Independent Joint Learning In Practice: Local Error Estimates To Improve Inverse Dynamics Control
2015 IEEE-RAS 15TH INTERNATIONAL CONFERENCE ON HUMANOID ROBOTS (HUMANOIDS)(2015)
摘要
Independent Joint Learning (IJL) was recently introduced as a learning-based approach to account for inverse dynamics (ID) model errors. The fundamental idea is to combine an ID model with learned torque error estimators that only rely on joint-local information. This approach improves task-to-task generalization and reduces learning times as each torque error estimators depends only on the state of a single joint instead of the global configuration.Herein, we adapt the IJL method to a real robotic platform, namely the COMAN compliant humanoid robot. We test the algorithm under different loading conditions in open and closed loop control (PD, forward non-linear control, and ID control). In our implementation, IJL becomes a flexible component that fits in between the output of an existing computed-torque controller and low-level motor drivers.Our results show that IJL reduces torque estimation errors in the open loop case and improves tracking performance in the closed loop case. Under varying loading conditions, IJL's performance is on par with and in some cases exceeds the adapted model (i.e. a modified ID model with updated inertial parameters).Finally, the compartmented design and limited number of assumptions of the algorithm allow it to be easily integrated into existing platforms.
更多查看译文
关键词
independent joint learning,local error estimates,inverse dynamics control improvement,IJL,learning-based approach,ID model errors,torque error estimators,joint-local information,task-to-task generalization improvement,learning time reduction,global configuration,COMAN compliant humanoid robot,loading conditions,open loop control,closed loop control,PD control,forward nonlinear control,ID control,computed-torque controller,low-level motor drivers,modified ID model,updated inertial parameters
AI 理解论文
溯源树
样例
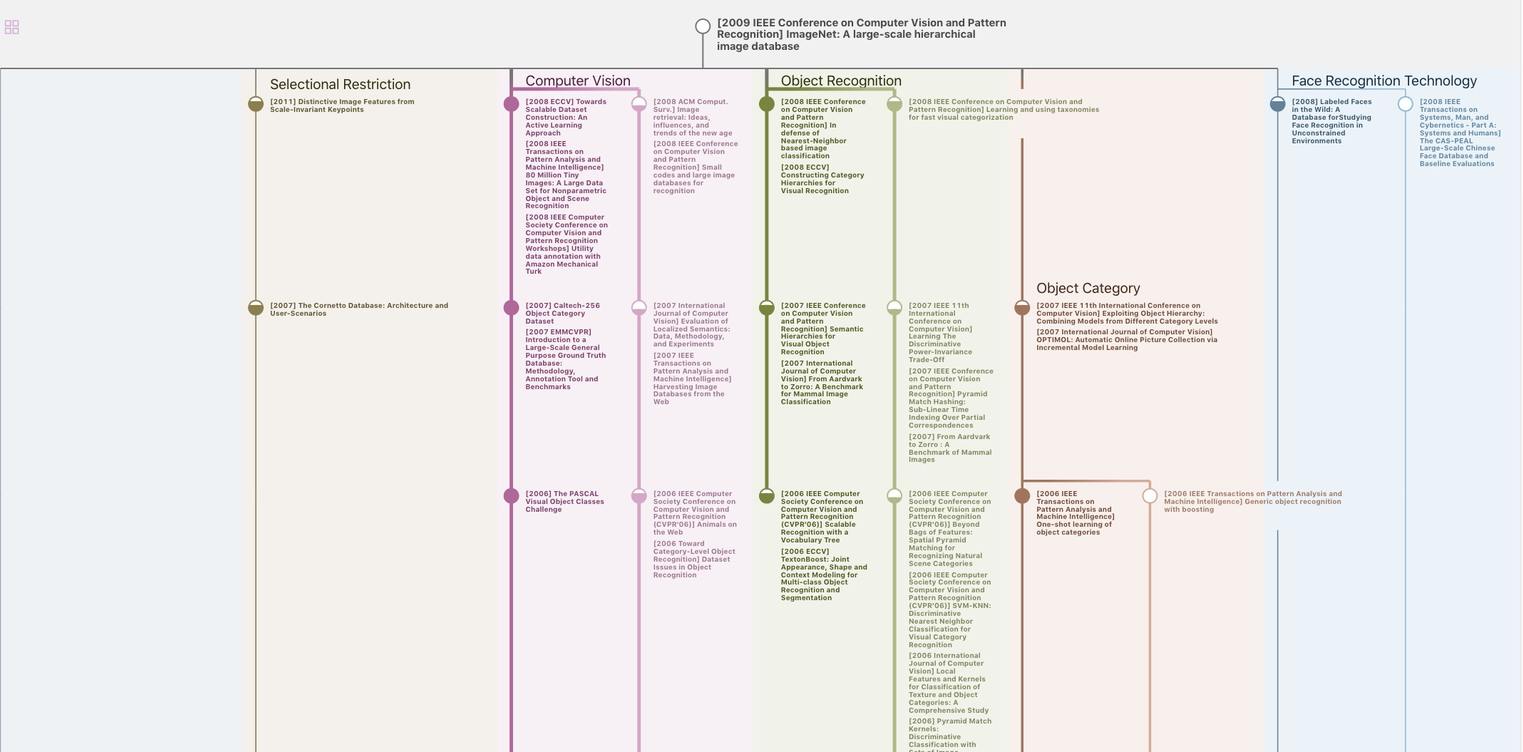
生成溯源树,研究论文发展脉络
Chat Paper
正在生成论文摘要