Probabilistic Belief Contraction: Considerations on Epistemic Entrenchment, Probability Mixtures and KL Divergence
AI 2015: ADVANCES IN ARTIFICIAL INTELLIGENCE(2015)
摘要
Probabilistic belief contraction is an operation that takes a probability distribution P representing a belief state along with an input sentence a representing some information to be removed from this belief state, and outputs a new probability distribution \(P^-_a\). The contracted belief state \(P^-_a\) can be represented as a mixture of two states: the original belief state P, and the resultant state \(P^*_{\lnot a}\) of revising P by \(\lnot a\). Crucial to this mixture is the mixing factor \(\epsilon \) which determines the proportion of P and \(P^*_{\lnot a}\) that are used in this process in a uniform manner. Ideas from information theory such as the principle of minimum cross-entropy have previously been used to motivate the choice of the probabilistic contraction operation. Central to this principle is the Kullback-Leibler (KL) divergence. In an earlier work we had shown that the KL divergence of \(P^-_a\) from P is fully determined by a function whose only argument is the mixing factor \(\epsilon \). In this paper we provide a way of interpreting \(\epsilon \) in terms of a belief ranking mechanism such as epistemic entrenchment that is in consonance with this result. We also provide a much needed justification for why the mixing factor \(\epsilon \) must be used in a uniform fashion by showing that the minimal divergence of \(P^-_{a}\) from P is achieved only when uniformity is respected.
更多查看译文
关键词
Belief Revision,Belief State,Belief Change,Revision Operator,Uniform Scaling
AI 理解论文
溯源树
样例
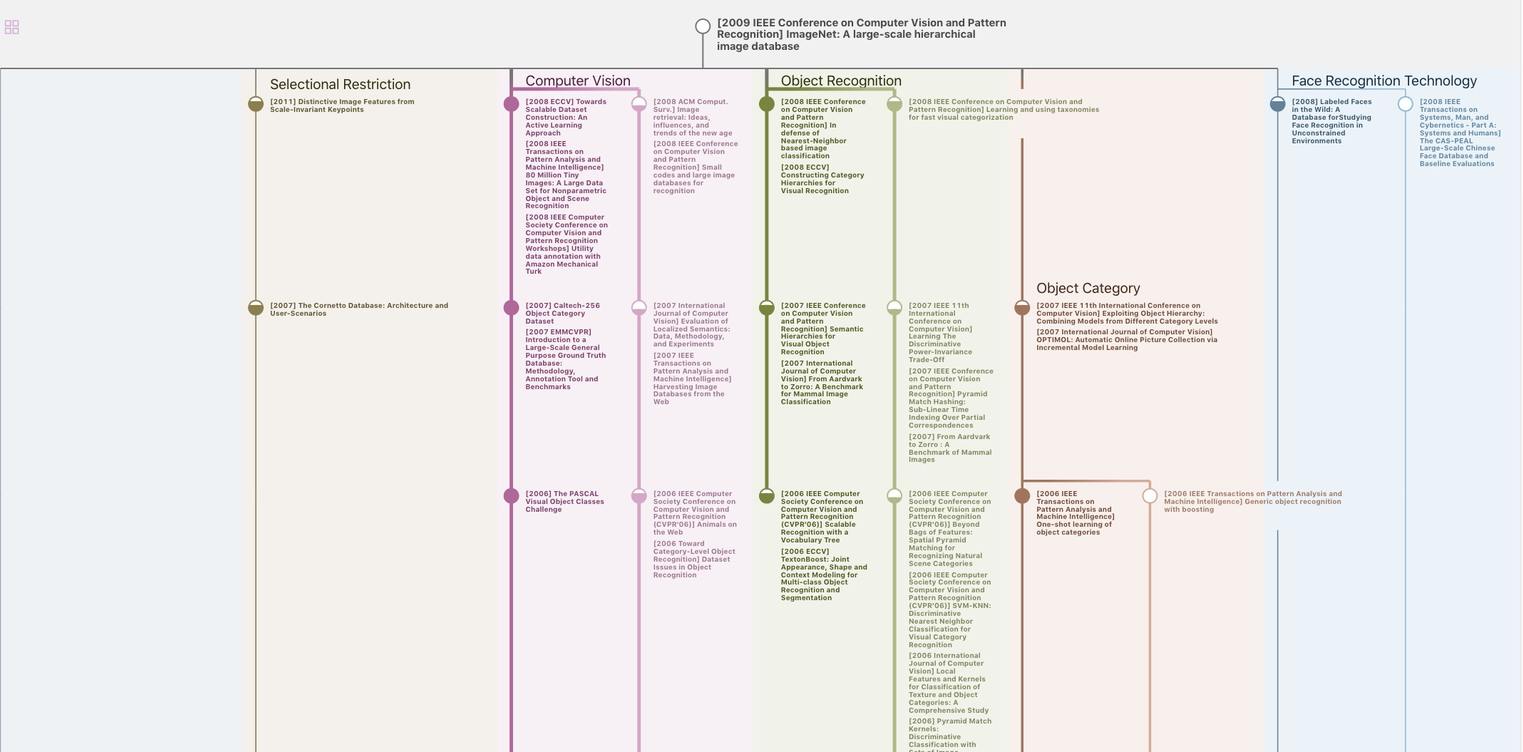
生成溯源树,研究论文发展脉络
Chat Paper
正在生成论文摘要