On The Relationship Between Visual Attributes And Convolutional Networks
CVPR(2015)
摘要
One of the cornerstone principles of deep models is their abstraction capacity, i.e. their ability to learn abstract concepts from 'simpler' ones. Through extensive experiments, we characterize the nature of the relationship between abstract concepts (specifically objects in images) learned by popular and high peiforming convolutional networks (conv-nets) and established mid-level representations used in computer vision (specifically semantic visual attributes). We focus on attributes due to their impact on several applications, such as object description, retrieval and mining, and active (and zero-shot) learning. Among the findings we uncover, we show empirical evidence of the existence of Attribute Centric Nodes (ACNs) within a conv-net, which is trained to recognize objects (not attributes) in images. These special conv-net nodes (1) collectively encode information pertinent to visual attribute representation and discrimination, (2) are unevenly and sparsely distribution across all layers of the conv-net, and (3) play an important role in conv-net based object recognition.
更多查看译文
关键词
semantic visual attribute,convolutional network,computer vision,attribute centric node,ACN,object recognition
AI 理解论文
溯源树
样例
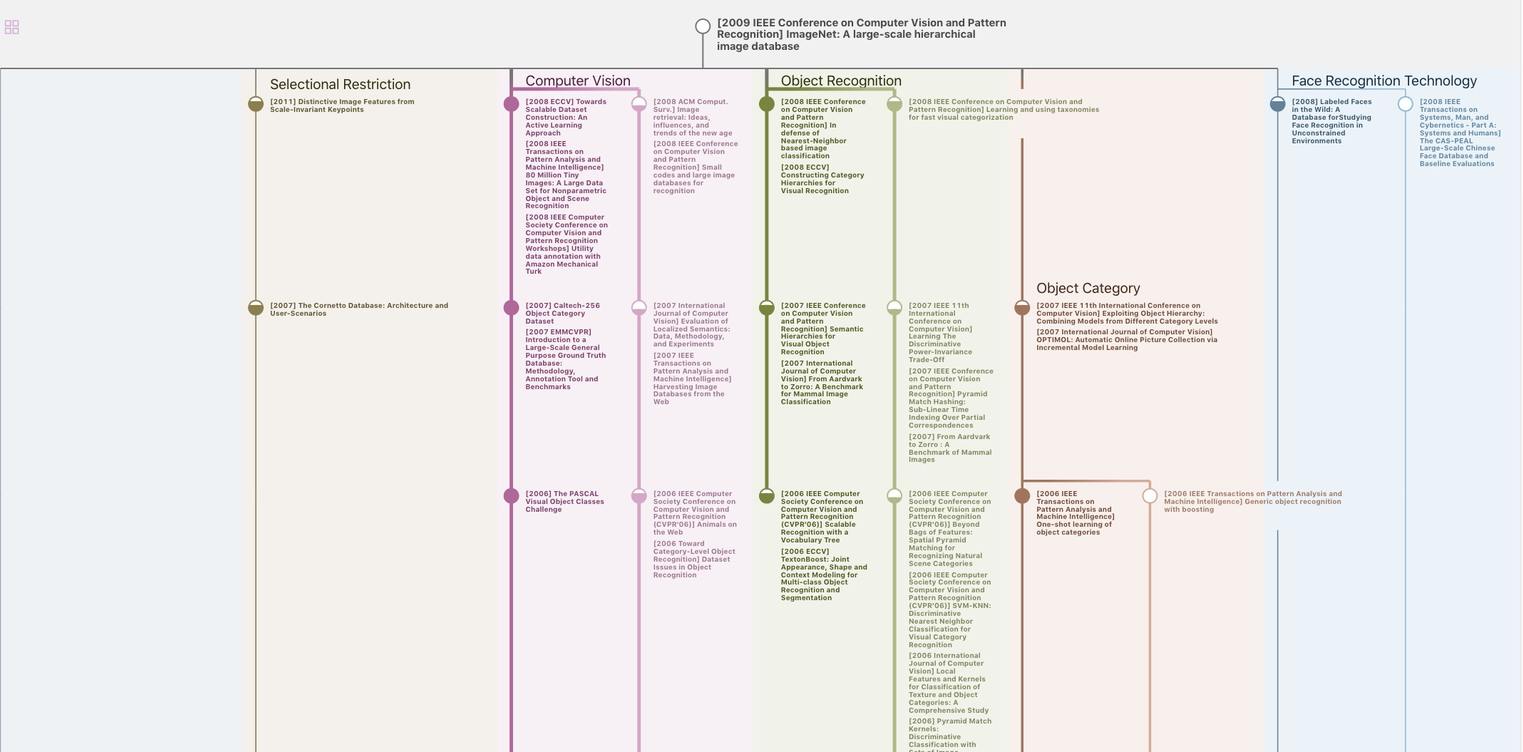
生成溯源树,研究论文发展脉络
Chat Paper
正在生成论文摘要