Approximate partitioning of observations in hierarchical particle filter body tracking
CVPR 2011 WORKSHOPS(2011)
摘要
This paper presents a model-based hierarchical particle filtering algorithm to estimate the pose and anthropometric parameters of humans in multi-view environments. Our method incorporates a novel likelihood measurement approach consisting of an approximate partitioning of observations. Provided that a partitioning of the human body model has been defined and associates body parts to state space variables, the proposed method estimates image regions that are relevant to that body part and thus to the state space variables of interest. The proposed regions are bounding boxes and consequently can be efficiently processed in a GPU. The algorithm is tested in a challenging dataset involving people playing tennis (TennisSense) and also in the well-known HumanEva dataset. The obtained results show the effectiveness of the proposed method.
更多查看译文
关键词
approximate partitioning,hierarchical particle filter body tracking,model-based hierarchical particle filtering algorithm,pose estimation,anthropometric parameter estimation,likelihood measurement approach,state space variables,GPU,graphical processing unit,TennisSense,HumanEva dataset
AI 理解论文
溯源树
样例
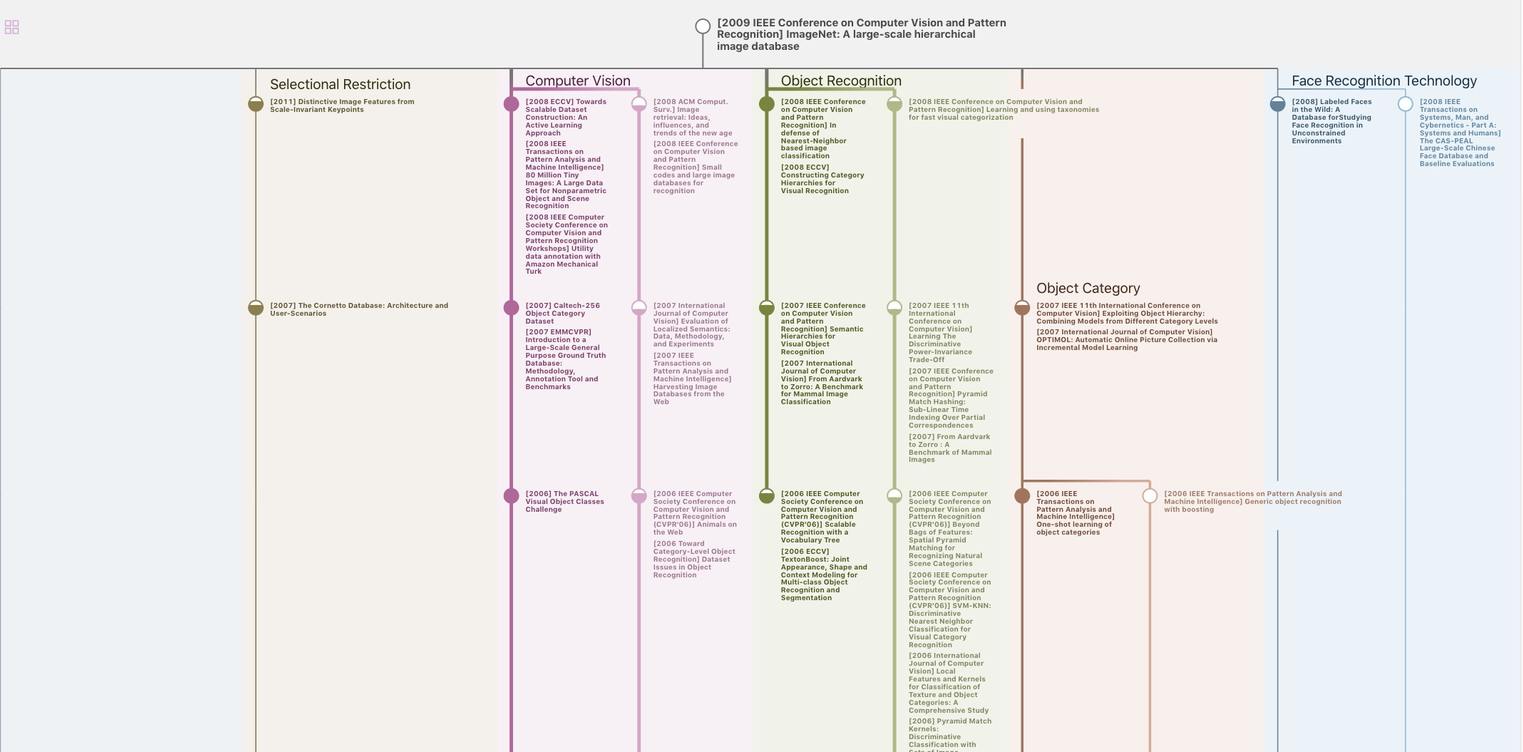
生成溯源树,研究论文发展脉络
Chat Paper
正在生成论文摘要