Improved Threshold Selection by Using Calibrated Probabilities for Random Forest Classifiers
CRV(2015)
摘要
Random Forest is a well-known ensemble learning method that achieves high recognition accuracies while preserving a fast training procedure. To construct a Random Forest classifier, several decision trees are arranged in a forest while a majority voting leads to the final decision. In order to split each node of a decision tree into two children, several possible variables are randomly selected while a splitting criterion is computed for each of them. Using this pool of possible splits, the Random Forest algorithm selects the best variable according to the splitting criterion. Often, this splitting is not reliable leading to a reduced recognition accuracy. In this paper, we propose to introduce an additional condition for selecting the best variable leading to an improvement of the recognition accuracy, especially for a smaller number of trees. We enhance the standard threshold selection by a quality estimation that is computed using a calibration method. The proposed method is evaluated on datasets for machine learning as well as object recognition.
更多查看译文
关键词
Random Forest,Machine Learning,Splitting,Object Recognition
AI 理解论文
溯源树
样例
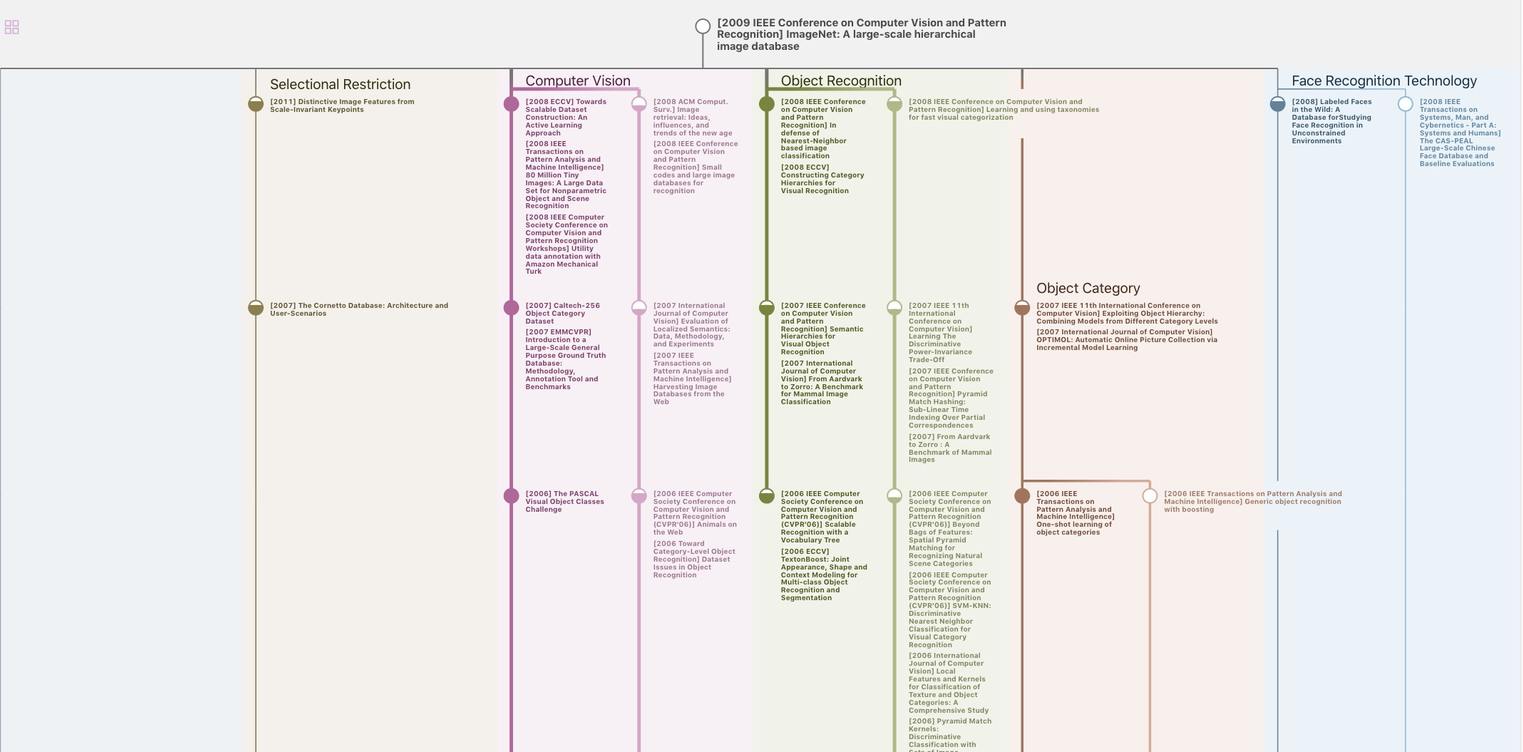
生成溯源树,研究论文发展脉络
Chat Paper
正在生成论文摘要