Learning to Rank in Person Re-Identification with Metric Ensembles
Computer Vision and Pattern Recognition(2015)
摘要
We propose an effective structured learning based approach to the problem of person re-identification which outperforms the current state-of-the-art on most benchmark data sets evaluated. Our framework is built on the basis of multiple low-level hand-crafted and high-level visual features. We then formulate two optimization algorithms, which directly optimize evaluation measures commonly used in person re-identification, also known as the Cumulative Matching Characteristic (CMC) curve. Our new approach is practical to many real-world surveillance applications as the re-identification performance can be concentrated in the range of most practical importance. The combination of these factors leads to a person re-identification system which outperforms most existing algorithms. More importantly, we advance state-of-the-art results on person re-identification by improving the rank-1 recognition rates from 40% to 50% on the iLIDS benchmark, 16% to 18% on the PRID2011 benchmark, 43% to 46% on the VIPeR benchmark, 34% to 53% on the CUHK01 benchmark and 21% to 62% on the CUHK03 benchmark.
更多查看译文
关键词
person reidentification,metric ensembles,structured learning,benchmark data sets,optimization algorithms,cumulative matching characteristic,CMC curve,PRID2011 benchmark,VIPeR benchmark,CUHK01 benchmark,CUHK03 benchmark
AI 理解论文
溯源树
样例
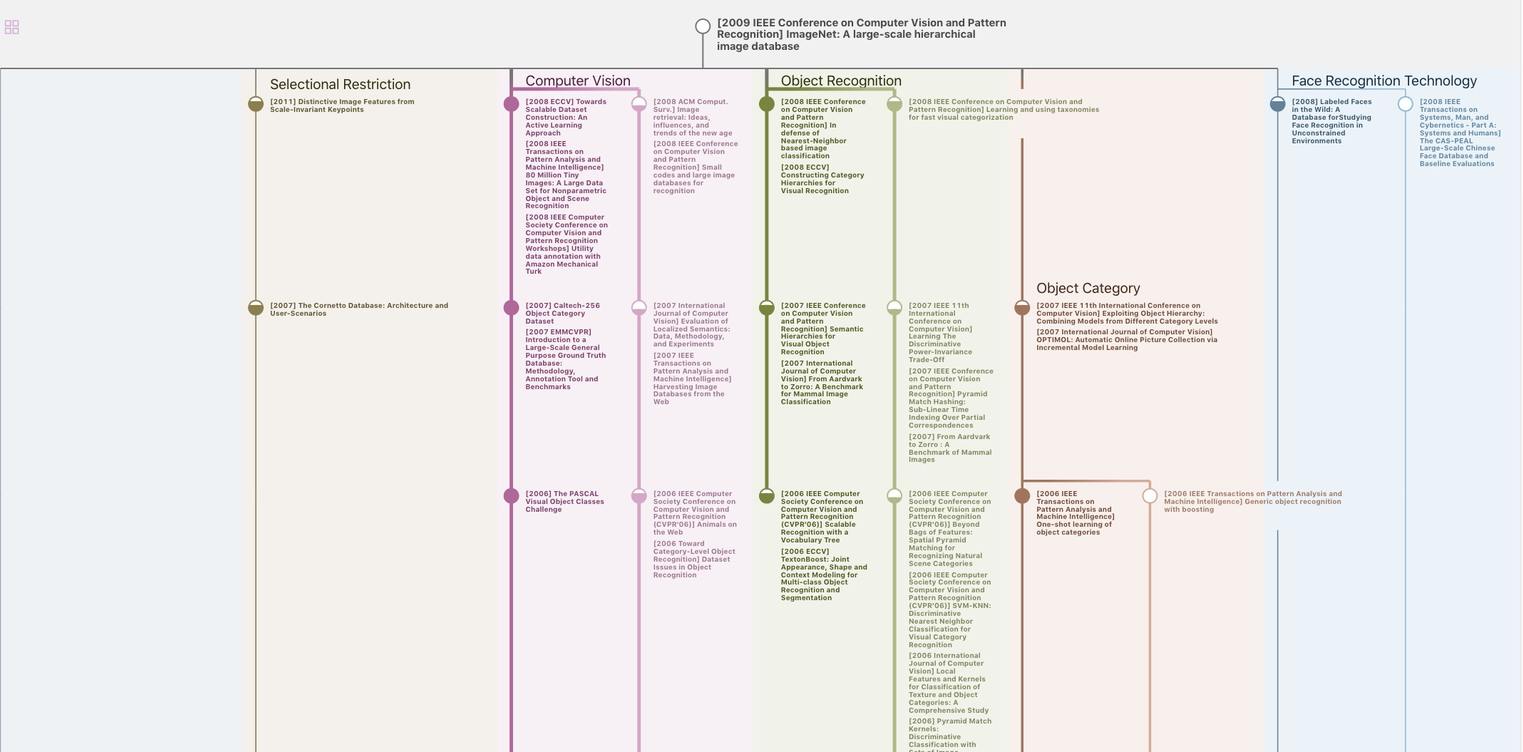
生成溯源树,研究论文发展脉络
Chat Paper
正在生成论文摘要