Finding the k in K-means Clustering: A Comparative Analysis Approach.
AI 2015: ADVANCES IN ARTIFICIAL INTELLIGENCE(2015)
摘要
This paper explores the application of inequality indices, a concept successfully applied in comparative software analysis among many application domains, to find the optimal value k for k-means when clustering road traffic data. We demonstrate that traditional methods for identifying the optimal value for k (such as gap statistic and Pham et al.'s method) are unable to produce meaningful values for k when applying them to a real-world dataset for road traffic. On the other hand, a method based on inequality indices shows significant promises in producing much more sensible values for the number k of clusters to be used in k-means clustering for the same road network traffic dataset.
更多查看译文
关键词
Traffic Volume, Traffic Data, Inequality Index, Theil Index, Traffic Dataset
AI 理解论文
溯源树
样例
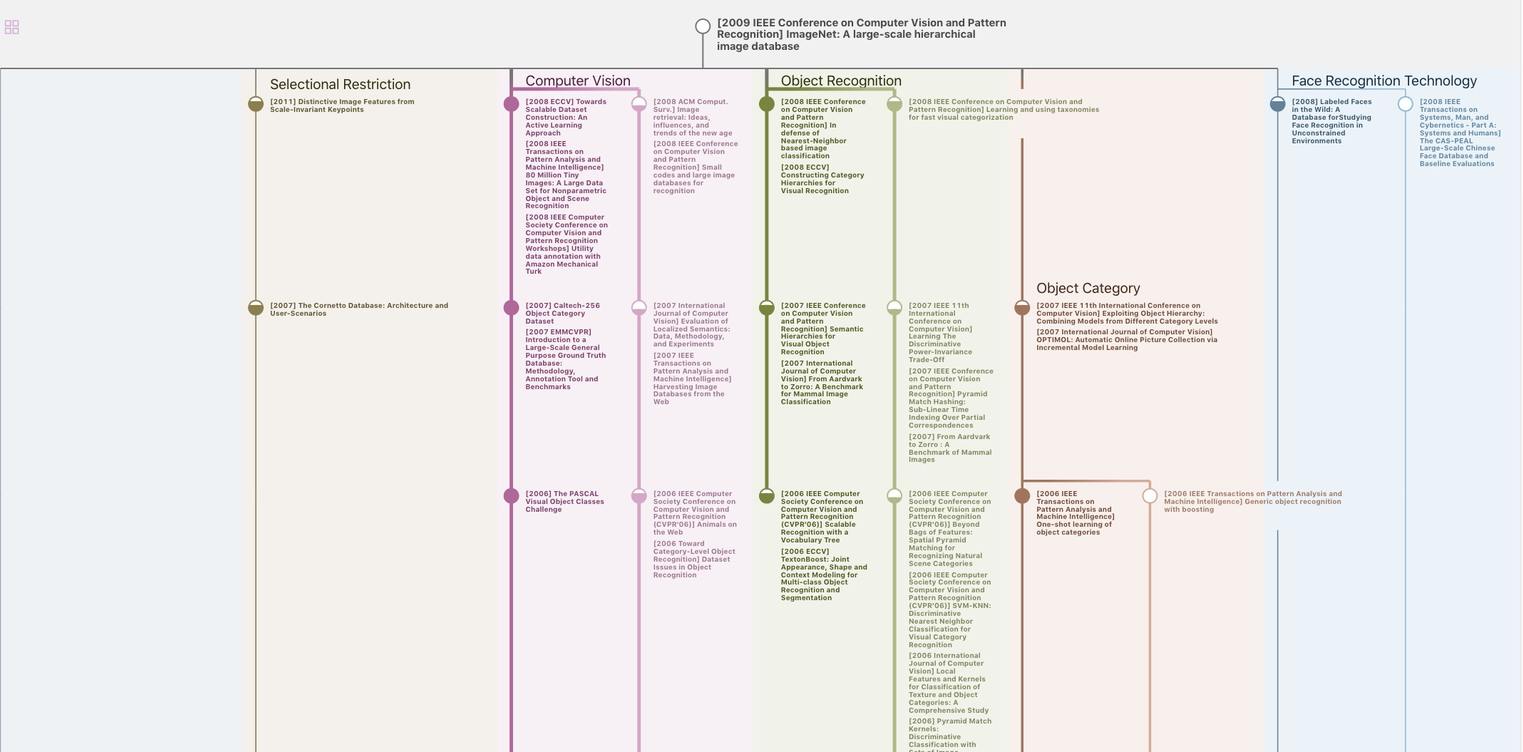
生成溯源树,研究论文发展脉络
Chat Paper
正在生成论文摘要