A mixture model clustering approach for temporal passenger pattern characterization in public transport
International Journal of Data Science and Analytics(2016)
摘要
Smartcard data provide a great number of information that are increasingly used nowadays. In the field of transport, they offer the opportunity to study passenger behavior, leading to a better knowledge of public transit demand and thereby granting the transport operators the ability to adapt their transport offer and services accordingly, both in space and in time. In particular, an accurate characterization of mobility patterns using data mining approaches has a very strong interest for transport planning purposes. This paper aims to propose a two-level generative mixture model that partitions passengers according to their temporal profiles. Using the timestamps of the passengers’ transactions in the public transport network, the first level models the passengers partitioning into a reduced set of clusters, whereas the second level captures how the trips made by each cluster of passengers are distributed over time. The proposed approach is applied on real ticketing data collected from the urban transport network of Rennes Métropole (France). The obtained results show that different passenger profiles can be discovered, thus highlighting several patterns of transport demand. The crossing of the clustering results with smartcard fare types as well as city characteristics such as academic centralities is also conducted in order to identify the close link between urban mobility and the socioeconomic characteristics of the city.
更多查看译文
关键词
Clustering,Smartcard data,Generative model,Public transport
AI 理解论文
溯源树
样例
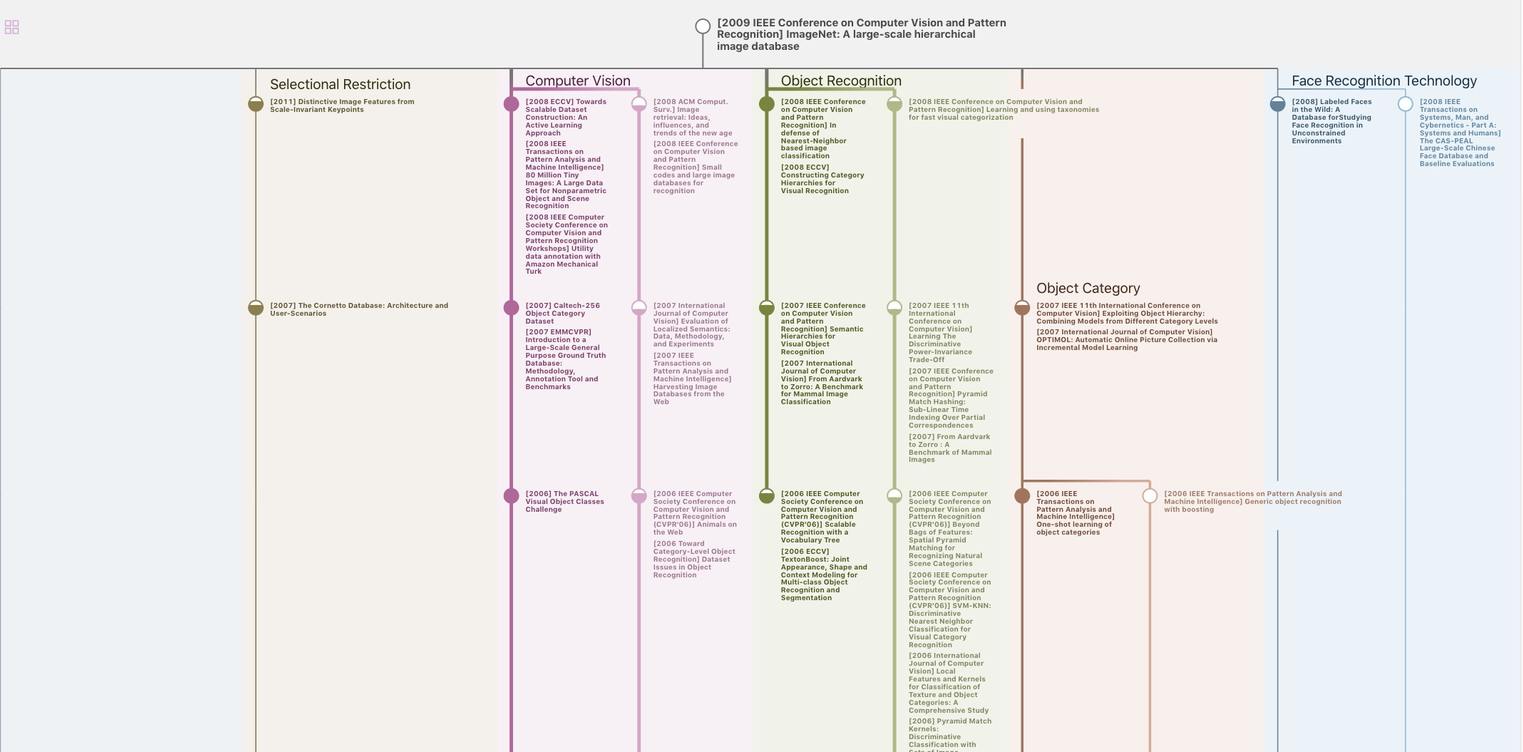
生成溯源树,研究论文发展脉络
Chat Paper
正在生成论文摘要