Client Clustering For Hiring Modeling In Work Marketplaces
KDD '15: The 21th ACM SIGKDD International Conference on Knowledge Discovery and Data Mining Sydney NSW Australia August, 2015(2015)
摘要
An important problem that online work marketplaces face is grouping clients into clusters, so that in each cluster clients are similar with respect 10 their luring criteria. Such a separation allows the marketplace to "learn" more accurately the hiring criteria in each cluster and recommend the right contractor to each client, for a successful collaboration. We propose a Maximum Likelihood definition of the "optimal" client clustering along with an efficient Expectation-Maximization clustering algorithm that can be applied in large marketplaces. Our results on the job hirings at oDesk over a seven-month period show that our client-clustering approach yields significant gains compared to "learning" the same hiring criteria for all clients. In addition, we analyze the clustering results to find interesting differences between the hiring criteria in the different groups of clients.
更多查看译文
AI 理解论文
溯源树
样例
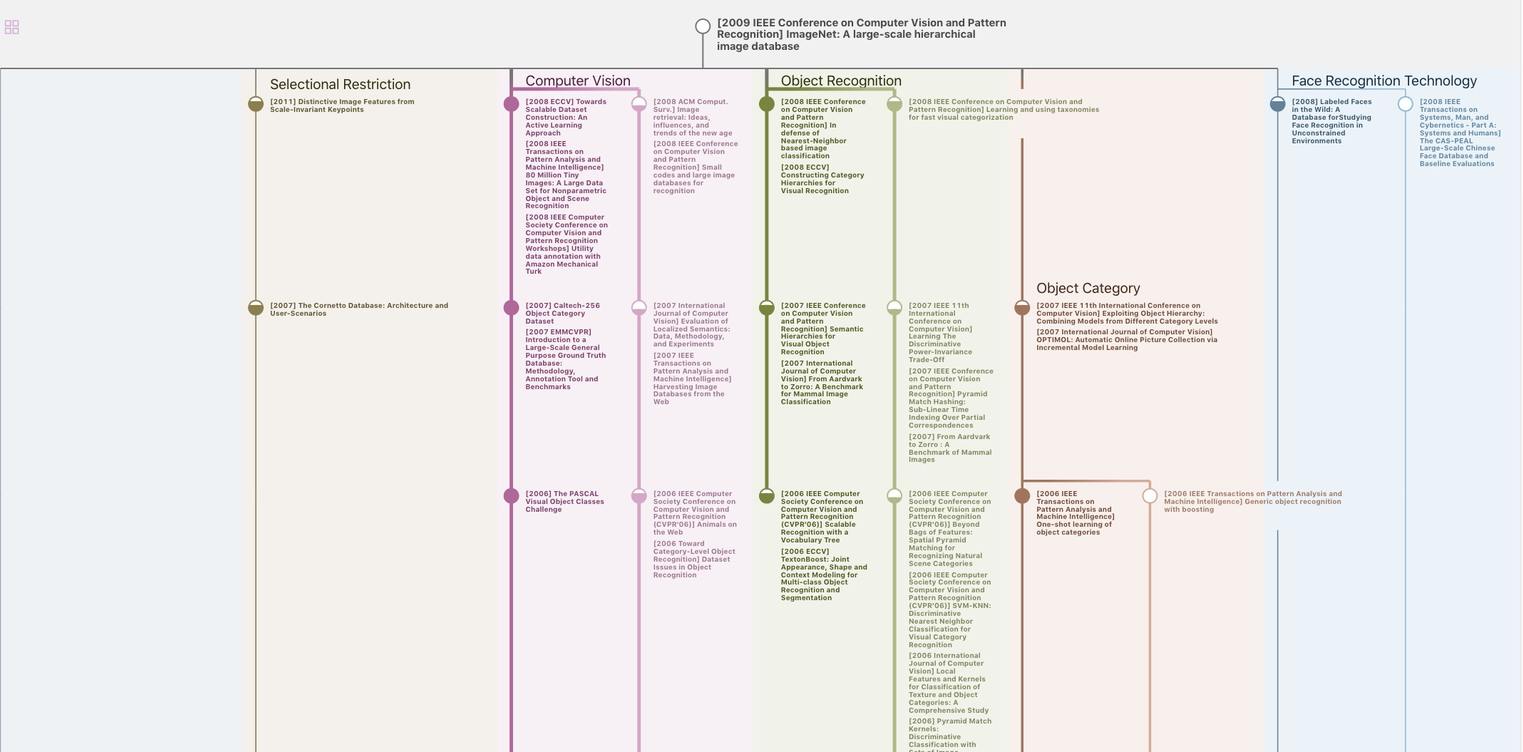
生成溯源树,研究论文发展脉络
Chat Paper
正在生成论文摘要