Pct: Partial Co-Alignment Of Social Networks
WWW '16: 25th International World Wide Web Conference Montréal Québec Canada April, 2016(2016)
摘要
People nowadays usually participate in multiple online social networks simultaneously to enjoy more social network services. Besides the common users, social networks providing similar services can also share many other kinds of information entities, e.g., locations, videos and products. However, these shared information entities in different networks are mostly isolated without any known corresponding connections. In this paper, we aim at inferring such potential corresponding connections linking multiple kinds of shared entities across networks simultaneously. Formally, the problem is referred to as the network "Partial Co-alignmenT" (PCT) problem. PCT is an important problem and can be the prerequisite for many concrete cross-network applications, like social network fusion, mutual information exchange and transfer. Meanwhile, the PCT problem is also very challenging to address due to various reasons, like (1) the heterogeneity of social networks, (2) lack of training instances to build models, and (3) one-to-one constraint on the correspondence connections. To resolve these challenges, a novel unsupervised network alignment framework, UNICOAT (UNsupervIsed COncurrent AlignmenT)), is introduced in this paper. Based on the heterogeneous information, uNiCOAT transforms the PCT problem into a joint optimization problem. To solve the objective function, the one-to-one constraint on the corresponding relationships is relaxed, and the redundant non-existing corresponding connections introduced by such a relaxation will be pruned with a novel network co-matching algorithm proposed in this paper. Extensive experiments conducted on real-world co aligned social network datasets demonstrate the effectiveness of uNiC OAT in addressing the PCT problem.
更多查看译文
关键词
Partial Network Co-Alignment,Multiple Heterogeneous Social Networks,Unsupervised Learning,Data Mining
AI 理解论文
溯源树
样例
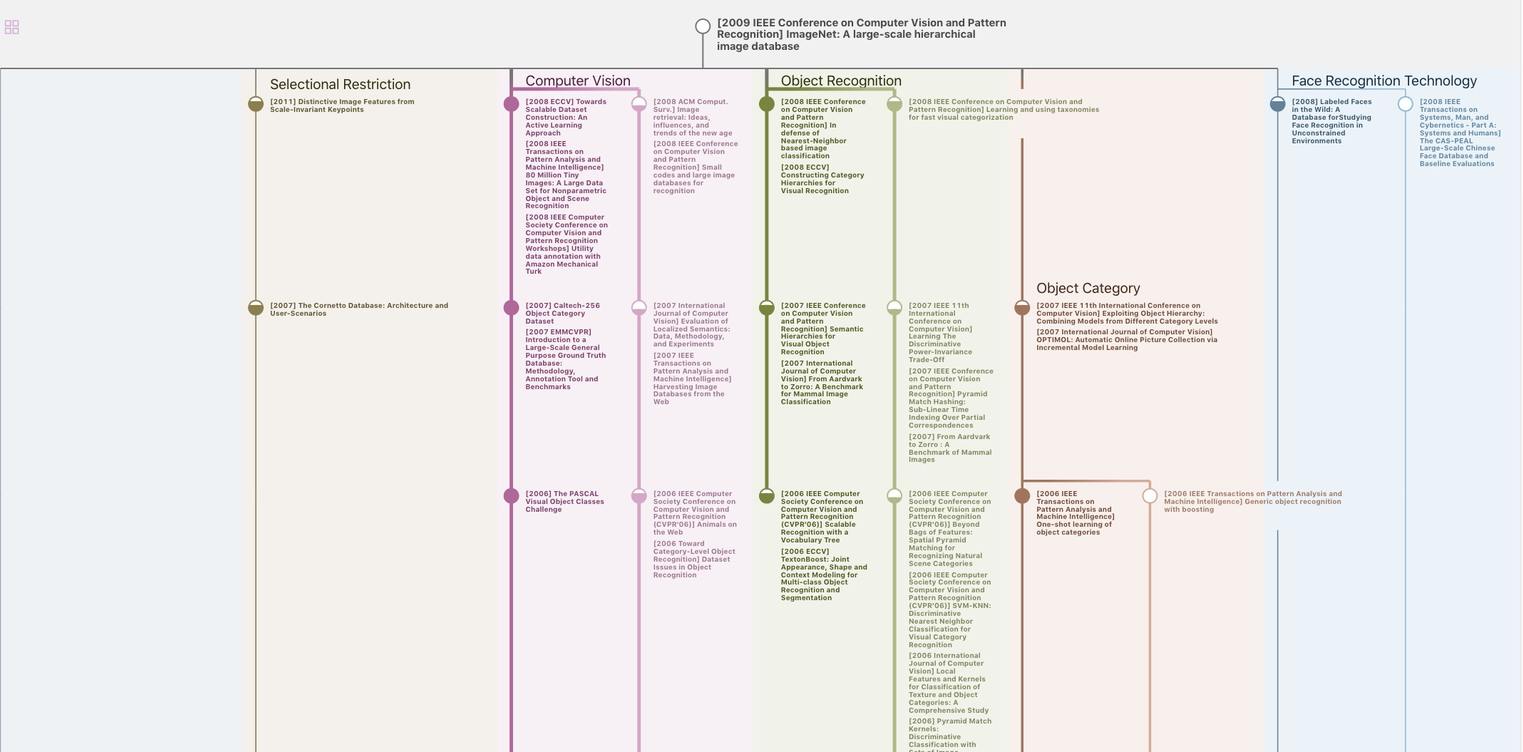
生成溯源树,研究论文发展脉络
Chat Paper
正在生成论文摘要