The Role of Machine Learning in Finding Chimeric RNAs
International Conference on Database and Expert Systems Applications(2015)
Abstract
High-throughput sequencing technology and bioinformatics have identified chimeric RNAs (chRNAs), raising the possibility of chRNAs expressing particularly in diseases can be used as potential biomarkers in both diagnosis and prognosis. The task of discriminating true chRNA from the false ones poses an interesting Machine Learning (ML) challenge. First of all, the sequencing data may contain false reads due to technical artefacts and during the analysis process, bioinformatics tools may generate false positives due to methodological biases. Thus predicting the real signal from the noise can be a hard task. Furthermore, even if we succeed to have a proper set of observations (enough sequencing data) about true chRNAs, chances are that the devised model can not be able to generalize beyond it. Like any other machine learning problem, the first big issue is finding the good data, observations, to build the prediction model. Unfortunately, as far as we were concerned, there is no common benchmark data available for chRNAs. And, the definition of a classification baseline is lacking in the related literature. In this work we are moving towards a benchmark data and a fair comparison analysis unraveling the role of ML techniques in finding chRNAs. We have developed a benchmark pipeline incorporating a mutated genome process and simulated RNA-seq data by Flux Simulator. These sequencing reads were aligned and annotated by CRAC. CRAC offers a new way to analyze the RNA-seq data by integrating genomic location and local coverage, allowing biological predictions in one step. The resulting data were used as a benchmark for our comparison analysis. We have observed that the no free lunch theorem do not hold for ensemble classifiers. Ensemble learning strategies demonstrated to be more robust to this classification problem, providing an average AUC performance of 95% (ACC=94%, Kappa=0.87%).
MoreTranslated text
Key words
Ensemble Learning,Classification,Chimeric RNAs,RNA-seq Data Analysis,High-throughput Sequencing
AI Read Science
Must-Reading Tree
Example
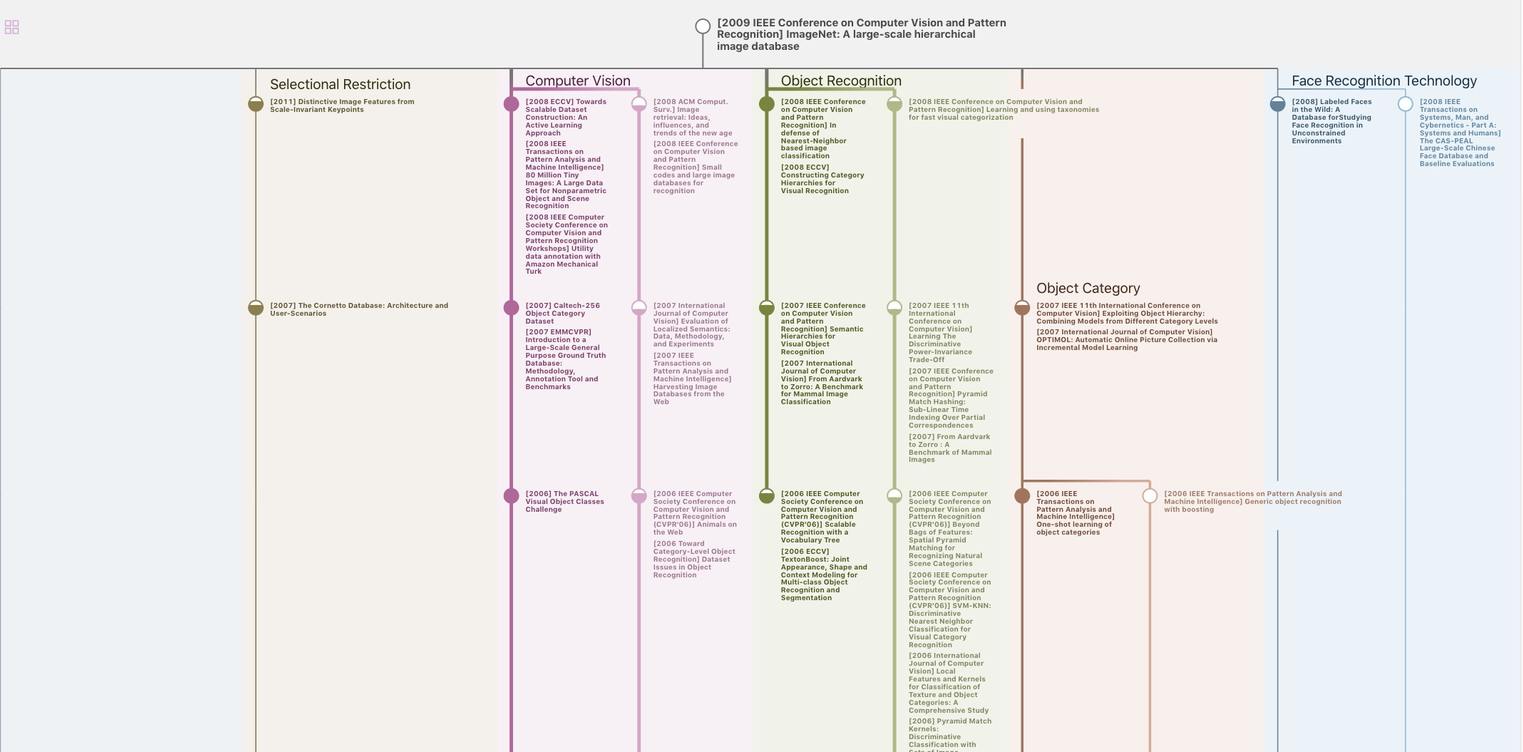
Generate MRT to find the research sequence of this paper
Chat Paper
Summary is being generated by the instructions you defined