Revisiting Kernelized Locality-Sensitive Hashing for Improved Large-Scale Image Retrieval
2015 IEEE Conference on Computer Vision and Pattern Recognition (CVPR)(2014)
摘要
We present a simple but powerful reinterpretation of kernelized locality-sensitive hashing (KLSH), a general and popular method developed in the vision community for performing approximate nearest-neighbor searches in an arbitrary reproducing kernel Hilbert space (RKHS). Our new perspective is based on viewing the steps of the KLSH algorithm in an appropriately projected space, and has several key theoretical and practical benefits. First, it eliminates the problematic conceptual difficulties that are present in the existing motivation of KLSH. Second, it yields the first formal retrieval performance bounds for KLSH. Third, our analysis reveals two techniques for boosting the empirical performance of KLSH. We evaluate these extensions on several large-scale benchmark image retrieval data sets, and show that our analysis leads to improved recall performance of at least 12%, and sometimes much higher, over the standard KLSH method.
更多查看译文
关键词
kernelized locality-sensitive hashing,vision community,approximate nearest-neighbor searches,reproducing kernel Hilbert space,RKHS,KLSH algorithm,retrieval performance bounds,large-scale benchmark image retrieval data sets,recall performance
AI 理解论文
溯源树
样例
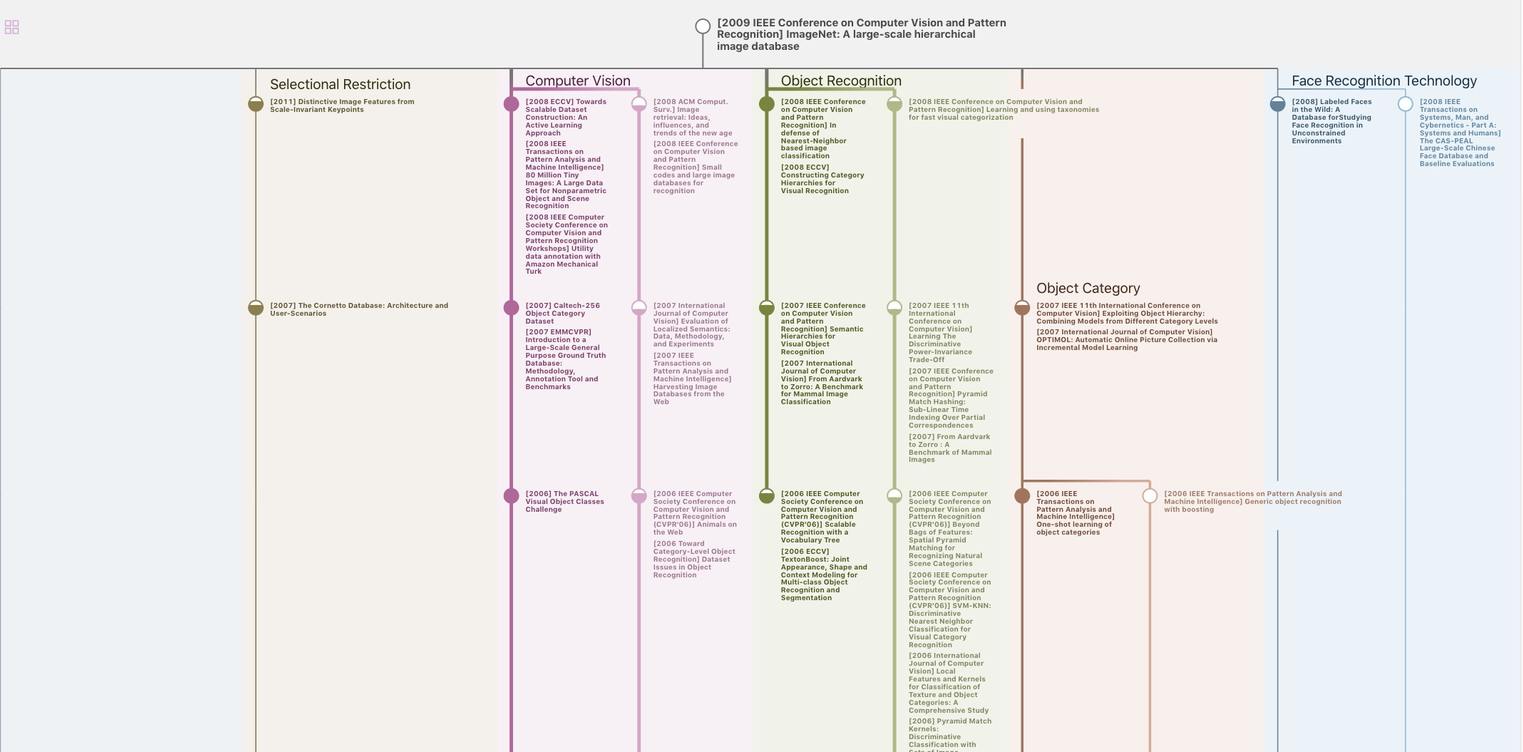
生成溯源树,研究论文发展脉络
Chat Paper
正在生成论文摘要