Big Text Visual Analytics in Sensemaking
2015 Big Data Visual Analytics (BDVA)(2015)
摘要
Learning from text data often involves a loop of tasks that iterate between foraging for information and synthesizing it in incremental hypotheses. Past research has shown the advantages of using spatial workspaces as a means for synthesizing information through externalizing hypotheses and creating spatial schemas. However, spatializing the entirety of datasets becomes prohibitive as the number of documents available to the analysts grows, particularly when only a small subset are relevant to the tasks at hand. To address this issue, we applied the multi-model semantic interaction (MSI) technique, which leverages user interactions to aid in the display layout (as was seen in previous semantic interaction work), forage for new, relevant documents as implied by the interactions, and place them in context of the user's existing spatial layout. Thus, this approach cleanly embeds visual analytics of big text collections directly into the human sensemaking process.
更多查看译文
关键词
human sensemaking process,big text collection,MSI technique,multimodel semantic interaction technique,learning,big text visual analytics
AI 理解论文
溯源树
样例
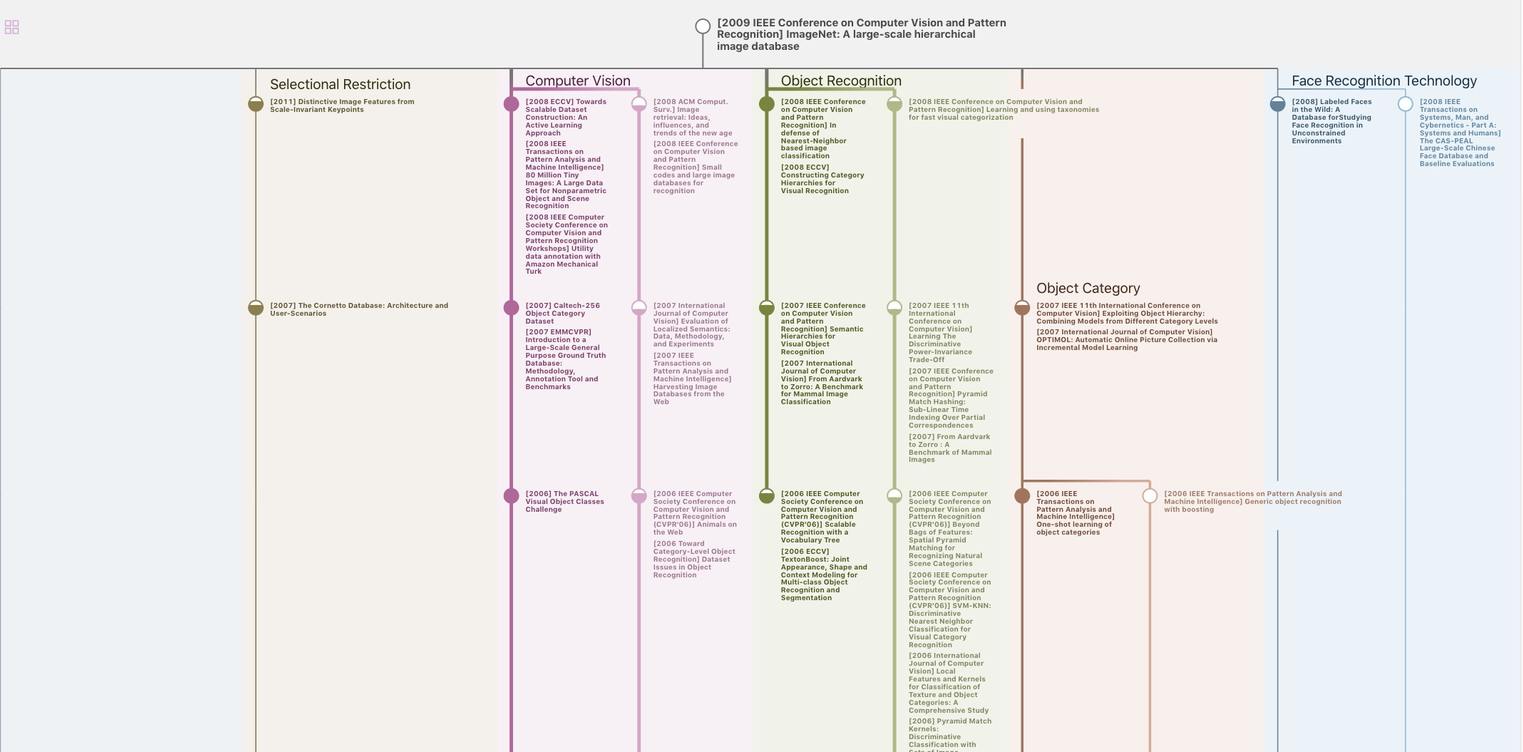
生成溯源树,研究论文发展脉络
Chat Paper
正在生成论文摘要