Instance Weighting For Patient-Specific Risk Stratification Models
KDD(2015)
摘要
Accurate risk models for adverse outcomes can provide important input to clinical decision-making. Surprisingly, one of the main challenges when using machine learning to build clinically useful risk models is the small amount of data available. Risk models need to be developed for specific patient populations, specific institutions, specific procedures, and specific outcomes. With each exclusion criterion, the amount of relevant training data decreases, until there is often an insufficient amount to learn an accurate model. This difficulty is compounded by the large class imbalance that is often present in medical applications.In this paper, we present an approach to address the problem of small data using transfer learning methods in the context of developing risk models for cardiac surgeries. We explore ways to build surgery-specific and hospital-specific models (the target task) using information from other kinds of surgeries and other hospitals (source tasks). We propose a novel method to weight examples based on their similarity to the target task training examples to take advantage of the useful examples while discounting less relevant ones.We show that incorporating appropriate source data in training can lead to improved performance over using only target task training data, and that our method of instance weighting can lead to further improvements. Applied to a surgical risk stratification task, our method, which used data from two institutions, performed comparably to the risk model published by the Society for Thoracic Surgeons, which was developed and tested on over one hundred thousand surgeries from hundreds of institutions.
更多查看译文
关键词
Risk stratification models,transfer learning,instance weighting
AI 理解论文
溯源树
样例
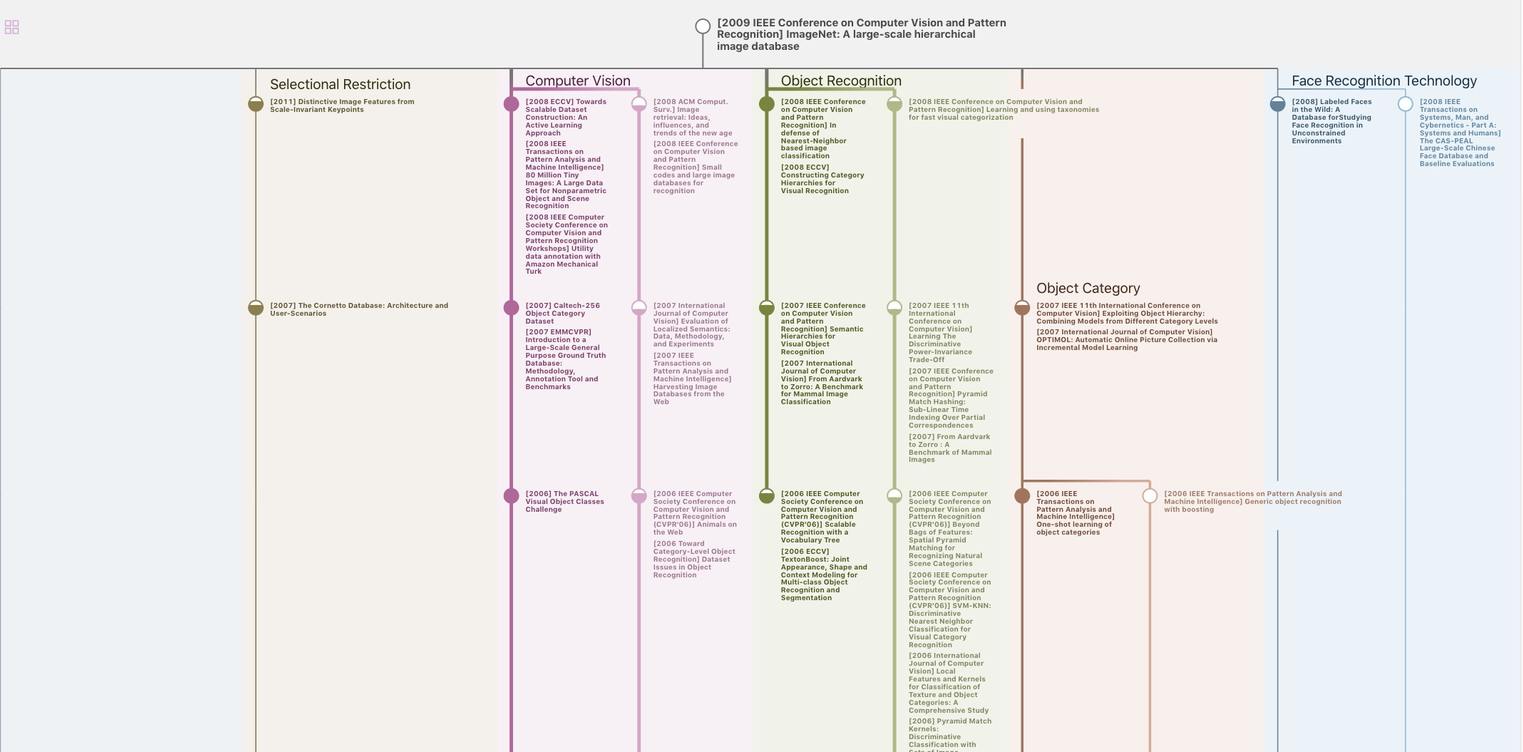
生成溯源树,研究论文发展脉络
Chat Paper
正在生成论文摘要