Short-Term Traffic Flow Forecasting: Multi-Metric Knn With Related Station Discovery
2015 12th International Conference on Fuzzy Systems and Knowledge Discovery (FSKD)(2015)
摘要
Nonparametric regression is a classic method for short-term traffic flow forecasting in Intelligent Transportation Systems (ITS). Feature space construction and distance metric selection are two important parts in nonparametric regression. Few of previous works have taken both these two aspects into account together. In addition, how to use information of related stations in network scale is a key to improve the performance of ITS. In this paper, we propose a novel three-stage framework based on KNN to handle the issues above for short-term traffic flow forecasting. In the first stage, the related origin stations and destination stations of target task are discovered from the whole traffic network. Then for each target task, a particular distance metric is learned in the second stage. Finally, an extended multi-metric k-nearest neighbor regression model is built in the third stage. Experimental results on real-world traffic dataset show that our multi-metric KNN model with Lasso outperforms the traditional KNN model and the feature construction method is effective.
更多查看译文
关键词
Metric Learning,Multi-Metric KNN,Traffic Flow Forecasting,Lasso
AI 理解论文
溯源树
样例
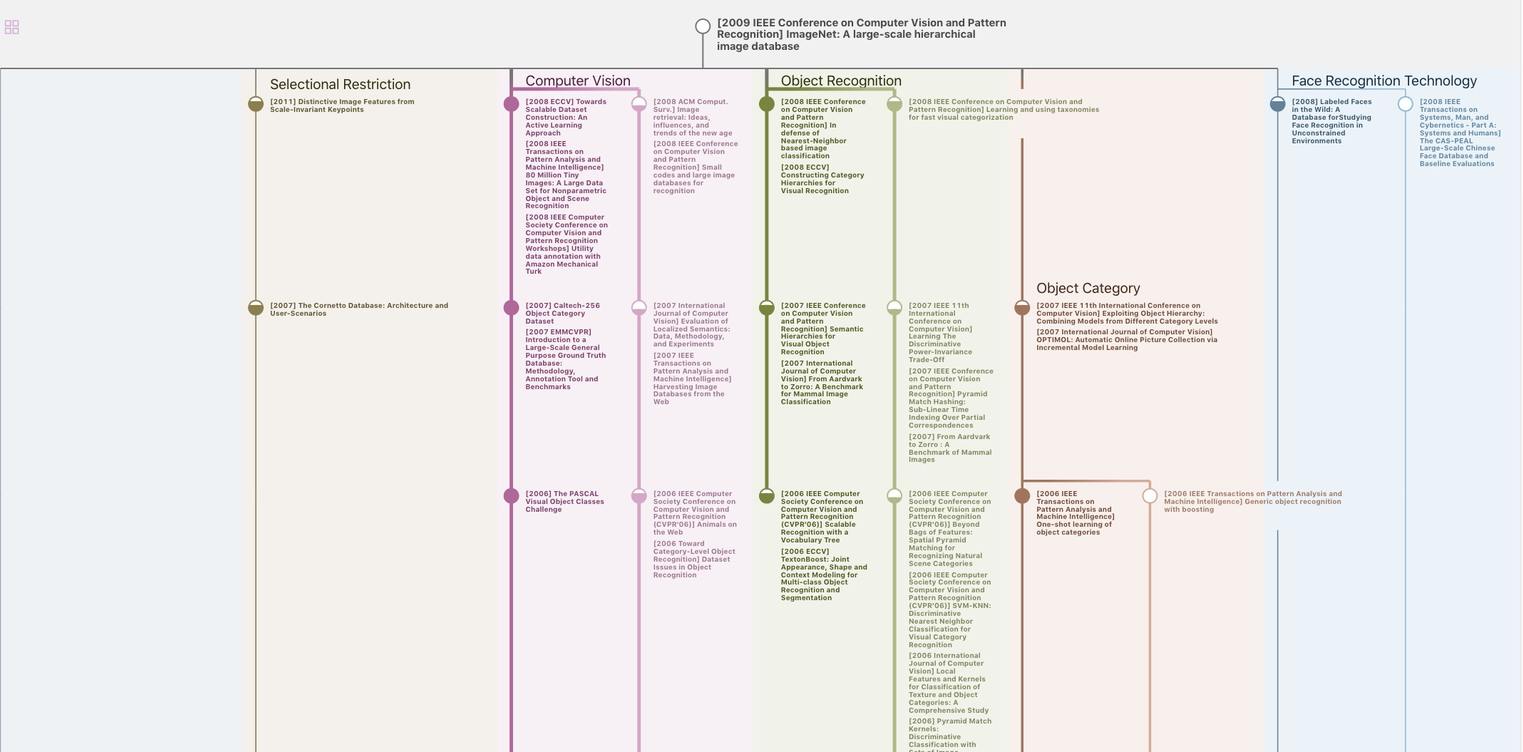
生成溯源树,研究论文发展脉络
Chat Paper
正在生成论文摘要