Dimension Reduction Methods for Collaborative Mobile Gossip Learning
2016 24th Euromicro International Conference on Parallel, Distributed, and Network-Based Processing (PDP)(2016)
摘要
Decentralized learning algorithms are very sensitive to the size of the raw data records due to the resulting large communication cost. This can, in the worst case, even make decentralized learning infeasible. Dimension reduction is a key technique to compress data and to obtain small models. In this paper, we propose a number of robust and efficient decentralized approaches to dimension reduction in the system model where each network node holds only one data record. These algorithms build on searching for good random projections. We present a thorough experimental comparison of the proposed algorithms and compare them with a variant of distributed singular value decomposition (SVD), a state-of-the-art algorithm for dimension reduction. We base our experiments on a trace of real mobile phone usage. We conclude that our method based on selecting good random projections is preferable and provides good quality results when the output is required on a very short timescale, within tens of minutes. We also present a hybrid method that combines the advantages of random projections and SVD. We demonstrate that the hybrid method offers good performance over all timescales.
更多查看译文
关键词
distributed data mining,gossip,dimension reduction
AI 理解论文
溯源树
样例
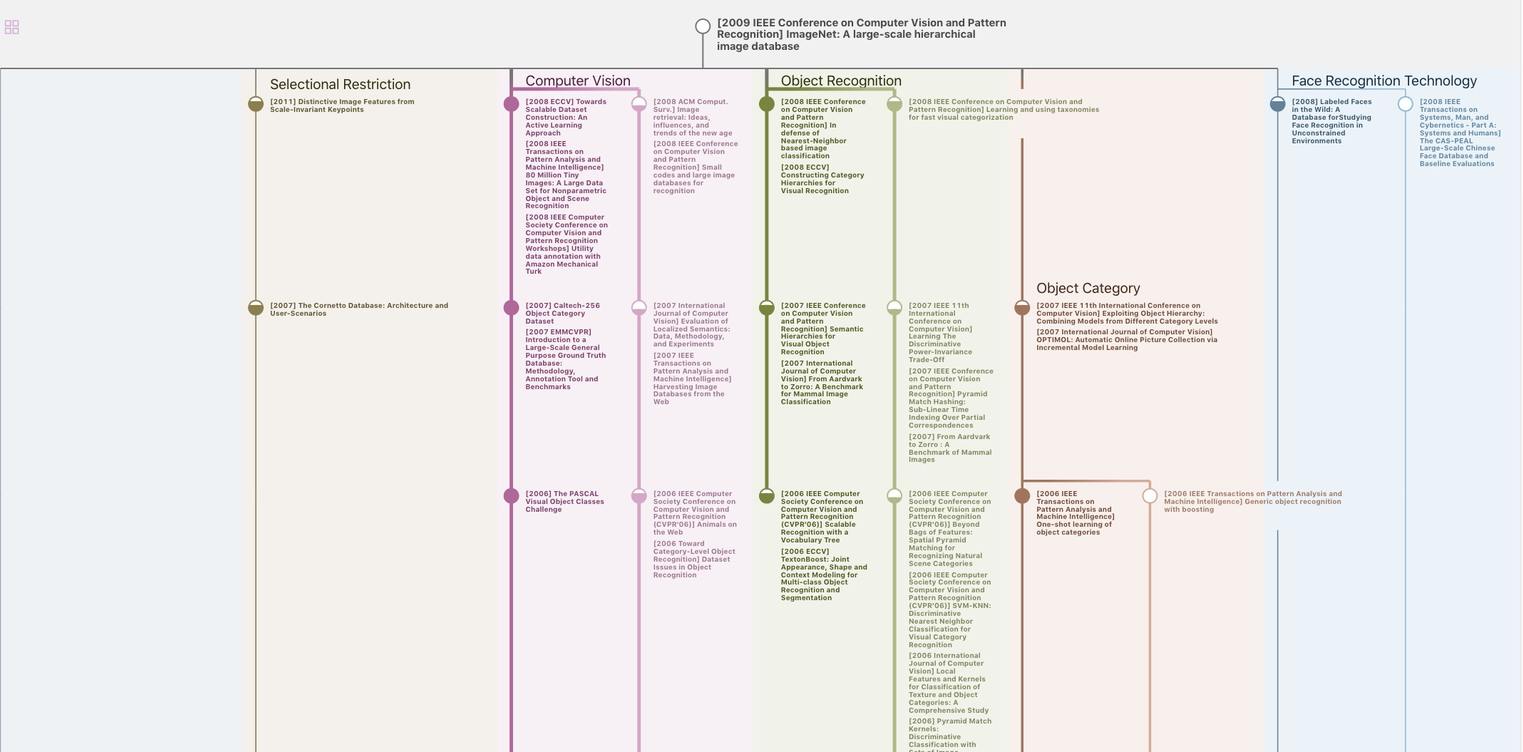
生成溯源树,研究论文发展脉络
Chat Paper
正在生成论文摘要