Modeling temporal dependencies in data using a DBN-LSTM
2015 IEEE International Conference on Data Science and Advanced Analytics (DSAA)(2015)
摘要
Since the advent of deep learning, it has been used to solve various problems using many different architectures. The application of such deep architectures to auditory data is also not uncommon. However, these architectures do not always adequately consider the temporal dependencies in data. We thus propose a new generic architecture called the Deep Belief Network - Long Short-Term Memory (DBN-LSTM) network that models sequences by keeping track of the temporal information while enabling deep representations in the data. We demonstrate this new architecture by applying it to the task of music generation and obtain state-of-the-art results.
更多查看译文
关键词
temporal dependencies modeling,deep learning,deep architectures,auditory data,generic architecture,deep belief network,long short-term memory,DBN-LSTM network,data deep representations,music generation
AI 理解论文
溯源树
样例
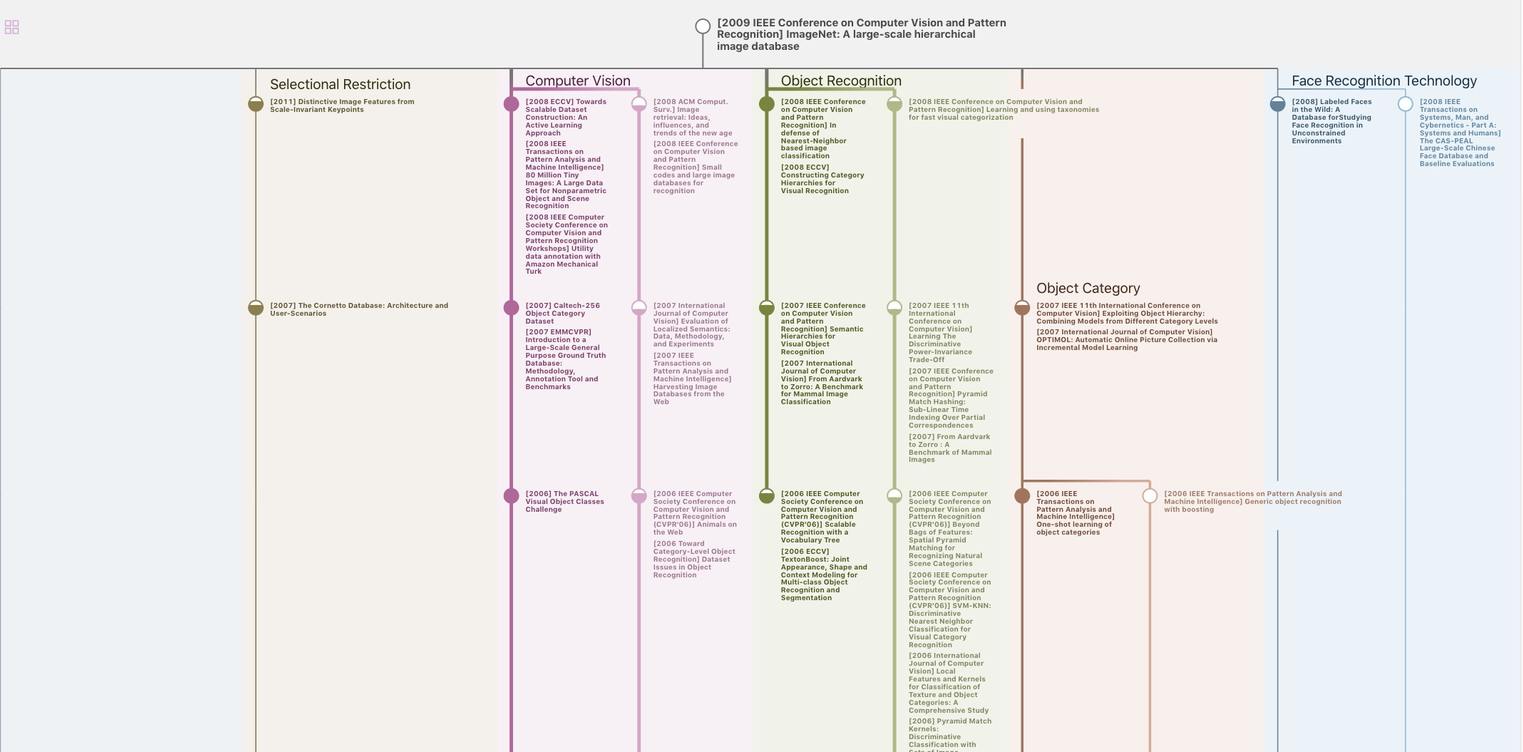
生成溯源树,研究论文发展脉络
Chat Paper
正在生成论文摘要