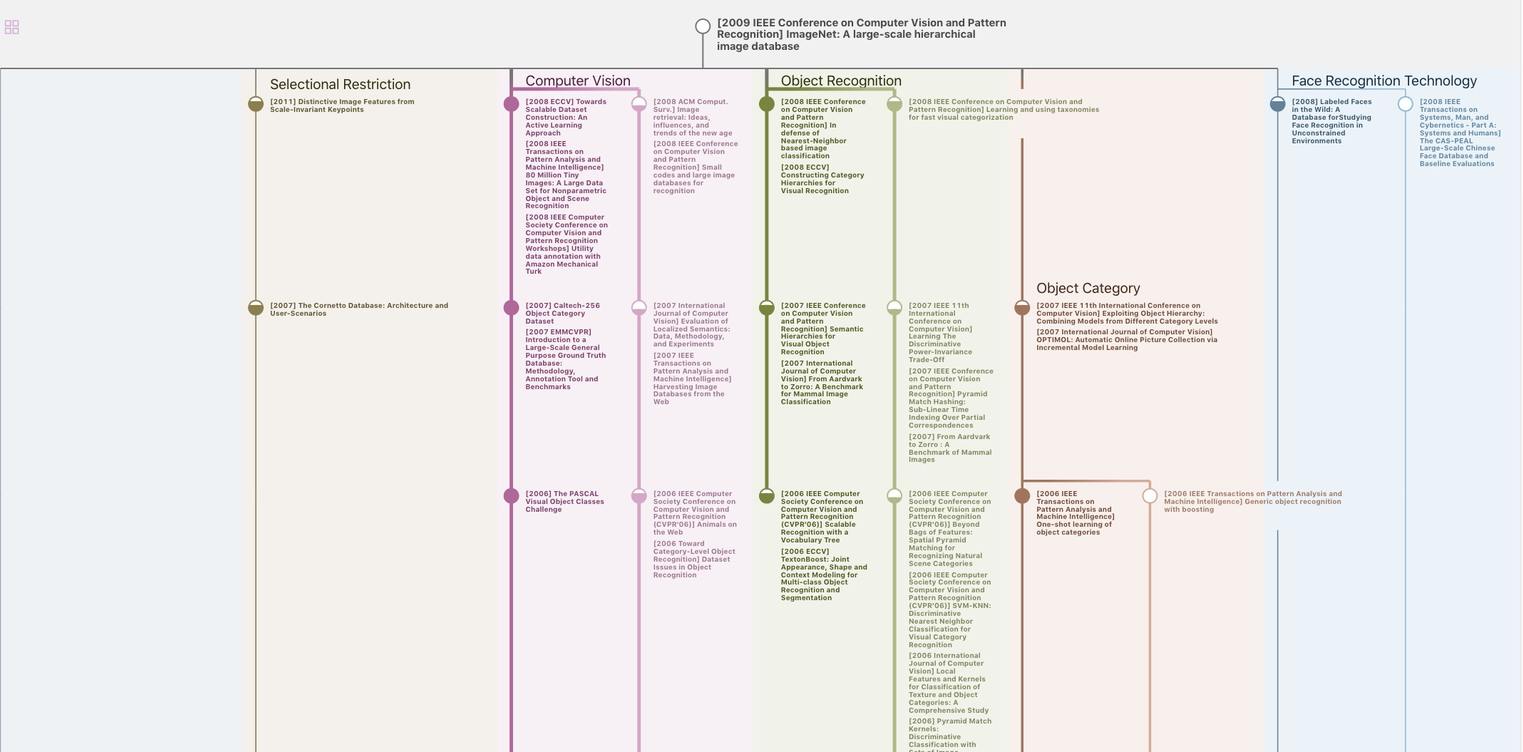
生成溯源树,研究论文发展脉络
Chat Paper
正在生成论文摘要