N-Gram Over Context
WWW '16: Proceedings of the 25th International Conference on World Wide Web(2016)
摘要
Our proposal, N-gram over Context (NOC), is a nonparametric topic model that aims to help our understanding of a given corpus, and be applied to many text mining applications. Like other topic models, NOC represents each document as a mixture of topics and generates each word from one topic. Unlike these models, NOC focuses on both a topic structure as an internal linguistic structure, and N-gram as an external linguistic structure. To improve the quality of topic specific N-grams, NOC reveals a tree of topics that captures the semantic relationship between topics from a given corpus as context, and forms N-gram by offering power-law distributions for word frequencies on this topic tree. To gain both these linguistic structures efficiently, NOC learns them from a given corpus in a unified manner. By accessing this entire tree at the word level in the generative process of each document, NOC enables each document to maintain a thematic coherence and form N-grams over context. We develop a parallelizable inference algorithm, DNOC, to support large data sets. Experiments on review articles/papers/tweet show that NOC is useful as a generative model to discover both the topic structure and the corresponding N-grams, and well complements human experts and domain specific knowledge. D-NOC can process large data sets while preserving full generative model performance, by the help of an open-source distributed machine learning framework.
更多查看译文
关键词
Nonparametric models,Topic models,Latent variable models,Graphical models,N-gram topic model,MapReduce
AI 理解论文
溯源树
样例
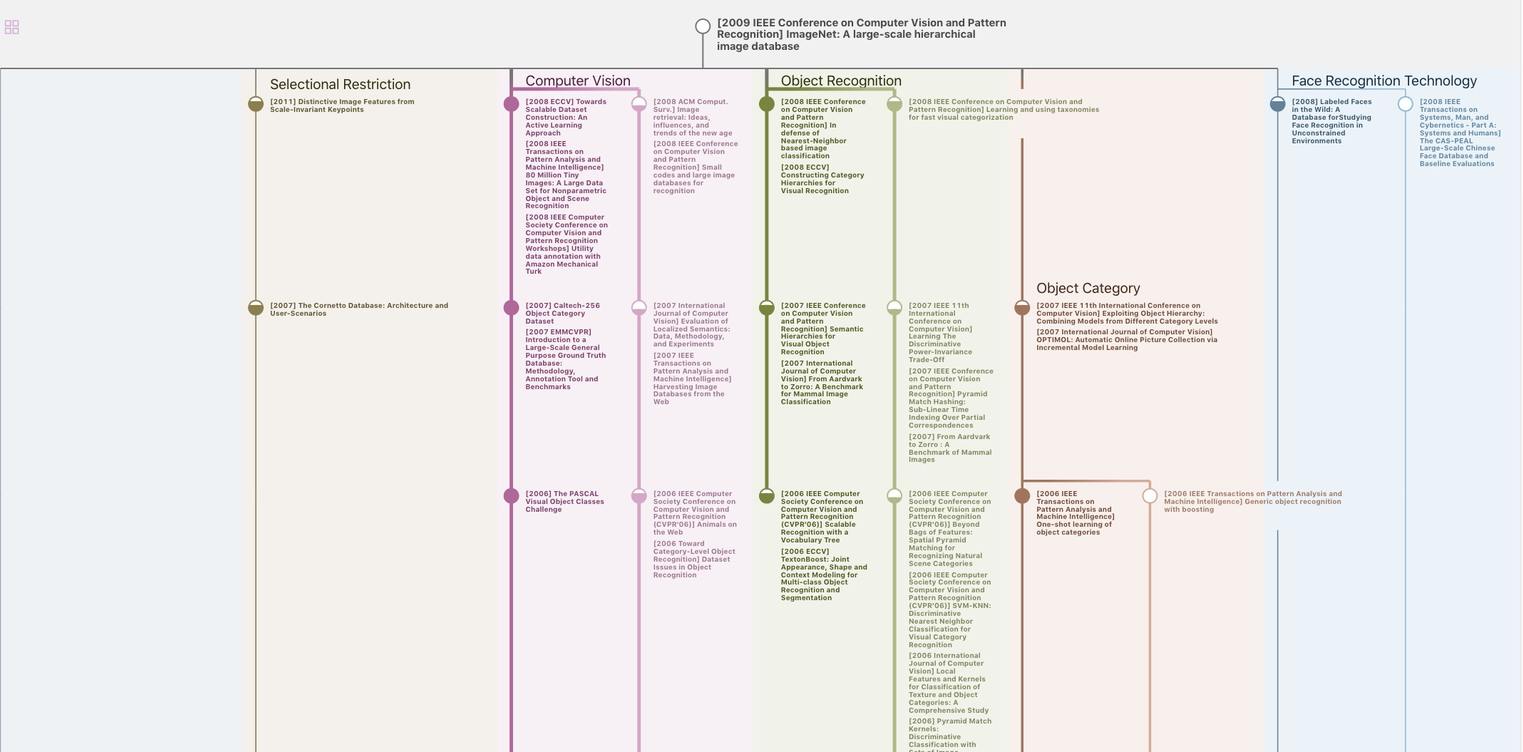
生成溯源树,研究论文发展脉络
Chat Paper
正在生成论文摘要