Cluster-based efficient particle PHD filter
2015 International Conference on Control, Automation and Information Sciences (ICCAIS)(2015)
摘要
Particle probability hypothesis density filtering has become a tractable means for multi-target tracking due to its capability of handling an unknown and time-varying number of targets in non-linear or non-Gaussian system in the presence of clutter and missing measurements. However, it is time-consuming because hundreds of thousands of particles are required to reach a satisfactory tracking accuracy, thus improving its efficiency is still a high challenge. One of major time-costly processing in the particle PHD lies in the updating step. To overcome this difficulty, this paper presents a clustering-based update scheme for the particle PHD filter, the key to this method is to efficiently find the measurements which make little contribution to each particle weight based on clustering and eliminate them when updating particle weights. Experiment shows that the proposed particle PHD filter reaches similar accuracy to the traditional particle PHD filter but with less computational costs.
更多查看译文
关键词
multi-target tracking,probability hypothesis density,particle filter,high speedup
AI 理解论文
溯源树
样例
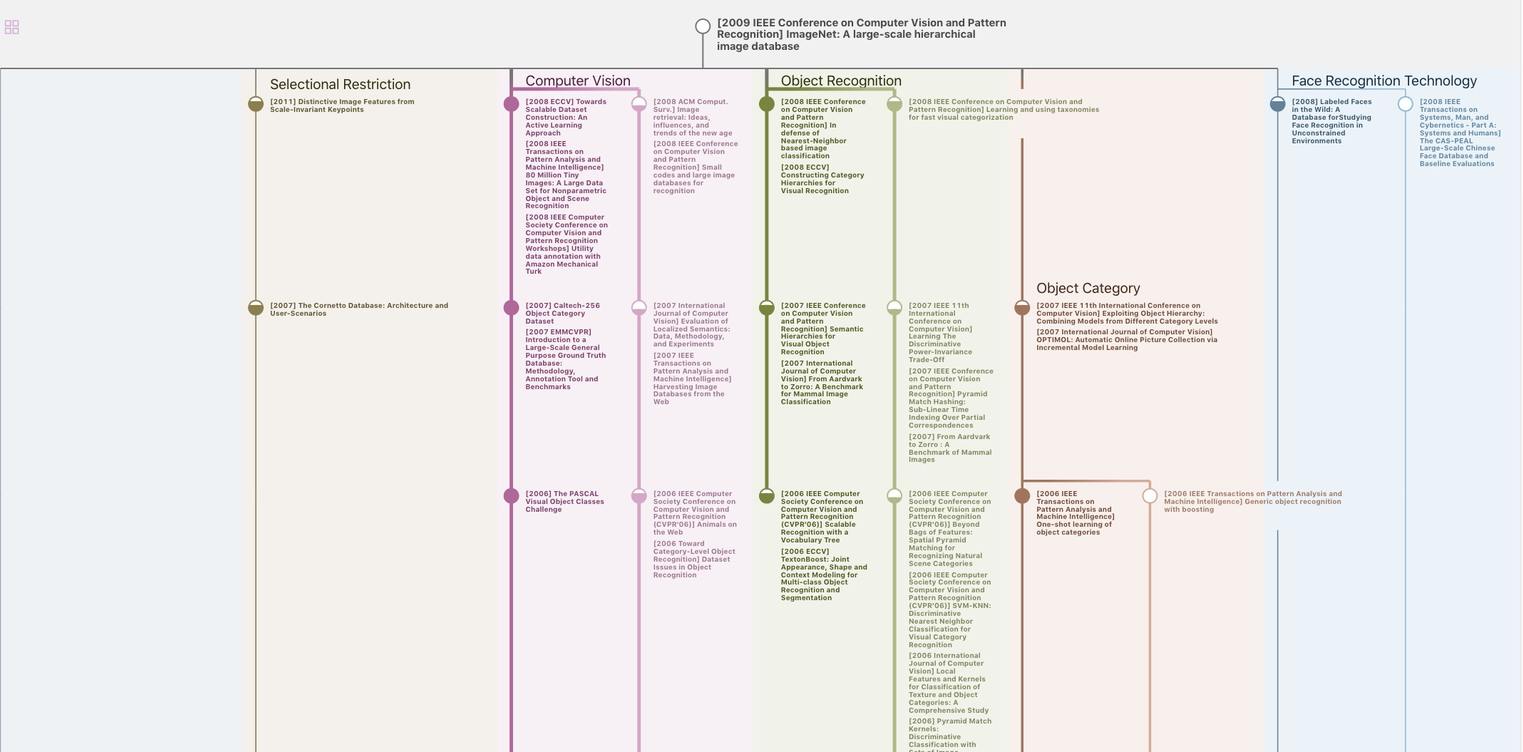
生成溯源树,研究论文发展脉络
Chat Paper
正在生成论文摘要