A learning-based approach to direction of arrival estimation in noisy and reverberant environments
2015 IEEE INTERNATIONAL CONFERENCE ON ACOUSTICS, SPEECH, AND SIGNAL PROCESSING (ICASSP)(2015)
摘要
This paper presents a learning-based approach to the task of direction of arrival estimation (DOA) from microphone array input. Traditional signal processing methods such as the classic least square (LS) method rely on strong assumptions on signal models and accurate estimations of time delay of arrival (TDOA) . They only work well in relatively clean conditions, but suffer from noise and reverberation distortions. In this paper, we propose a learning-based approach that can learn from a large amount of simulated noisy and reverberant microphone array inputs for robust DOA estimation. Specifically, we extract features from the generalised cross correlation (GCC) vectors and use a multilayer perceptron neural network to learn the nonlinear mapping from such features to the DOA. One advantage of the learning based method is that as more and more training data becomes available, the DOA estimation will become more and more accurate. Experimental results on simulated data show that the proposed learning based method produces much better results than the state-of-the-art LS method. The testing results on real data recorded in meeting rooms show improved root-mean-square error (RMSE) compared to the LS method.
更多查看译文
关键词
microphone arrays, direction of arrival, least squares, machine learning, neural networks
AI 理解论文
溯源树
样例
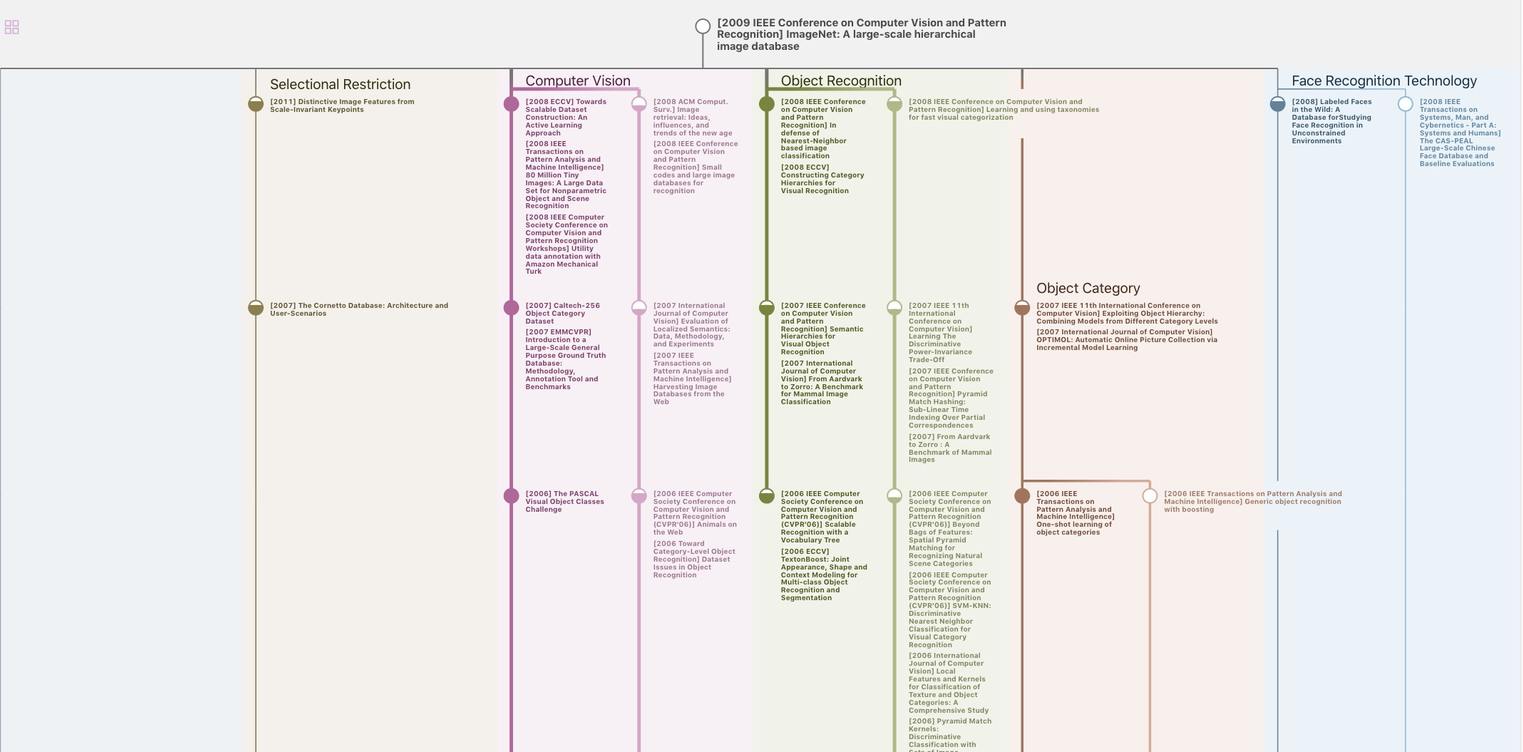
生成溯源树,研究论文发展脉络
Chat Paper
正在生成论文摘要