Single Image Super-Resolution From Transformed Self-Exemplars
2015 IEEE Conference on Computer Vision and Pattern Recognition (CVPR)(2015)
摘要
Self-similarity based super-resolution (SR) algorithms are able to produce visually pleasing results without extensive training on external databases. Such algorithms exploit the statistical prior that patches in a natural image tend to recur within and across scales of the same image. However, the internal dictionary obtained from the given image may not always be sufficiently expressive to cover the textural appearance variations in the scene. In this paper, we extend self-similarity based SR to overcome this drawback. We expand the internal patch search space by allowing geometric variations. We do so by explicitly localizing planes in the scene and using the detected perspective geometry to guide the patch search process. We also incorporate additional affine transformations to accommodate local shape variations. We propose a compositional model to simultaneously handle both types of transformations. We extensively evaluate the performance in both urban and natural scenes. Even without using any external training databases, we achieve significantly superior results on urban scenes, while maintaining comparable performance on natural scenes as other state-of-the-art SR algorithms.
更多查看译文
关键词
single image super-resolution,self-similarity based super-resolution algorithms,statistical prior,internal dictionary,self-similarity based SR algorithm,geometric variations,patch search process,local shape variations
AI 理解论文
溯源树
样例
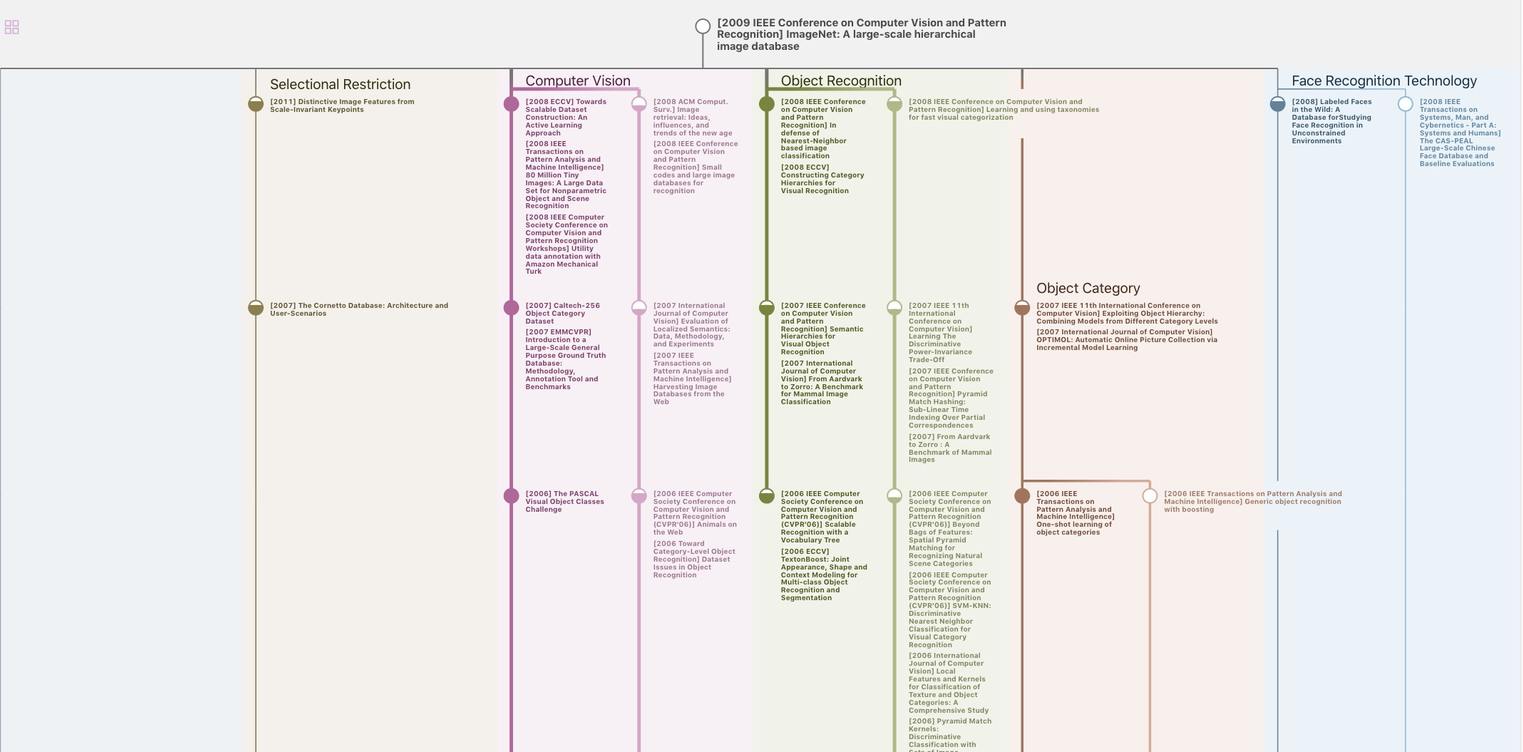
生成溯源树,研究论文发展脉络
Chat Paper
正在生成论文摘要