An efficient fashion-driven learning approach to model user preferences in on-line shopping scenarios
CVPR Workshops(2010)
摘要
In this work we tackle the problem of search personalization for on-line soft goods shopping. By learning what the user likes and what the user does not like, better search rankings and therefore a better overall shopping experience can be obtained. The first contribution of the work is in terms of feature selection: given the specific nature of the domain, we combine the traditional visual and text feature into a fashion-driven low dimensional space, compact yet very discriminative. On the learning stage, we describe a two step hybrid learning algorithm, that combines a discriminative model learned off-line over historical data, with an extremely efficient generative model, updated on-line according to the user behavior. Qualitative and quantitative analyses show promising results.
更多查看译文
关键词
Internet,behavioural sciences computing,data mining,image representation,learning (artificial intelligence),recommender systems,fashion-driven learning approach,feature selection,model user preferences,on-line shopping scenarios,search personalization,
AI 理解论文
溯源树
样例
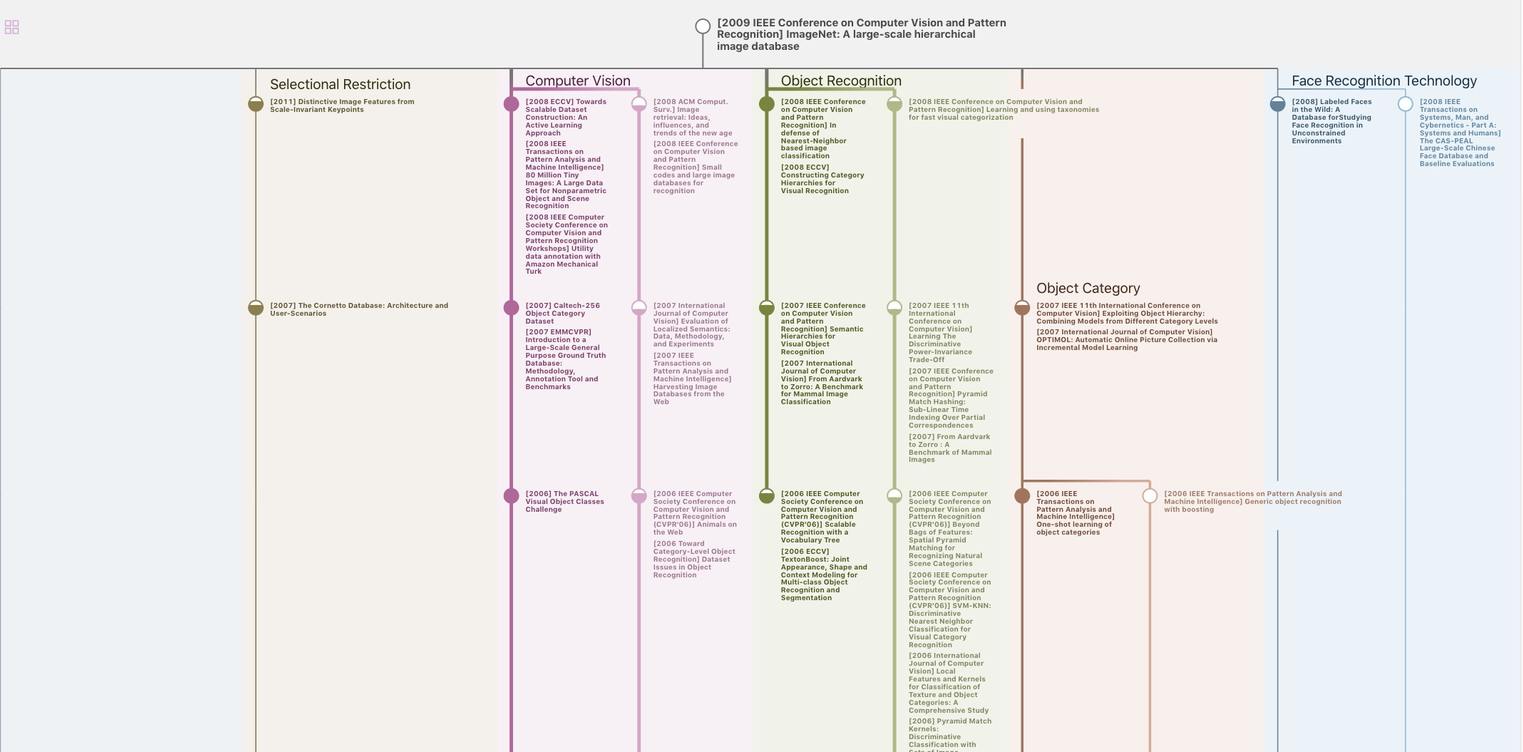
生成溯源树,研究论文发展脉络
Chat Paper
正在生成论文摘要