Unbiased Ranking Evaluation On A Budget
WWW '15: 24th International World Wide Web Conference Florence Italy May, 2015(2015)
摘要
We address the problem of assessing the quality of a ranking system (e.g., search engine, recommender system, review ranker) given a fixed budget for collecting expert judgments. In particular, we propose a method that selects which items to judge in order to optimize the accuracy of the quality estimate. Our method is not only efficient, but also provides estimates that are unbiased - unlike common approaches that tend to underestimate performance or that have a bias against new systems that are evaluated re-using previous relevance scores [1]. Our method is based on the insight that we can write many common performance measures as expectations, and then use Monte Carlo techniques, such as importance sampling, to estimate these expectations [1].We compare against the traditional approach of ranking evaluation under budget constraints that is employed in the pooling method used in TREC [8]. Instead of judging all queries to their full depths, only the top k (e.g., k = 100) documents for each query are judged until the budget is exhausted. While for small document collections it is reasonable to assume that all relevant documents are within the top k documents, this working hypothesis is less valid for larger collections [3]. More complicated approaches include stratified sampling or greedy sample selection [11, 2], but usually result in algorithms that are difficult to apply for practitioners. Somewhat related to our method is the scenario in which one wants to re-use interaction logs of a system for evaluation [6, 7] or data from logged interleaving experiments [4].Our contributions are as follows. First, we show how to get an unbiased estimator for Discounted Cumulative Gain (DCG) [5] using importance sampling. Second, we outline a simple proposal for selecting the sampling distribution. Lastly, we compare our method to two traditional approaches and show that it is vastly superior in terms of bias and accuracy.
更多查看译文
AI 理解论文
溯源树
样例
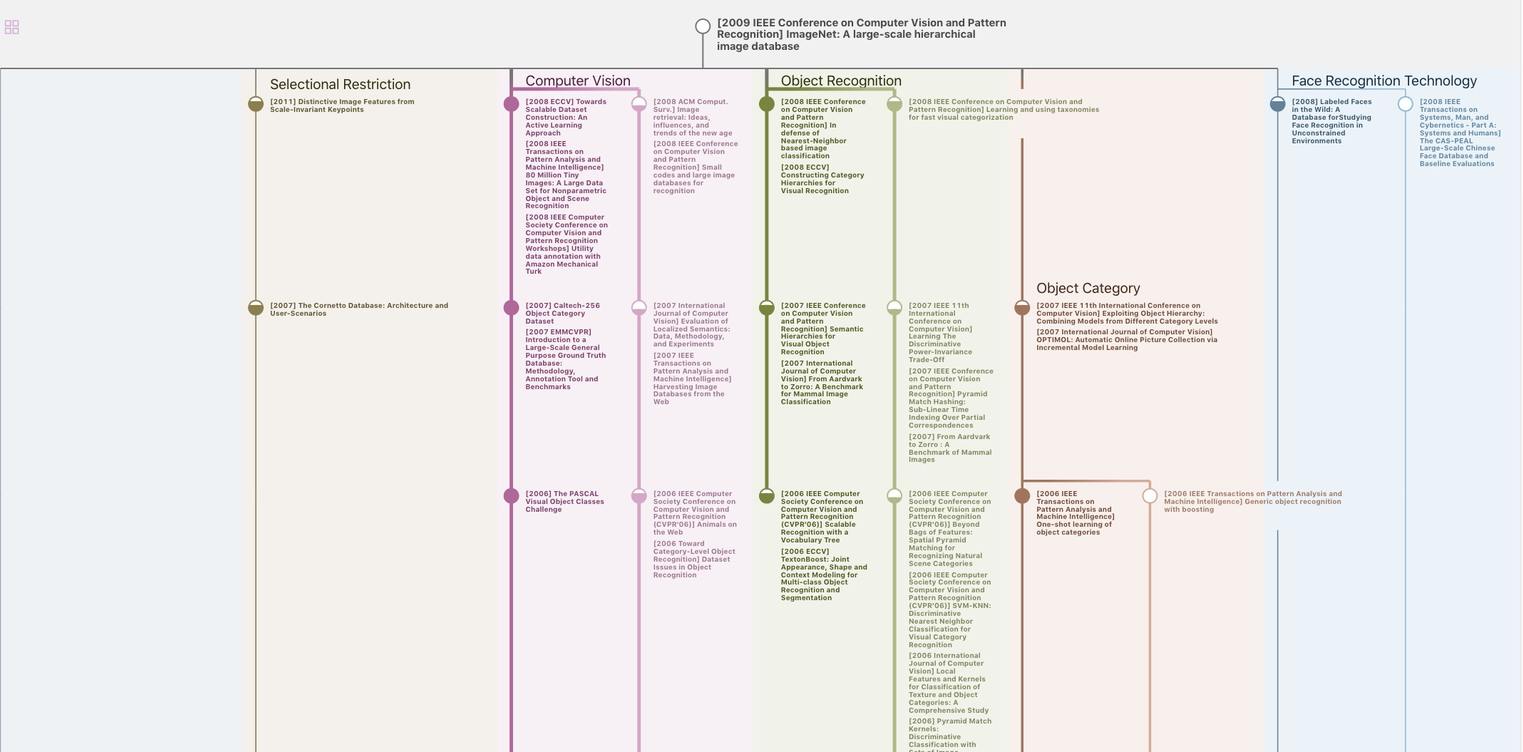
生成溯源树,研究论文发展脉络
Chat Paper
正在生成论文摘要