Transfer Learning For Bilingual Content Classification
KDD '15: The 21th ACM SIGKDD International Conference on Knowledge Discovery and Data Mining Sydney NSW Australia August, 2015(2015)
摘要
LinkedIn Groups provide a platform an which professionals with similar background, target and specialities can share content, take part in discussions and establish opinions on industry topics. As in most online social communities, spam content in LinkedIn Groups poses great challenges to the user experience and could eventually lead to substantial loss of active users. Building an intelligent and scalable span detection system is highly desirable but faces difficulties such as lack of labeled training data, particularly for languages other than English. In this paper, we take the spam (Spanish) job posting detection as the target problem and build a generic machine learning pipeline for multi-lingual spam detection. The main components are feature generation and knowledge migration via transfer learning. Specifically, in the feature generation phase, a relatively large labeled data set is generated via machine translation. Together with a large set of unlabeled human written Spanish data, unigram features are generated based on the frequency. In the second phase, machine translated data are properly reweighted to capture the discrepancy from human written ones and classifiers can be built on top of them. To make effective use of a small portion of labeled data available in human written Spanish, an adaptive transfer learning algorithm is proposed to further improve the performance. We evaluate the proposed method on LinkedIn's production data and the promising results verify the efficacy of our proposed algorithm. The pipeline is ready for production.
更多查看译文
关键词
transfer learning,classfication,text mining,NLP
AI 理解论文
溯源树
样例
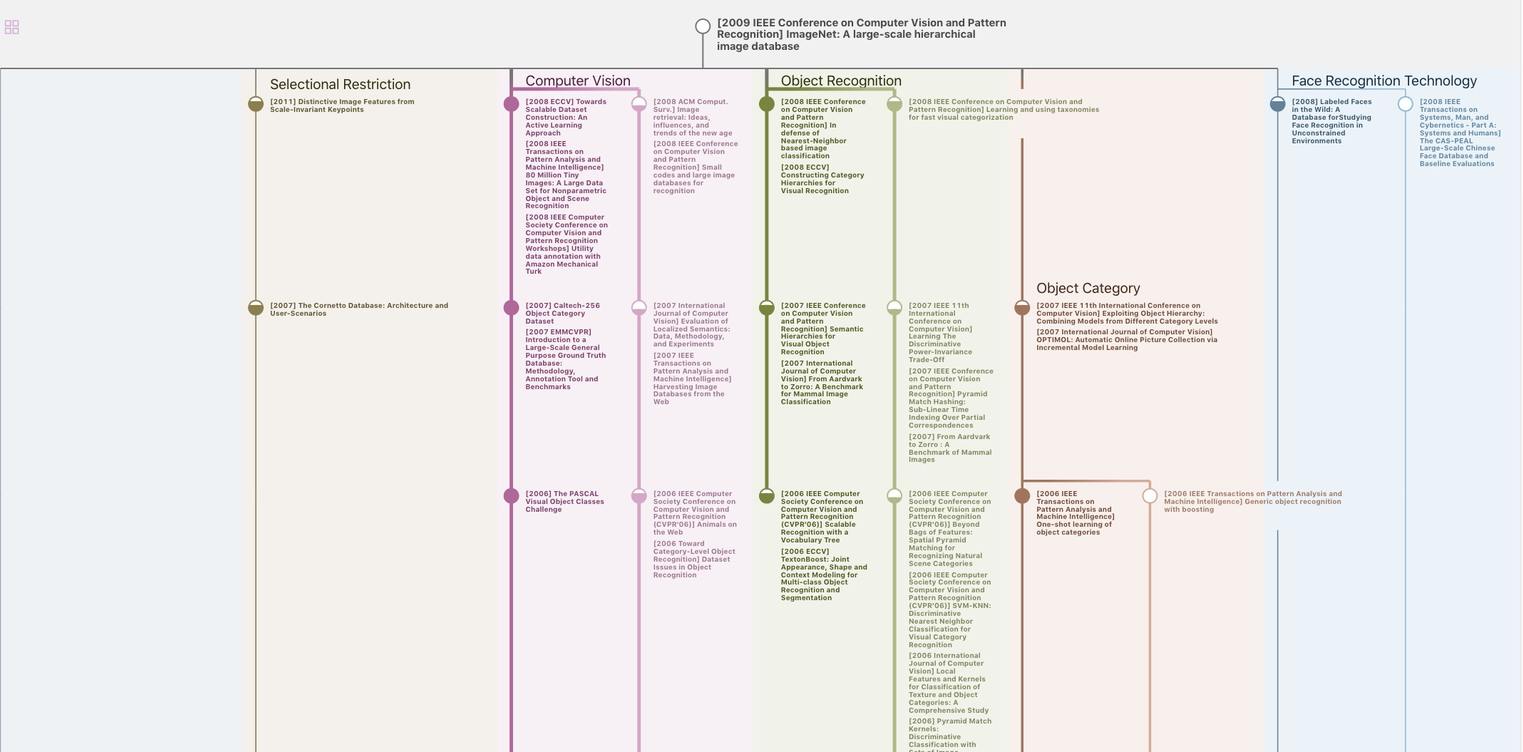
生成溯源树,研究论文发展脉络
Chat Paper
正在生成论文摘要