Spectral Validation Of Measurements In A Vehicle Tracking Dddas
INTERNATIONAL CONFERENCE ON COMPUTATIONAL SCIENCE, ICCS 2015 COMPUTATIONAL SCIENCE AT THE GATES OF NATURE(2015)
摘要
Vehicle tracking in adverse environments is a challenging problem because of the high number of factors constraining their motion and possibility of frequent occlusion. In such conditions, identification rates drop dramatically. Hyperspectral imaging is known to improve the robustness of target identification by recording extended data in many wavelengths. However, it is impossible to transmit such a high rate data in real time with a conventional full hyperspectral sensor. Thus, we present a persistent ground-based target tracking system, taking advantage of a state-of-the-art, adaptive, multi-modal sensor controlled by Dynamic Data Driven Applications Systems (DDDAS) methodology. This overcomes the data challenge of hyperspectral tracking by only using spectral data as required. Spectral features are inserted in a feature matching algorithm to identify spectrally likely matches and simplify multidimensional assignment algorithm. The sensor is tasked for spectra acquisition by the prior estimates from the Gaussian Sum Filter and foreground mask generated by the background subtraction. Prior information matching the target features is used to tackle false negatives in the background subtraction output. The proposed feature-aided tracking system is evaluated in a challenging scene with a realistic vehicular simulation.
更多查看译文
关键词
vehicle tracking, efficient outlier exclusion, spectral features, multi-modal sensor
AI 理解论文
溯源树
样例
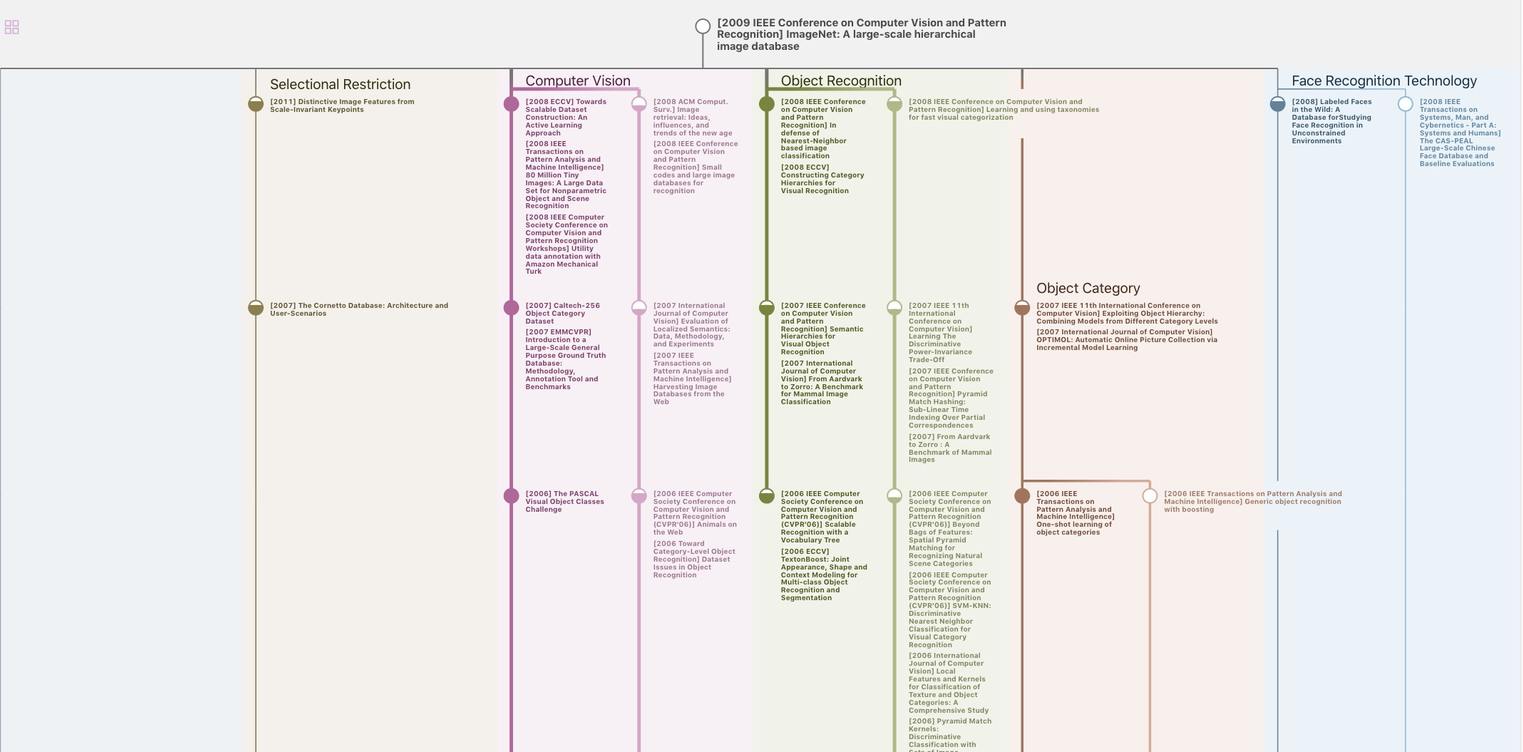
生成溯源树,研究论文发展脉络
Chat Paper
正在生成论文摘要