Subjective Similarity: Personalizing Alternative Item Recommendations
WWW '15: 24th International World Wide Web Conference Florence Italy May, 2015(2015)
摘要
We present a new algorithm for recommending alternatives to a given item in an e-commerce setting. Our algorithm is an incremental improvement over an earlier system, which recommends similar items by first assigning the input item to clusters and then selecting best quality items within those clusters. The original algorithm does not consider the recent context and our new algorithm improves the earlier system by personalizing the recommendations to user intentions. The system measures user intention using the recent queries, which are used to determine the level of abstraction in similarity and relative importance of similarity dimensions. We show that user engagement increases when recommended item titles share more terms with most recent queries. Moreover, the new algorithm increases query coverage without sacrificing input item similarity and item quality.
更多查看译文
关键词
eCommerce,Recommender Systems,Personalization,Context-aware alternative item recommendations
AI 理解论文
溯源树
样例
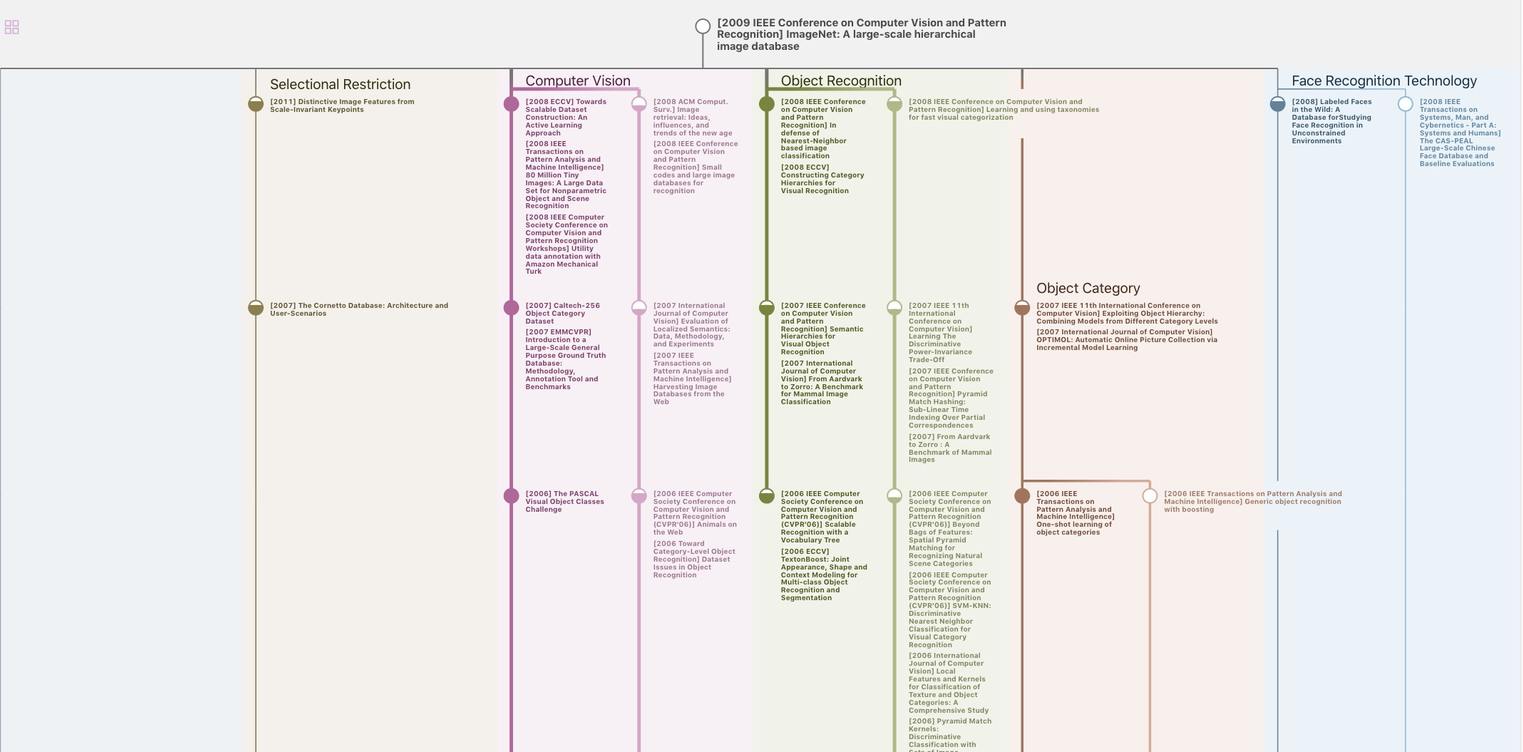
生成溯源树,研究论文发展脉络
Chat Paper
正在生成论文摘要