Hard Drive Failure Prediction Using Big Data
SRDS Workshop(2015)
摘要
We design a general framework named Hdoctor for hard drive failure prediction. Hdoctor leverages the power of big data to achieve a significant improvement comparing to all previous researches that used sophisticated machine learning algorithms. Hdoctor exhibits a series of engineering innovations: (1) constructing time dependent features to characterize the Self-Monitoring, Analysis and Reporting Technology (SMART) value transitions during disk failures, (2) combining features to enable the model to learn the correlation among different SMART attributes, (3) regarding circumstance data such as cluster workload, temperature, humidity, location as related features. Meanwhile, Hdoctor collects/labels samples and updates model automatically, and works well for all kinds of disk failure prediction in our intelligent data center. In this work, we use Hdoctor to collect 74,477,717 training records from our clusters involving 220,022 disks. By training a simple and scalable model, our system achieves a detection rate of 97.82%, with a false alarm rate (FAR) of 0.3%, which hugely outperforms all previous algorithms. In addition, Hdoctor is an excellent indicator for how to predict different hardware failures efficiently under various circumstances.
更多查看译文
关键词
hard drive failure prediction,Big Data,Hdoctor framework,self-monitoring analysis and reporting technology SMART,false alarm rate,FAR
AI 理解论文
溯源树
样例
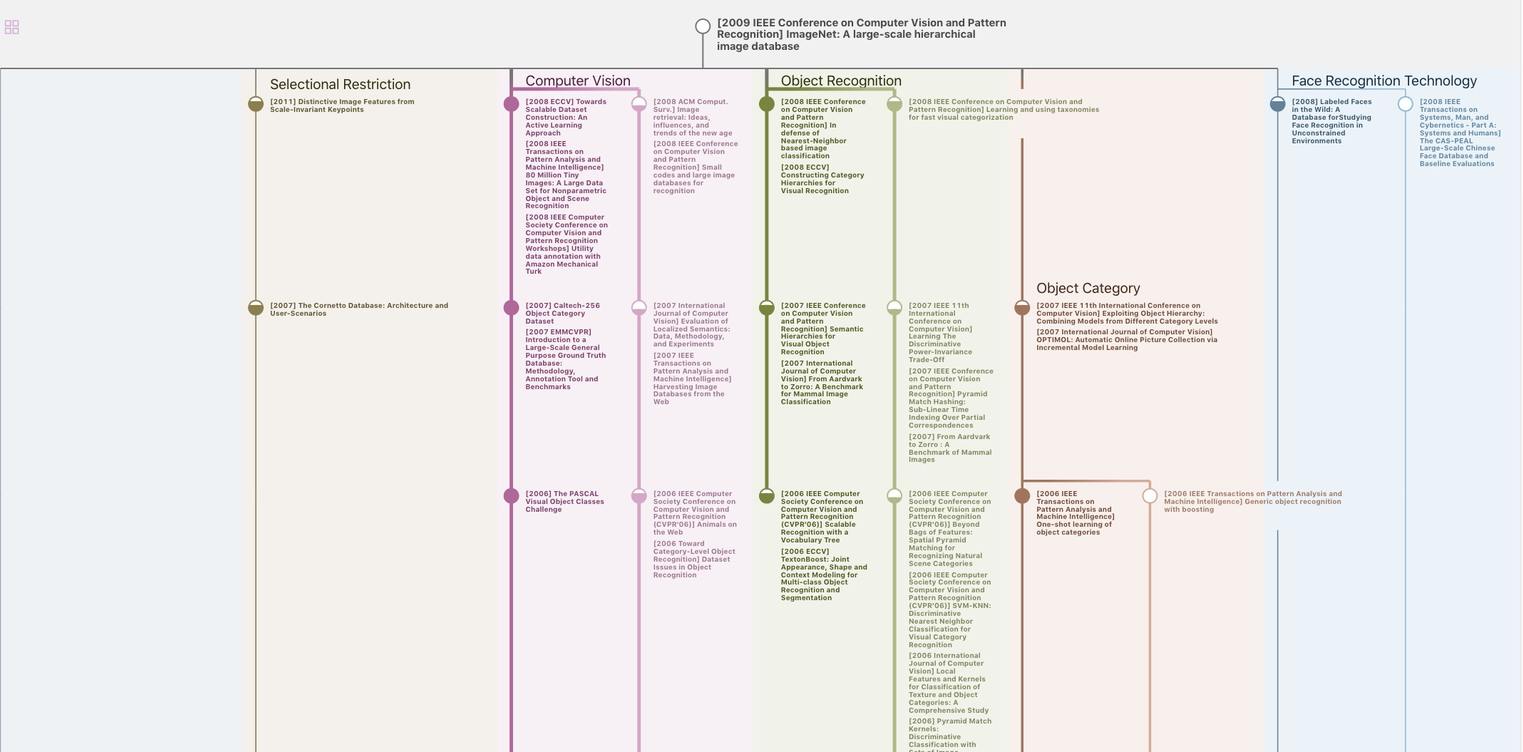
生成溯源树,研究论文发展脉络
Chat Paper
正在生成论文摘要