Robust Excitation-Based Features for Automatic Speech Recognition
ICASSP(2015)
摘要
In this paper we investigate the use of noise-robust features characterizing the speech excitation signal as complementary features to the usually considered vocal tract based features for Automatic Speech Recognition (ASR). The proposed Excitation-based Features (EBF) are tested in a state-of-the-art Deep Neural Network (DNN) based hybrid acoustic model for speech recognition. The suggested excitation features expand the set of periodicity features previously considered for ASR, expecting that these features help in a better discrimination of the broad phonetic classes (e.g., fricatives, nasal, vowels, etc.). Our experiments on the AMI meeting transcription system showed that the proposed EBF yield a relative word error rate reduction of about 5% when combined with conventional PLP features. Further experiments led on Aurora4 confirmed the robustness of the EBF to both additive and convolutive noises, with a relative improvement of 4.3% obtained by combinining them with mel filter banks.
更多查看译文
关键词
neural networks,automatic speech recognition,speech excitation signal
AI 理解论文
溯源树
样例
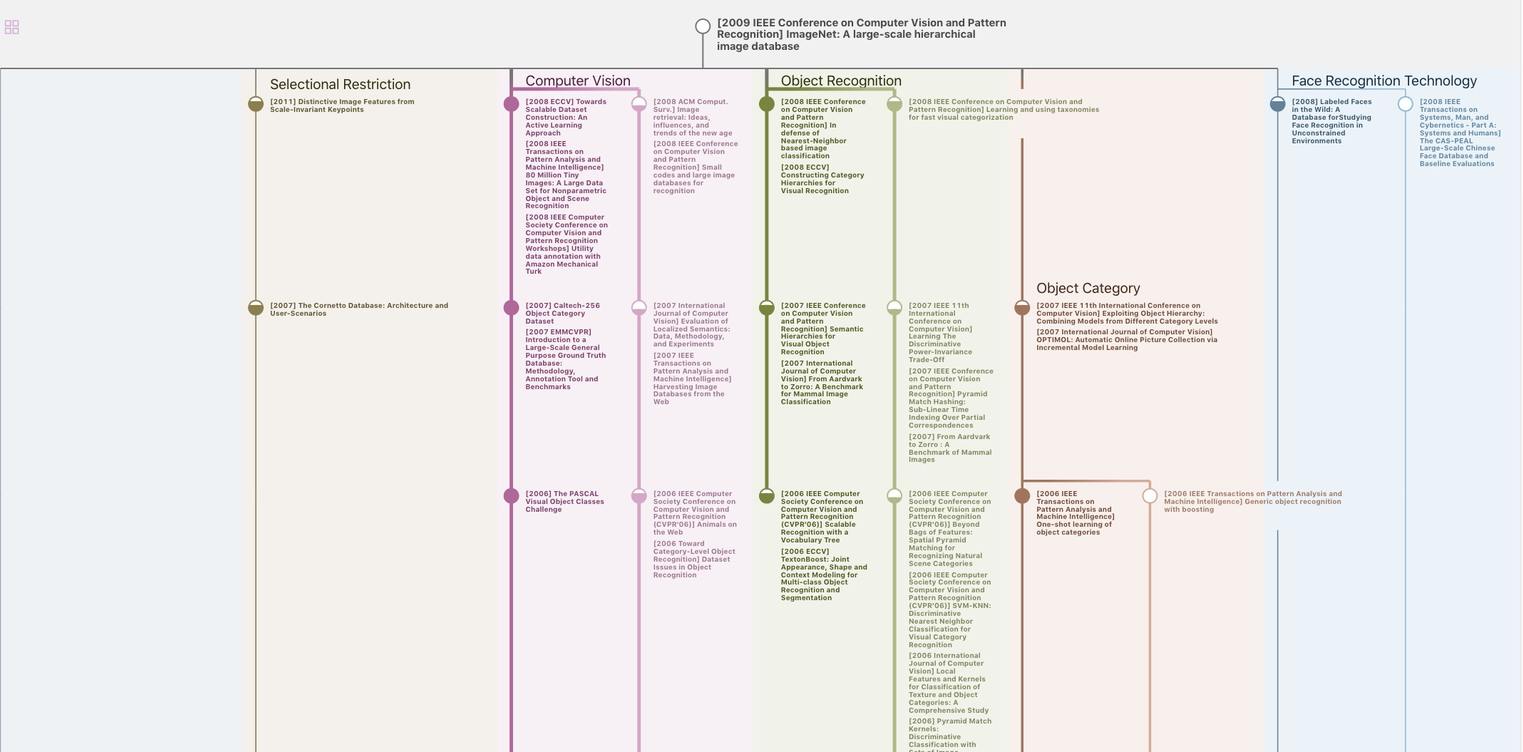
生成溯源树,研究论文发展脉络
Chat Paper
正在生成论文摘要