Soft-Hard Clustering for Multiview Data
Information Reuse and Integration(2015)
摘要
With rapid advances in technology and connectivity, the capability to capture data from multiple sources has given rise to multiview learning wherein each object has multiple representations and a learned model, whether supervised or unsupervised, needs to integrate these different representations. Multiview learning has shown to yield better predictive and clustering models, it also is able to provide a better insight into relationships between different views for making better decisions. In this paper, we consider the problem of multiview clustering and present a soft-hard clustering approach. In our approach, all object views are first independently mapped into a unit hypercube via soft clustering. The mapped views are next integrated via a hard clustering approach to yield the final results. Both soft and hard clustering stages utilize k-means or its variant c-means, which makes our method suitable for large-scale data problems. Furthermore, additional parallelization of the view mapping stage in parallel is possible, thus making the method more attractive for large-scale data applications. The performance of the method using three benchmark data sets is demonstrated and a comparison with other published results shows our method mostly yields a slightly better performance.
更多查看译文
关键词
soft-hard clustering,multiview data,multiview learning,object representation,unsupervised learning,predictive model,clustering model,object view,unit hypercube,k-means clustering,c-means clustering,large-scale data problem,view mapping parallelization,large-scale data application
AI 理解论文
溯源树
样例
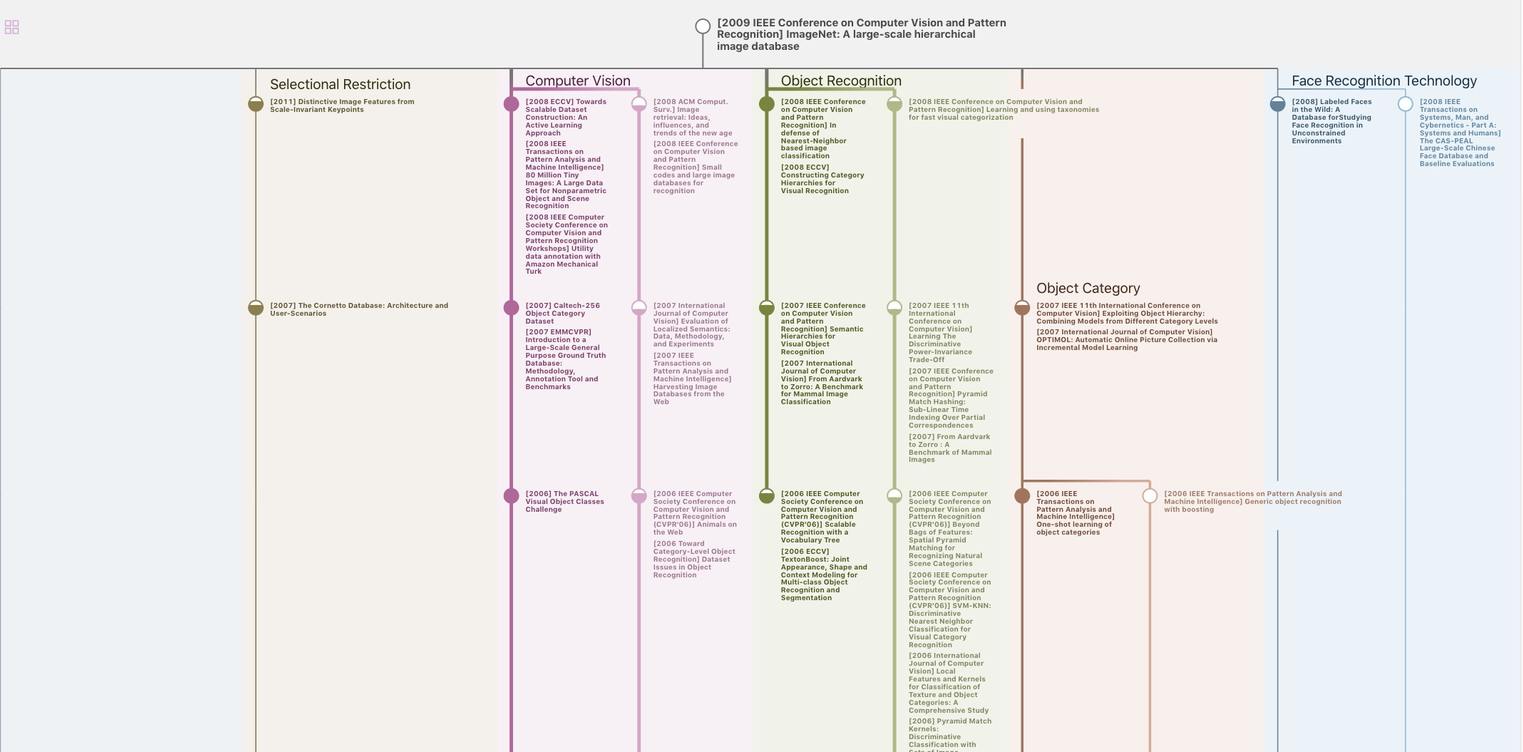
生成溯源树,研究论文发展脉络
Chat Paper
正在生成论文摘要