Detecting Clusters of Fake Accounts in Online Social Networks.
CCS(2015)
摘要
ABSTRACTFake accounts are a preferred means for malicious users of online social networks to send spam, commit fraud, or otherwise abuse the system. A single malicious actor may create dozens to thousands of fake accounts in order to scale their operation to reach the maximum number of legitimate members. Detecting and taking action on these accounts as quickly as possible is imperative in order to protect legitimate members and maintain the trustworthiness of the network. However, any individual fake account may appear to be legitimate on first inspection, for example by having a real-sounding name or a believable profile. In this work we describe a scalable approach to finding groups of fake accounts registered by the same actor. The main technique is a supervised machine learning pipeline for classifying {\em an entire cluster} of accounts as malicious or legitimate. The key features used in the model are statistics on fields of user-generated text such as name, email address, company or university; these include both frequencies of patterns {\em within} the cluster (e.g., do all of the emails share a common letter/digit pattern) and comparison of text frequencies across the entire user base (e.g., are all of the names rare?). We apply our framework to analyze account data on LinkedIn grouped by registration IP address and registration date. Our model achieved AUC 0.98 on a held-out test set and AUC 0.95 on out-of-sample testing data. The model has been productionalized and has identified more than 250,000 fake accounts since deployment.
更多查看译文
AI 理解论文
溯源树
样例
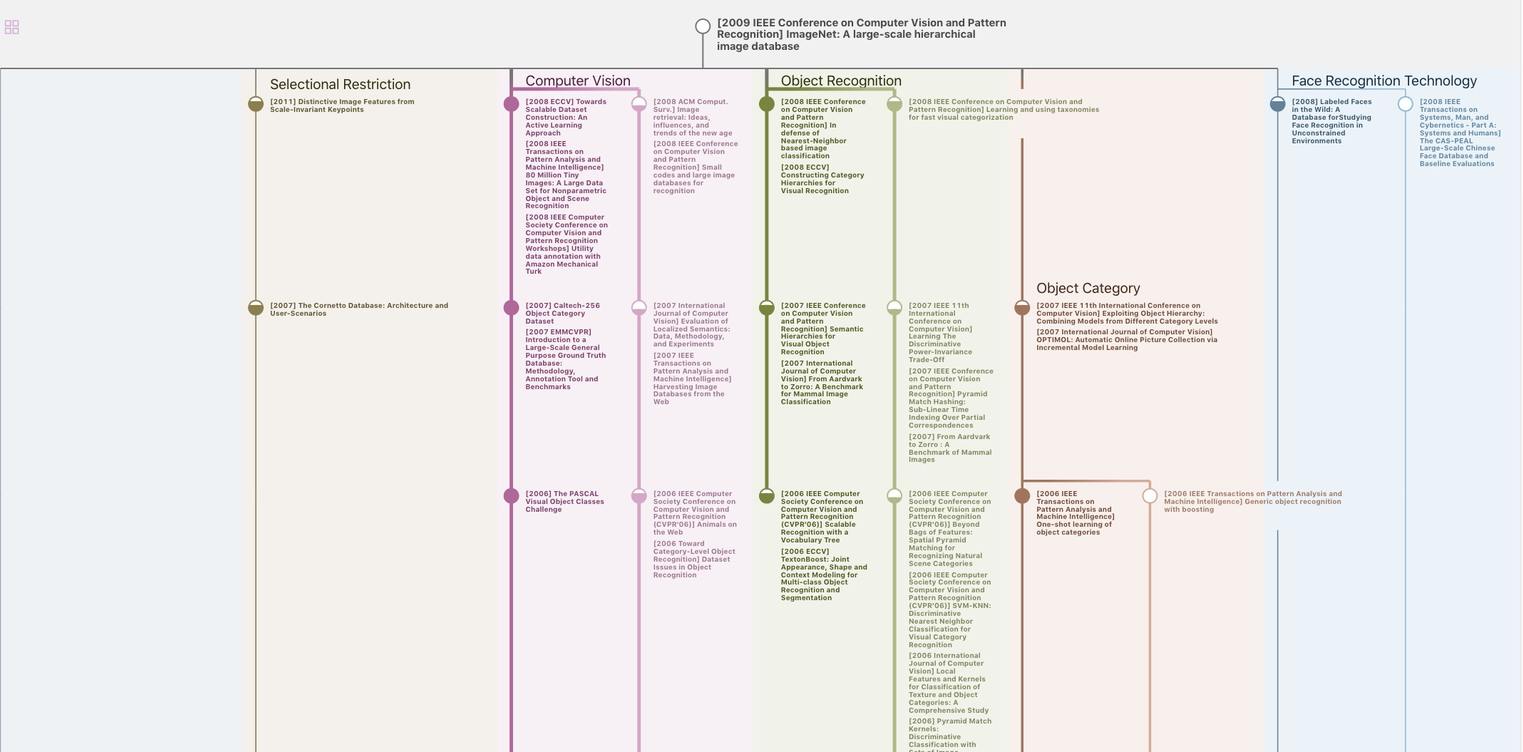
生成溯源树,研究论文发展脉络
Chat Paper
正在生成论文摘要